The DLCC Node Classification Benchmark for Analyzing Knowledge Graph Embeddings.
IEEE International Semantic Web Conference(2022)
摘要
Knowledge graph embedding is a representation learning technique that projects entities and relations in a knowledge graph to continuous vector spaces. Embeddings have gained a lot of uptake and have been heavily used in link prediction and other downstream prediction tasks. Most approaches are evaluated on a single task or a single group of tasks to determine their overall performance. The evaluation is then assessed in terms of how well the embedding approach performs on the task at hand. Still, it is hardly evaluated (and often not even deeply understood) what information the embedding approaches are actually learning to represent. To fill this gap, we present the DLCC (Description Logic Class Constructors) benchmark, a resource to analyze embedding approaches in terms of which kinds of classes they can represent. Two gold standards are presented, one based on the real-world knowledge graph DBpedia and one synthetic gold standard. In addition, an evaluation framework is provided that implements an experiment protocol so that researchers can directly use the gold standard. To demonstrate the use of DLCC, we compare multiple embedding approaches using the gold standards. We find that many DL constructors on DBpedia are actually learned by recognizing different correlated patterns than those defined in the gold standard and that specific DL constructors, such as cardinality constraints, are particularly hard to be learned for most embedding approaches.
更多查看译文
关键词
Knowledge graph embedding,Node classification,Description logics,Benchmark,Evaluation framework
AI 理解论文
溯源树
样例
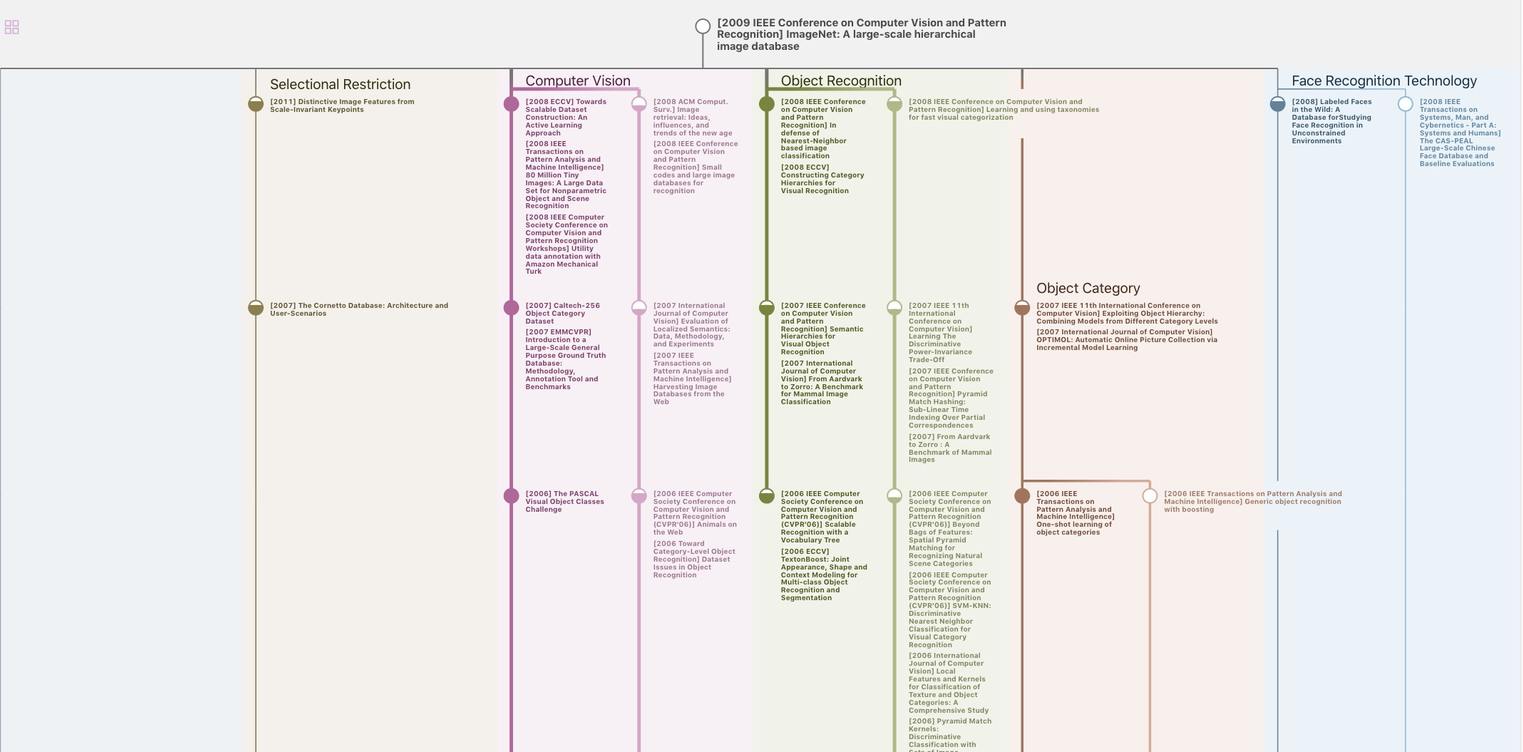
生成溯源树,研究论文发展脉络
Chat Paper
正在生成论文摘要