Spatiotemporal Propagation Learning for Network-Wide Flight Delay Prediction
IEEE TRANSACTIONS ON KNOWLEDGE AND DATA ENGINEERING(2024)
摘要
Accurate and interpretable delay predictions are vital for decision-making in the aviation industry. However, effectively incorporating spatiotemporal dependencies and external factors related to delay propagation remains a challenge. To address this challenge, we propose the SpatioTemporal Propagation Network (STPN), a novel space-time separable graph convolutional network that models delay propagation by considering both spatial and temporal factors. STPN uses a multi-graph convolution model that considers both geographic proximity and airline schedules from a spatial perspective, while employing a multi-head self-attention mechanism that can be learned end-to-end and explicitly accounts for various types of temporal dependencies in delay time series from a temporal perspective. Experiments on two real-world delay datasets show that STPN outperforms state-of-the-art methods for multi-step ahead arrival and departure delay prediction in large-scale airport networks. Additionally, the counterfactuals generated by STPN provide evidence of its ability to learn explainable delay propagation patterns. Comprehensive experiments also demonstrate that STPN sets a robust benchmark for general spatiotemporal forecasting. The code for STPN is available at https://github.com/Kaimaoge/STPN.
更多查看译文
关键词
Delay propagation,flight delay prediction,graph neural networks,predictive models
AI 理解论文
溯源树
样例
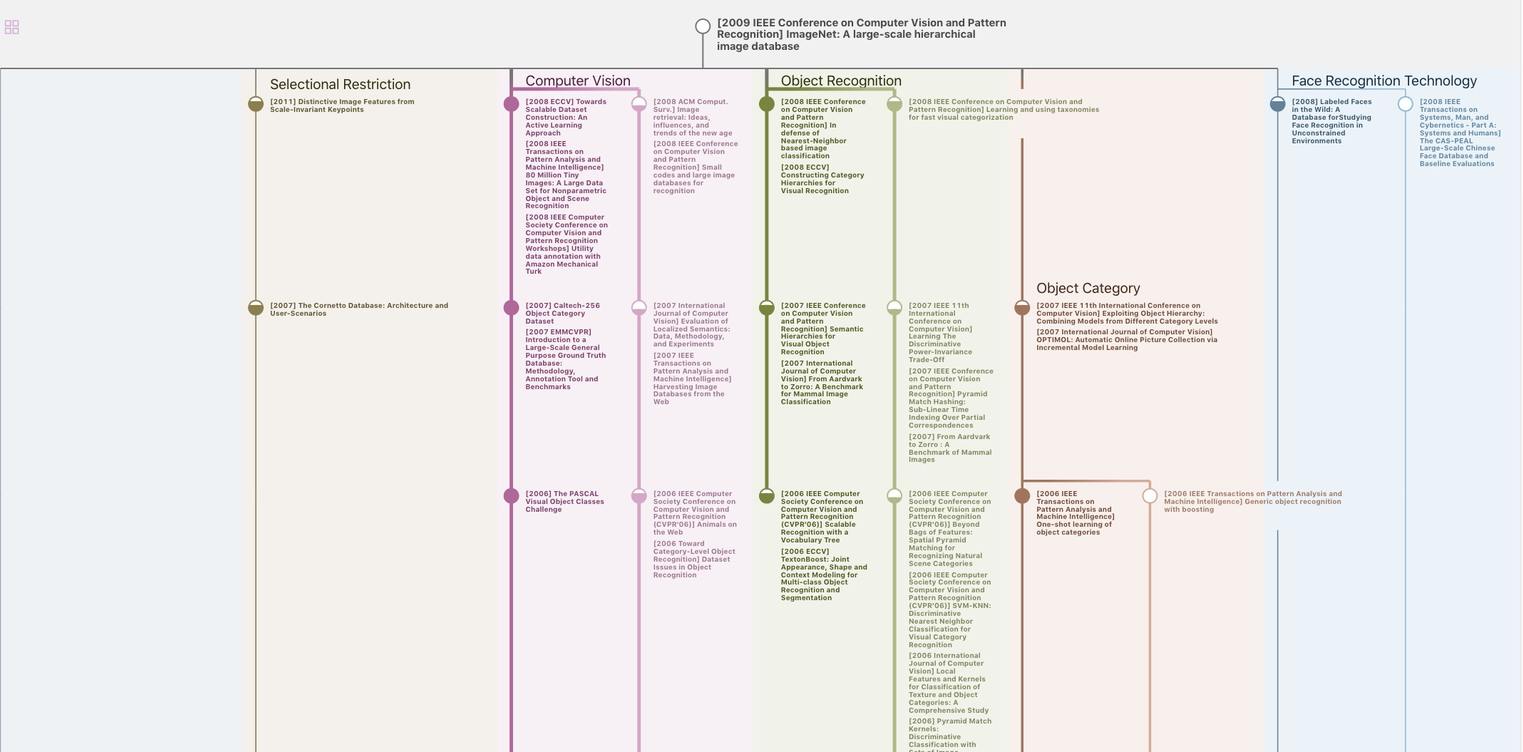
生成溯源树,研究论文发展脉络
Chat Paper
正在生成论文摘要