Multi-level context features extraction for named entity recognition
Computer Speech & Language(2023)
摘要
Bidirectional long short-term memory (Bi-LSTM), as one of the effective networks for sequence labeling tasks, is widely used in named entity recognition (NER). However, the sequential nature of Bi-LSTM and the inability to recognize multiple sentences at the same time make it impossible to obtain overall information. In this paper, to make up for the shortcomings of Bi-LSTM in extracting global information, we propose a hierarchical context model embedded with sentence level and document-level feature extraction. In sentence-level feature extraction, we use the self attention mechanism to extract sentence-level representations considering the different contribution of each word to the sentence. For document-level feature extraction, 3D convolutional neural network (CNN), which not only can extract features within sentences, but also pays attention to the sequential relationship between sentences, is used to extract document-level representations. Furthermore, we investigate a layer-by-layer residual (LBL Residual) structure to optimize each Bi-LSTM block of our model, which can solve the degradation problem that appears as the number of model layers increases. Experiments show that our model achieves results competitive with the state-of-the-art records on the CONLL-2003 and Ontonotes5.0 English datasets respectively.
更多查看译文
关键词
Bi-LSTM,Sentence-level feature,Document-level feature,Layer-by-layer Residual
AI 理解论文
溯源树
样例
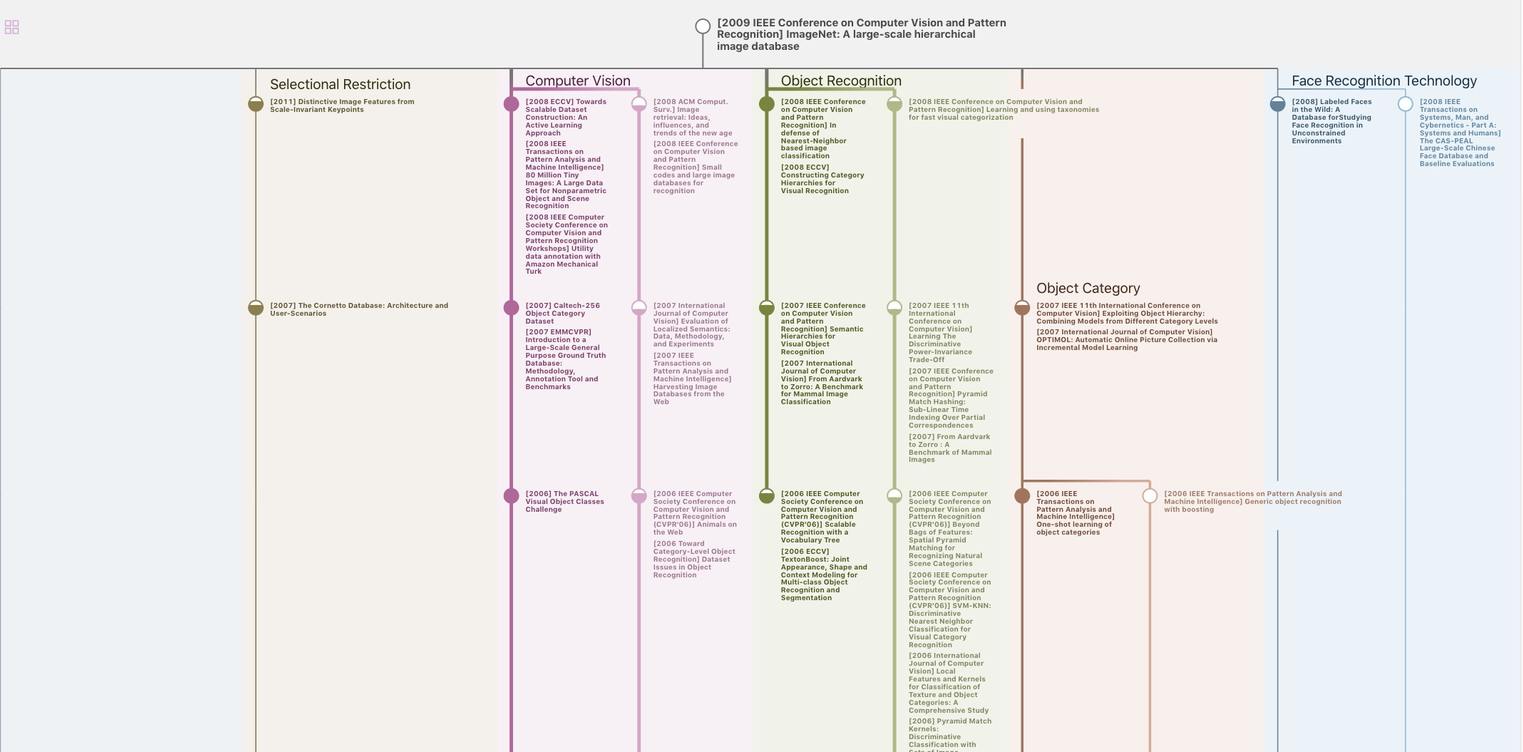
生成溯源树,研究论文发展脉络
Chat Paper
正在生成论文摘要