Subgraph Frequency Distribution Estimation using Graph Neural Networks
arxiv(2022)
摘要
Small subgraphs (graphlets) are important features to describe fundamental units of a large network. The calculation of the subgraph frequency distributions has a wide application in multiple domains including biology and engineering. Unfortunately due to the inherent complexity of this task, most of the existing methods are computationally intensive and inefficient. In this work, we propose GNNS, a novel representational learning framework that utilizes graph neural networks to sample subgraphs efficiently for estimating their frequency distribution. Our framework includes an inference model and a generative model that learns hierarchical embeddings of nodes, subgraphs, and graph types. With the learned model and embeddings, subgraphs are sampled in a highly scalable and parallel way and the frequency distribution estimation is then performed based on these sampled subgraphs. Eventually, our methods achieve comparable accuracy and a significant speedup by three orders of magnitude compared to existing methods.
更多查看译文
关键词
subgraph frequency distribution estimation,subgraph neural networks
AI 理解论文
溯源树
样例
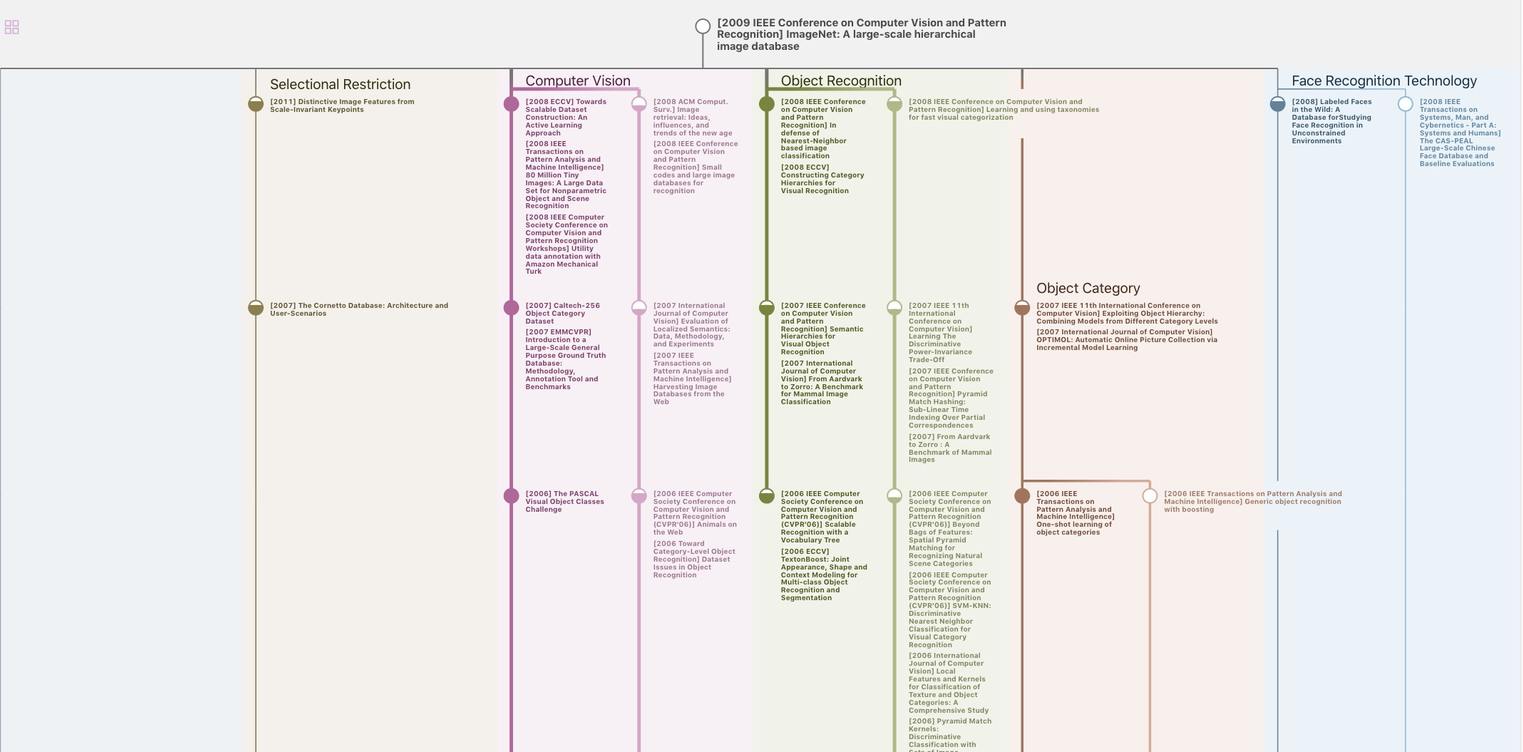
生成溯源树,研究论文发展脉络
Chat Paper
正在生成论文摘要