E2FIF: Push the Limit of Binarized Deep Imagery Super-Resolution Using End-to-End Full-Precision Information Flow.
arxiv(2023)
摘要
Binary neural network (BNN) provides a promising solution to deploy parameter-intensive deep single image super-resolution (SISR) models onto real devices with limited storage and computational resources. To achieve comparable performance with the full-precision counterpart, most existing BNNs for SISR mainly focus on compensating for the information loss incurred by binarizing weights and activations in the network through better approximations to the binarized convolution. In this study, we revisit the difference between BNNs and their full-precision counterparts and argue that the key to good generalization performance of BNNs lies on preserving a complete full-precision information flow along with an accurate gradient flow passing through each binarized convolution layer. Inspired by this, we propose to introduce a full-precision skip connection, or a variant thereof, over each binarized convolution layer across the entire network, which can increase the forward expressive capability and the accuracy of back-propagated gradient, thus enhancing the generalization performance. More importantly, such a scheme can be applied to any existing BNN backbones for SISR without introducing any additional computation cost. To validate the efficacy of the proposed approach, we evaluate it using four different backbones for SISR on four benchmark datasets and report obviously superior performance over existing BNNs and even some 4-bit competitors.
更多查看译文
关键词
Binary neural network, image super-resolution
AI 理解论文
溯源树
样例
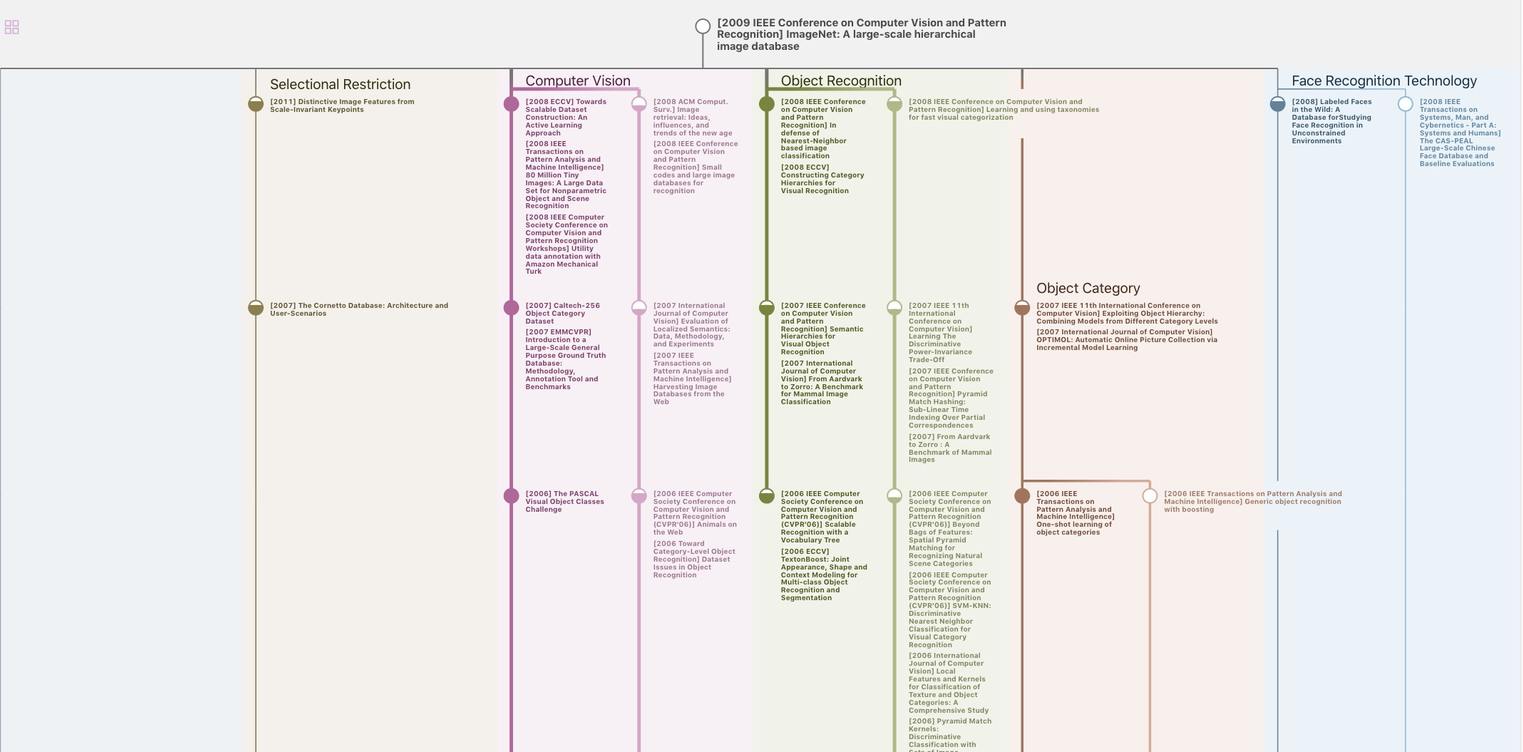
生成溯源树,研究论文发展脉络
Chat Paper
正在生成论文摘要