Wayformer: Motion Forecasting via Simple & Efficient Attention Networks
2023 IEEE INTERNATIONAL CONFERENCE ON ROBOTICS AND AUTOMATION, ICRA(2023)
摘要
Motion forecasting for autonomous driving is a challenging task because complex driving scenarios involve a heterogeneous mix of static and dynamic inputs. It is an open problem how best to represent and fuse information about road geometry, lane connectivity, time-varying traffic light state, and history of a dynamic set of agents and their interactions into an effective encoding. To model this diverse set of input features, many approaches proposed to design an equally complex system with a diverse set of modality specific modules. This results in systems that are difficult to scale, extend, or tune in rigorous ways to trade off quality and efficiency. In this paper, we present Wayformer, a family of simple and homogeneous attention based architectures for motion forecasting. Wayformer offers a compact model description consisting of an attention based scene encoder and a decoder. In the scene encoder we study the choice of early, late and hierarchical fusion of input modalities. For each fusion type we explore strategies to trade off efficiency and quality via factorized attention or latent query attention. We show that early fusion, despite its simplicity, is not only modality agnostic but also achieves state-of-the-art results on both Waymo Open Motion Dataset (WOMD) and Argoverse leaderboards, demonstrating the effectiveness of our design philosophy.
更多查看译文
AI 理解论文
溯源树
样例
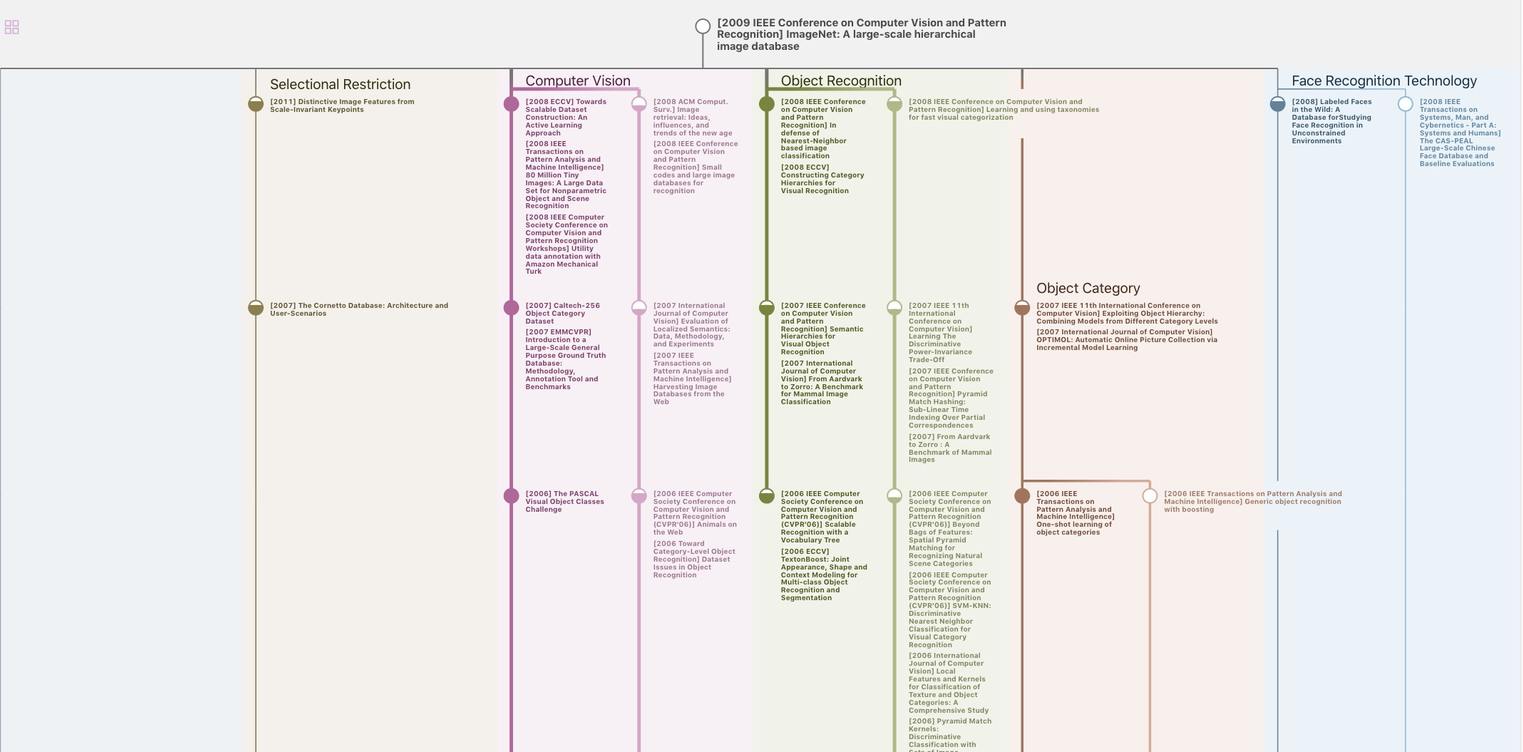
生成溯源树,研究论文发展脉络
Chat Paper
正在生成论文摘要