Computational portraits of the tumoral microenvironment in human breast cancer
Virchows Archiv(2022)
摘要
Breast cancer is the most diagnosed cancer in humans. In recent years, myxoid and proportionated stroma have been described as clinically significant in many cancer subtypes. Here computational portraits of tumor-associated stromata were created from a machine learning (ML) classifier using QuPath to evaluate proportionated stromal area (PSA), myxoid stromal ratio (MSR), and immune stroma proportion (ISP) from whole slide images (WSI). The ML classifier was validated in independent training ( n = 40) and validation ( n = 109) cohorts finding MSR, PSA, and ISP to be associated with tumor stage, lymph node status, Nottingham grade, stromal differentiation (SD), tumor size, estrogen receptor (ER), progesterone receptor (PR), and receptor tyrosine-protein kinase erbB-2 (HER-2). Overall, MSR correlated better with the clinicopathologic profile than PSA and ISP. High MSR was found to be associated with high tumor stage, low ISP, and high Nottingham histologic score. As a computational biomarker, high MSR was more likely to be associated with luminal B like, Her-2 enriched, and triple-negative biomarker status when compared to luminal A like. The supervised ML superpixel approach demonstrated here can be performed by a trained pathologist to provide a faster and more uniformed approach to the analysis to the tumoral microenvironment (TME). The TME may be relevant for clinical decision-making, determining chemotherapeutic efficacy, and guiding a more overall precision-based breast cancer care.
更多查看译文
关键词
Whole slide imaging, Computation, Portraits, Tumoral microenvironment, Human breast cancer
AI 理解论文
溯源树
样例
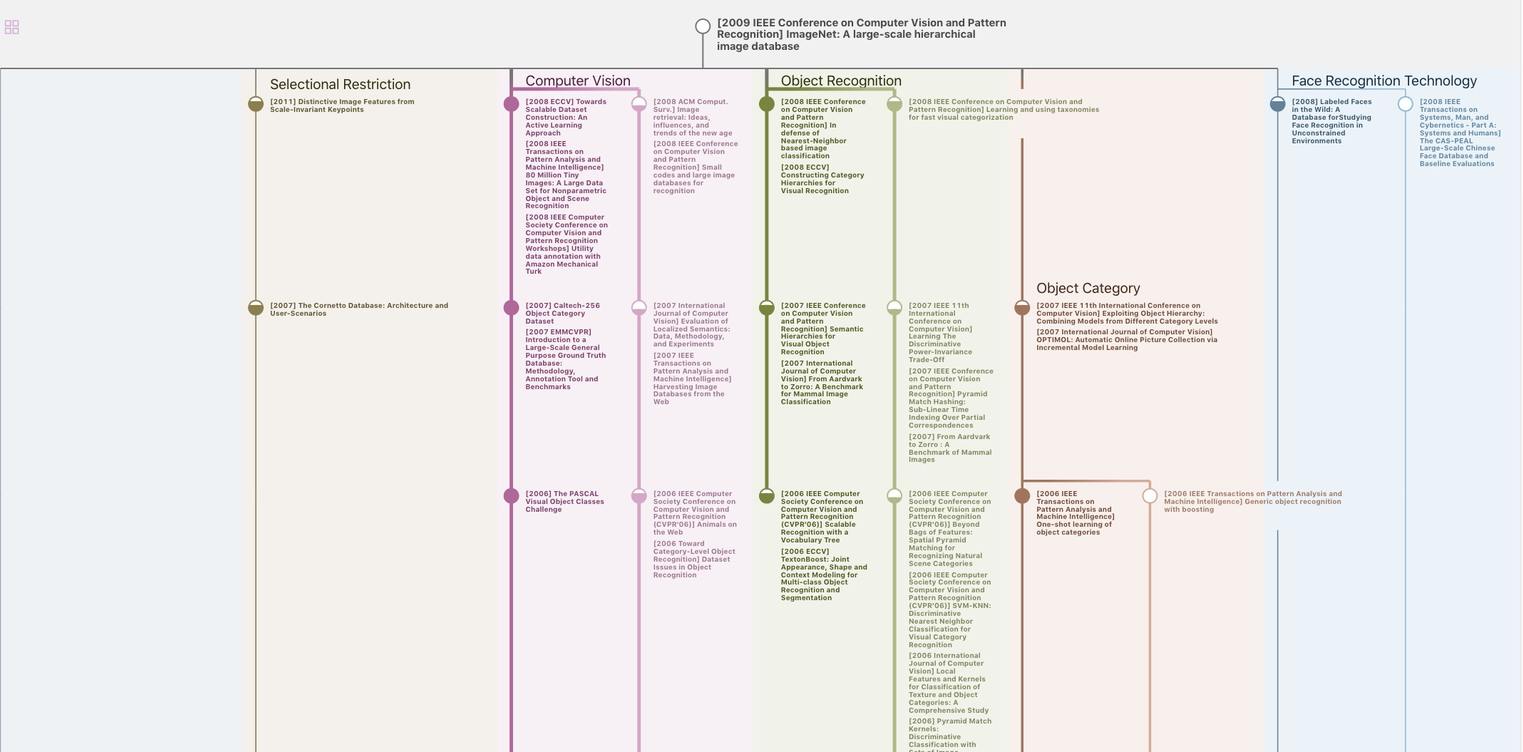
生成溯源树,研究论文发展脉络
Chat Paper
正在生成论文摘要