Multiscale unsupervised domain adaptation for automatic pancreas segmentation in CT volumes using adversarial learning
MEDICAL PHYSICS(2022)
摘要
Purpose Computer-aided automatic pancreas segmentation is essential for early diagnosis and treatment of pancreatic diseases. However, the annotation of pancreas images requires professional doctors and considerable expenditure. Due to imaging differences among various institution population, scanning devices, imaging protocols, and so on, significant degradation in the performance of model inference results is prone to occur when models trained with domain-specific (usually institution-specific) datasets are directly applied to new (other centers/institutions) domain data. In this paper, we propose a novel unsupervised domain adaptation method based on adversarial learning to address pancreas segmentation challenges with the lack of annotations and domain shift interference. Methods A 3D semantic segmentation model with attention module and residual module is designed as the backbone pancreas segmentation model. In both segmentation model and domain adaptation discriminator network, a multiscale progressively weighted structure is introduced to acquire different field of views. Features of labeled data and unlabeled data are fed in pairs into the proposed multiscale discriminator to learn domain-specific characteristics. Then the unlabeled data features with pseudodomain label are fed to the discriminator to acquire domain-ambiguous information. With this adversarial learning strategy, the performance of the segmentation network is enhanced to segment unseen unlabeled data. Results Experiments were conducted on two public annotated datasets as source datasets, respectively, and one private dataset as target dataset, where annotations were not used for the training process but only for evaluation. The 3D segmentation model achieves comparative performance with state-of-the-art pancreas segmentation methods on source domain. After implementing our domain adaptation architecture, the average dice similarity coefficient (DSC) of the segmentation model trained on the NIH-TCIA source dataset increases from 58.79% to 72.73% on the local hospital dataset, while the performance of the target domain segmentation model transferred from the medical segmentation decathlon (MSD) source dataset rises from 62.34% to 71.17%. Conclusions Correlations of features across data domains are utilized to train the pancreas segmentation model on unlabeled data domain, improving the generalization of the model. Our results demonstrate that the proposed method enables the segmentation model to make meaningful segmentation for unseen data of the training set. In the future, the proposed method has the potential to apply segmentation model trained on public dataset to clinical unannotated CT images from local hospital, effectively assisting radiologists in clinical practice.
更多查看译文
关键词
adversarial training, deep learning, pancreas segmentation, transfer learning, unsupervised domain adaptation
AI 理解论文
溯源树
样例
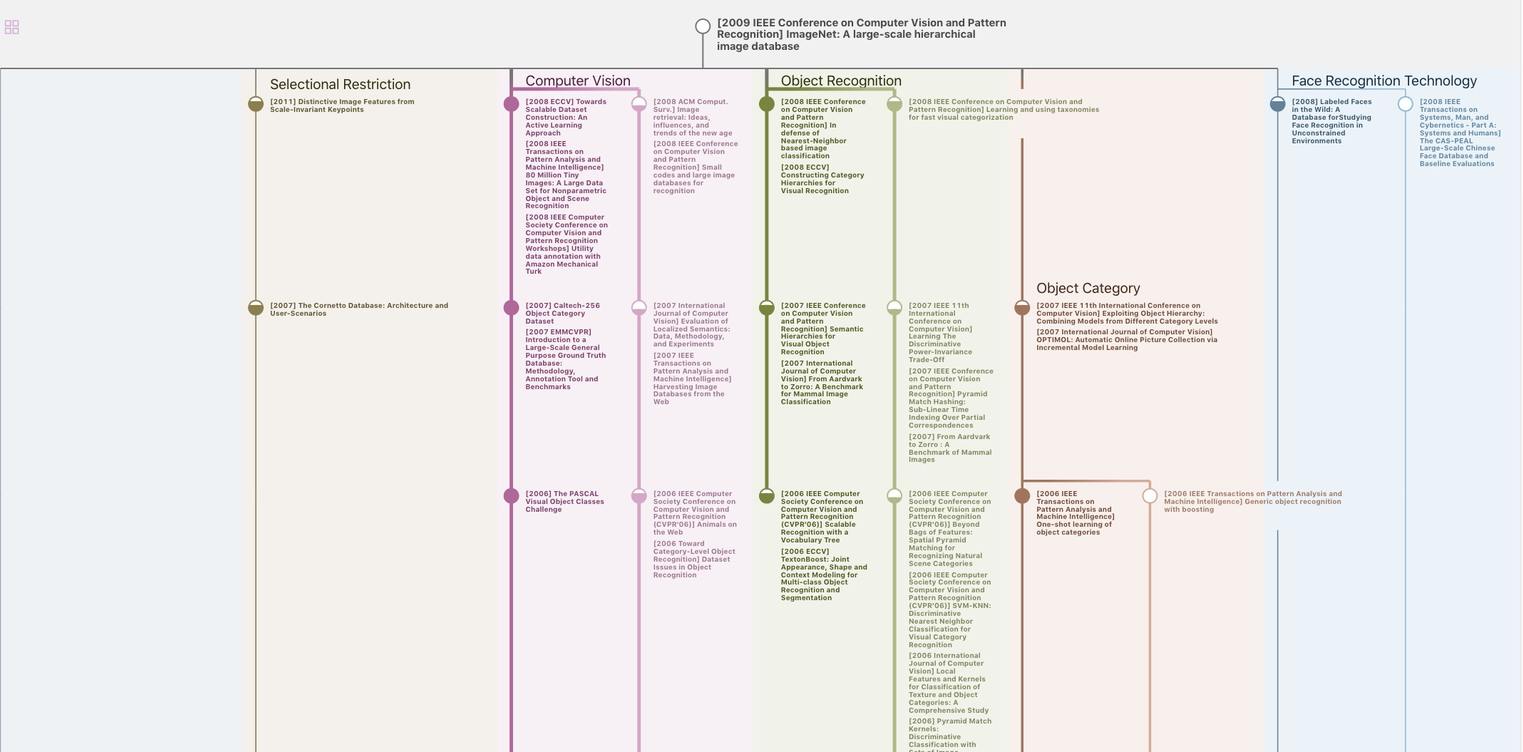
生成溯源树,研究论文发展脉络
Chat Paper
正在生成论文摘要