Machine-designed biotherapeutics: opportunities, feasibility and advantages of deep learning in computational antibody discovery
BRIEFINGS IN BIOINFORMATICS(2022)
摘要
Antibodies are versatile molecular binders with an established and growing role as therapeutics. Computational approaches to developing and designing these molecules are being increasingly used to complement traditional lab-based processes. Nowadays, in silico methods fill multiple elements of the discovery stage, such as characterizing antibody-antigen interactions and identifying developability liabilities. Recently, computational methods tackling such problems have begun to follow machine learning paradigms, in many cases deep learning specifically. This paradigm shift offers improvements in established areas such as structure or binding prediction and opens up new possibilities such as language-based modeling of antibody repertoires or machine-learning-based generation of novel sequences. In this review, we critically examine the recent developments in (deep) machine learning approaches to therapeutic antibody design with implications for fully computational antibody design.
更多查看译文
关键词
antibody,drug discovery,machine learning,deep learning,artificial intelligence,immunoinformatics
AI 理解论文
溯源树
样例
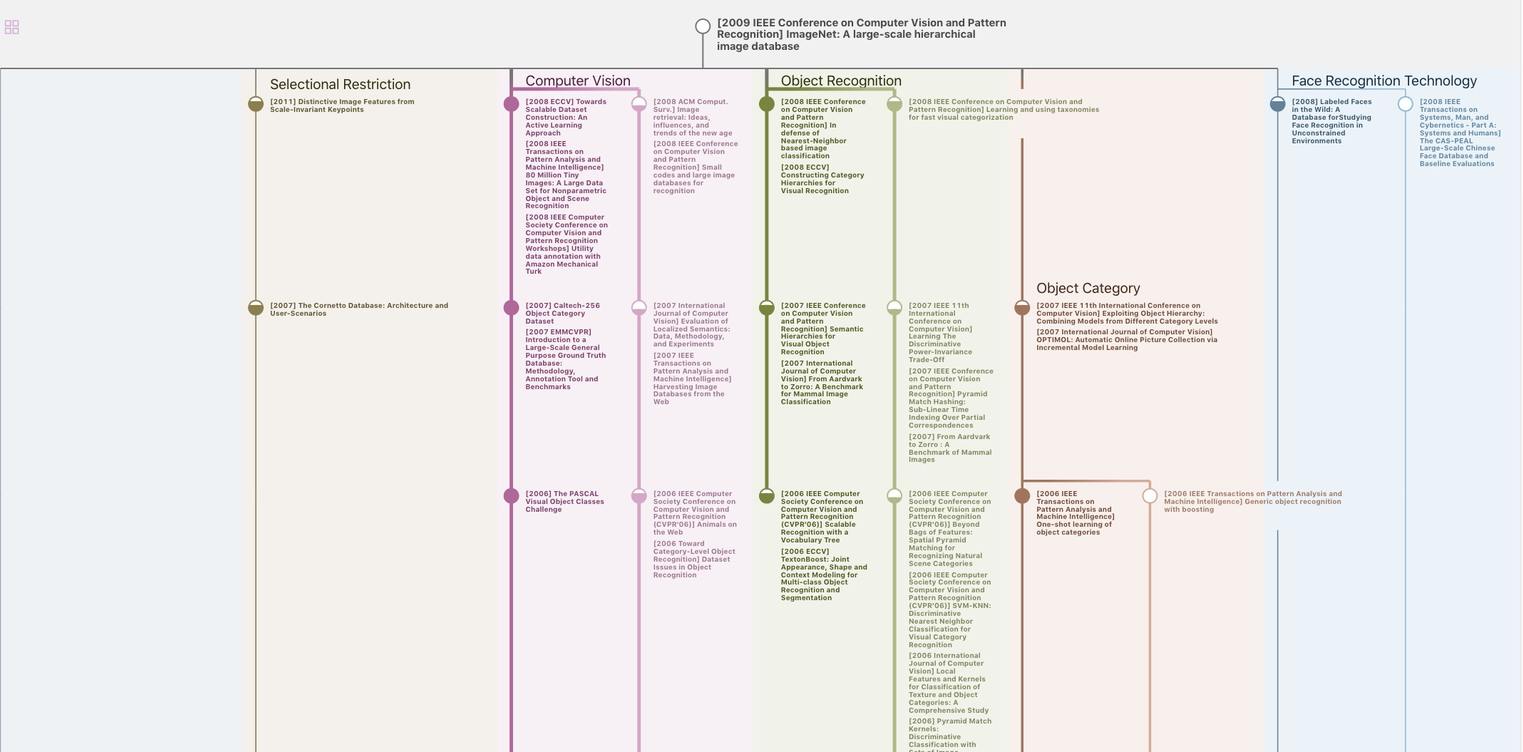
生成溯源树,研究论文发展脉络
Chat Paper
正在生成论文摘要