Personalized Federated Learning with Adaptive Batchnorm for Healthcare
IEEE Transactions on Big Data(2022)
摘要
There is a growing interest in applying machine learning techniques to healthcare. Recently, federated machine learning (FL) is gaining popularity since it allows researchers to train powerful models without compromising data privacy and security. However, the performance of existing FL approaches often deteriorates when encountering non-iid situations where there exist distribution gaps among clients, and few previous efforts focus on personalization in healthcare. In this article, we propose FedAP to tackle domain shifts and obtain personalized models for local clients. FedAP learns the similarity between clients via the statistics of the batch normalization layers while preserving the specificity of each client with different local batch normalization. Comprehensive experiments on five healthcare benchmarks demonstrate that FedAP achieves better accuracy compared to state-of-the-art methods (e.g., 10%+ accuracy improvement for PAMAP2) with faster convergence speed.
更多查看译文
关键词
Distributed Computing,Federated Learning,Personalization,Batch Normalization,Healthcare
AI 理解论文
溯源树
样例
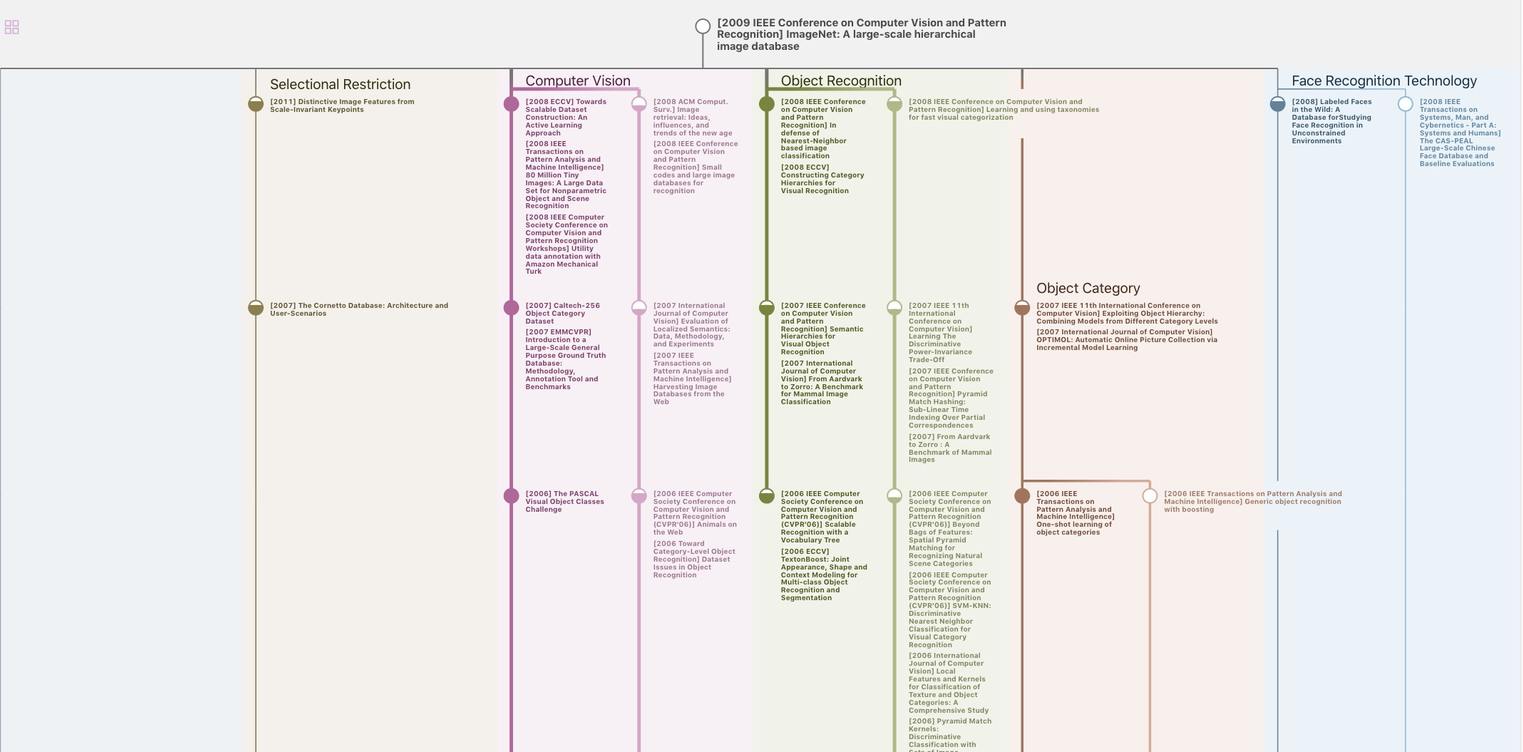
生成溯源树,研究论文发展脉络
Chat Paper
正在生成论文摘要