Rethinking Super-Resolution as Text-Guided Details Generation
International Multimedia Conference(2022)
摘要
ABSTRACTDeep neural networks have greatly promoted the performance of single image super-resolution (SISR). Conventional methods still resort to restoring the single high-resolution (HR) solution only based on the input of image modality. However, the image-level information is insufficient to predict adequate details and photo-realistic visual quality facing large upscaling factors (×8, ×16). In this paper, we propose a new perspective that regards the SISR as a semantic image detail enhancement problem to generate semantically reasonable HR image that are faithful to the ground truth. To enhance the semantic accuracy and the visual quality of the reconstructed image, we explore the multi-modal fusion learning in SISR by proposing a Text-Guided Super-Resolution (TGSR) framework, which can effectively utilize the information from the text and image modalities. Different from existing methods, the proposed TGSR could generate HR image details that match the text descriptions through a coarse-to-fine process. Extensive experiments and ablation studies demonstrate the effect of the TGSR, which exploits the text reference to recover realistic images.
更多查看译文
关键词
details,generation,super-resolution,text-guided
AI 理解论文
溯源树
样例
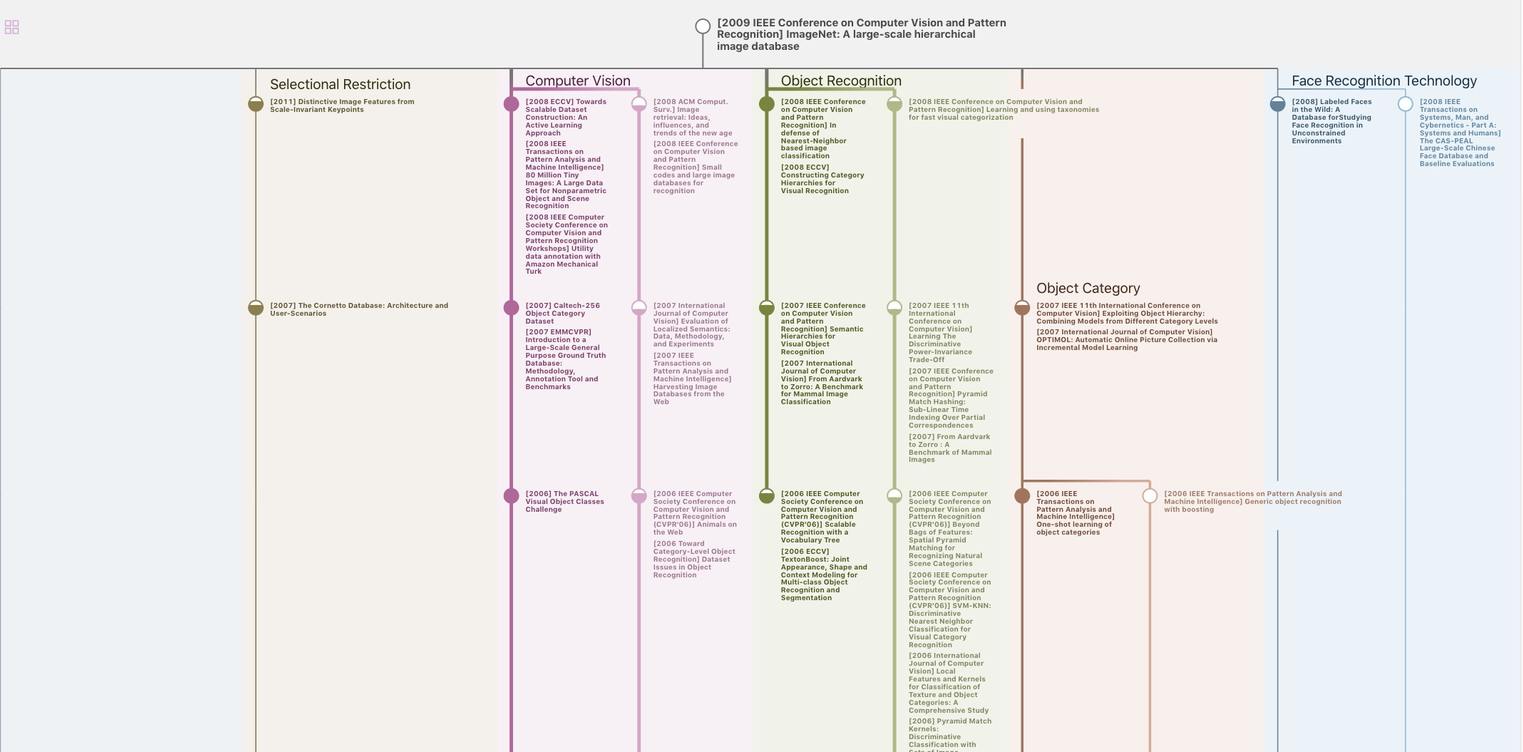
生成溯源树,研究论文发展脉络
Chat Paper
正在生成论文摘要