Comparative Study on Deep Learning Methods for Apple Ripeness Estimation on Tree
INTELLIGENT SYSTEMS DESIGN AND APPLICATIONS, ISDA 2021(2022)
摘要
In this paper, we propose an apple ripeness detection system based on deep learning methods. Three deep learning models, namely, Mask R-CNN, YOLOv5 and YOLOx, representing two-stage and one-stage object detectors, are employed to conduct apple fruit ripeness detection. Digital images related to three apple ripeness stages are collected from real scene to form dataset used for training and testing the three models. We conclude that YOLOv5, as the representative of one-stage algorithm, outperforms Mask R-CNN, as the representative of two-stage models in speed and mean average precision. In addition, YOLOv5 exceed YOLOx, an other one stage detector, in speed and mean average precision. Results showed the performance achieved by the ripeness detection system that provides up to 0.99 mean average precision.
更多查看译文
关键词
Machine learning, Object detection, Fruit ripeness, Deep learning, Neural Network, Classification, Localization
AI 理解论文
溯源树
样例
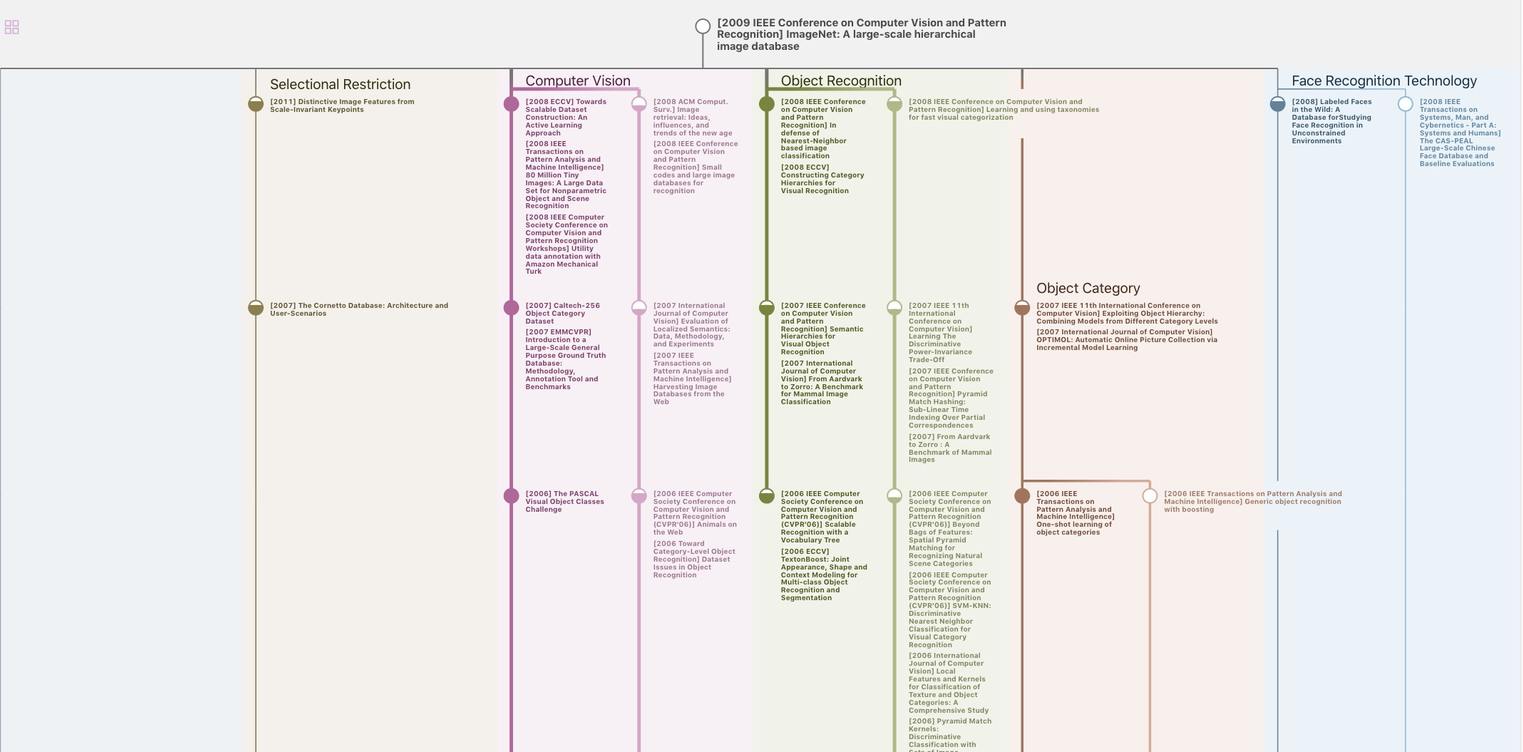
生成溯源树,研究论文发展脉络
Chat Paper
正在生成论文摘要