A High-Accuracy and Energy-Efficient CORDIC Based Izhikevich Neuron With Error Suppression and Compensation
IEEE Transactions on Biomedical Circuits and Systems(2022)
摘要
Bio-inspired neuron models are the key building blocks of brain-like neural networks for brain-science exploration and neuromorphic engineering applications. The efficient hardware design of bio-inspired neuron models is one of the challenges to implement brain-like neural networks, as the balancing of model accuracy, energy consumption and hardware cost is very challenging. This paper proposes a high-accuracy and energy-efficient Fast-Convergence COordinate Rotation DIgital Computer (FC-CORDIC) based Izhikevich neuron design. For ensuring the model accuracy, an error propagation model of the Izhikevich neuron is presented for systematic error analysis and effective error reduction. Parameter-Tuning Error Compensation (PTEC) method and Bitwidth-Extension Error Suppression (BEES) method are proposed to reduce the error of Izhikevich neuron design effectively. In addition, by utilizing the FC-CORDIC instead of conventional CORDIC for square calculation in the Izhikevich model, the redundant CORDIC iterations are removed and therefore, both the accumulated errors and required computation are effectively reduced, which significantly improve the accuracy and energy efficiency. An optimized fixed-point design of FC-CORDIC is also proposed to save hardware overhead while ensuring the accuracy. FPGA implementation results exhibit that the proposed Izhikevich neuron design can achieve high accuracy and energy efficiency with an acceptable hardware overhead, among the state-of-the-art designs.
更多查看译文
关键词
Izhikevich neuron model,biological neuron model,CORDIC,error analysis,fixed-point design optimization,brain-like neural network,spiking neural network,neuromorphic,FPGA
AI 理解论文
溯源树
样例
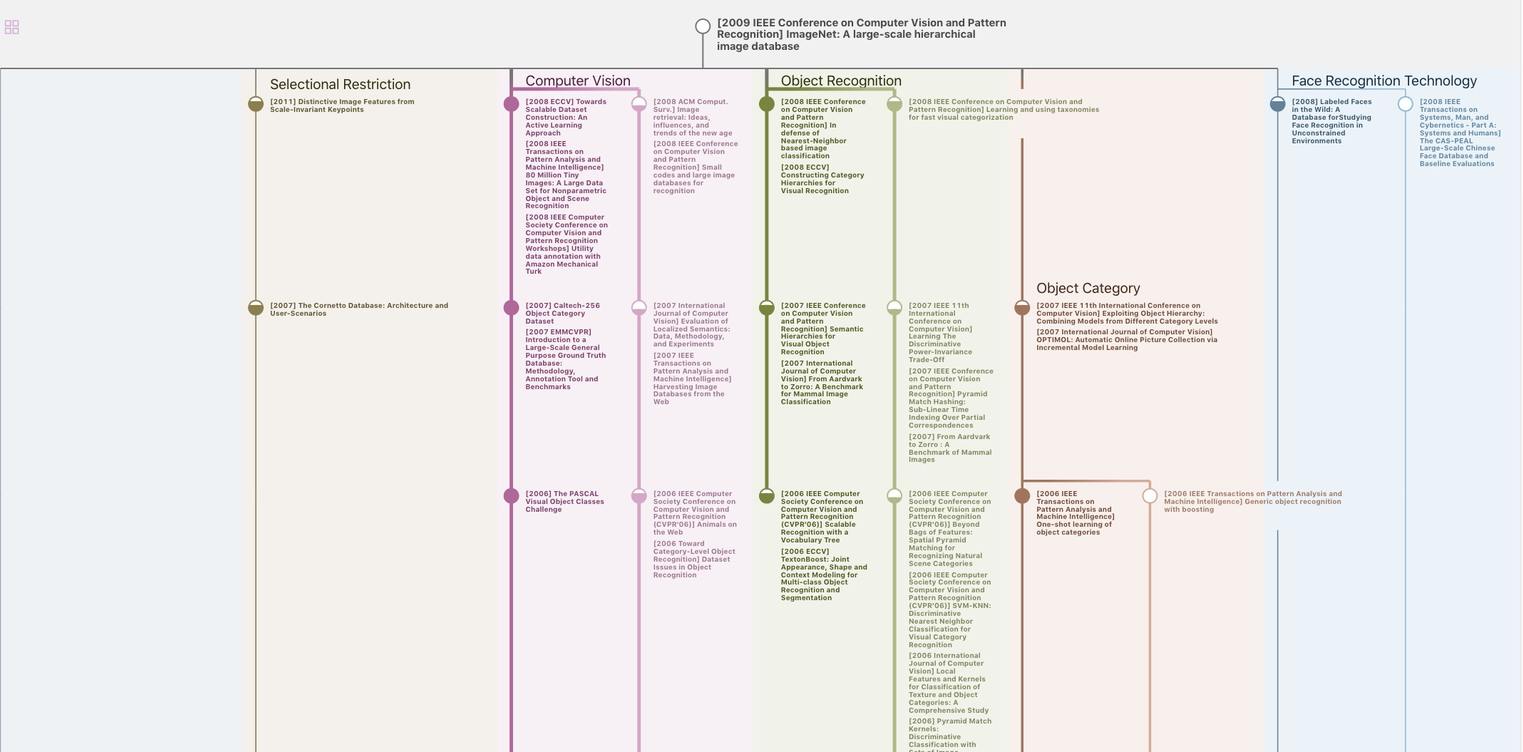
生成溯源树,研究论文发展脉络
Chat Paper
正在生成论文摘要