NRNet: Retinex Decomposition with Realistic Noise
2022 7th International Conference on Intelligent Computing and Signal Processing (ICSP)(2022)
摘要
Computer vision tasks fail in low-light environments, so high-quality restoration of low-light images is of great significance to improve the robustness of computer vision. Traditional low-light enhancement algorithms use statistical methods or need to manually adjust parameters, which will fail in poor enhancement effect and robustness. Based on the deep learning method and the Retinex method, this paper proposes a self-supervised low-light enhancement algorithm based on the attention mechanism. We decouple the illumination component and reflection component of Retinex, and then synthesize it into an input RGB image, and finally use the estimated components to adaptively adjust different areas of the image. In experiments, we use several different datasets to test our method. Experiments show that our method is able to enhance low-light images under various scene conditions.
更多查看译文
关键词
low-light,self-supervised,Retinex,attention mechanism
AI 理解论文
溯源树
样例
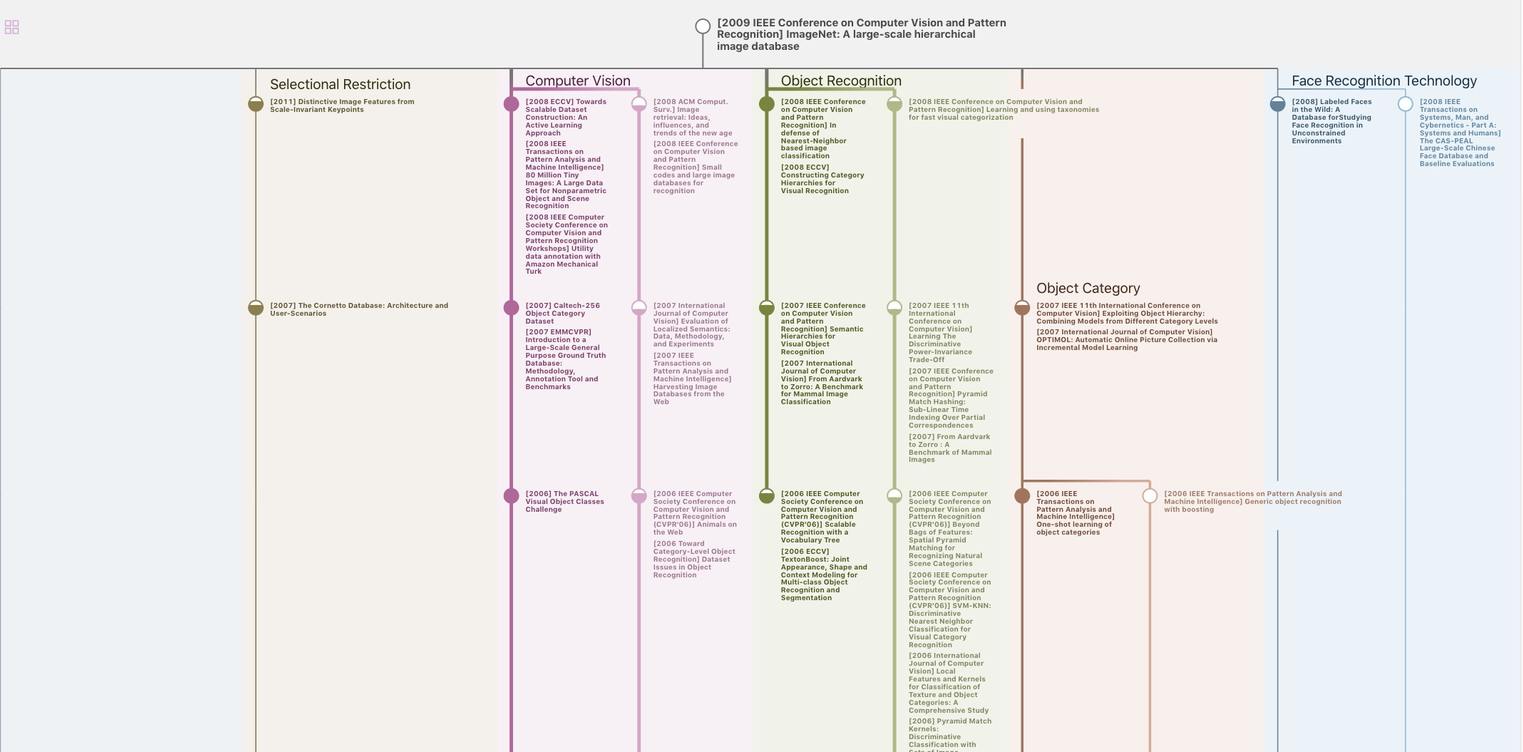
生成溯源树,研究论文发展脉络
Chat Paper
正在生成论文摘要