Model-Based Quantitative Elasticity Reconstruction Using ADMM
IEEE Transactions on Medical Imaging(2022)
摘要
We introduce two model-based iterative methods to obtain shear modulus images of tissue using magnetic resonance elastography. The first method jointly finds the displacement field that best fits tissue displacement data and the corresponding shear modulus. The displacement satisfies a viscoelastic wave equation constraint, discretized using the finite element method. Sparsifying regularization terms in both shear modulus and displacement are used in the cost function minimized for the best fit. The second method extends the first method for multifrequency tissue displacement data. The formulated problems are bi-convex. Their solution can be obtained iteratively by using the alternating direction method of multipliers. Sparsifying regularizations and the wave equation constraint filter out sensor noise and compressional waves. Our methods do not require bandpass filtering as a preprocessing step and converge fast irrespective of the initialization. We evaluate our new methods in multiple in silico and phantom experiments, with comparisons with existing methods, and we show improvements in contrast to noise and signal-to-noise ratios. Results from an in vivo liver imaging study show elastograms with mean elasticity comparable to other values reported in the literature.
更多查看译文
关键词
Elasticity,Phantoms, Imaging,Elasticity Imaging Techniques,Algorithms,Signal-To-Noise Ratio
AI 理解论文
溯源树
样例
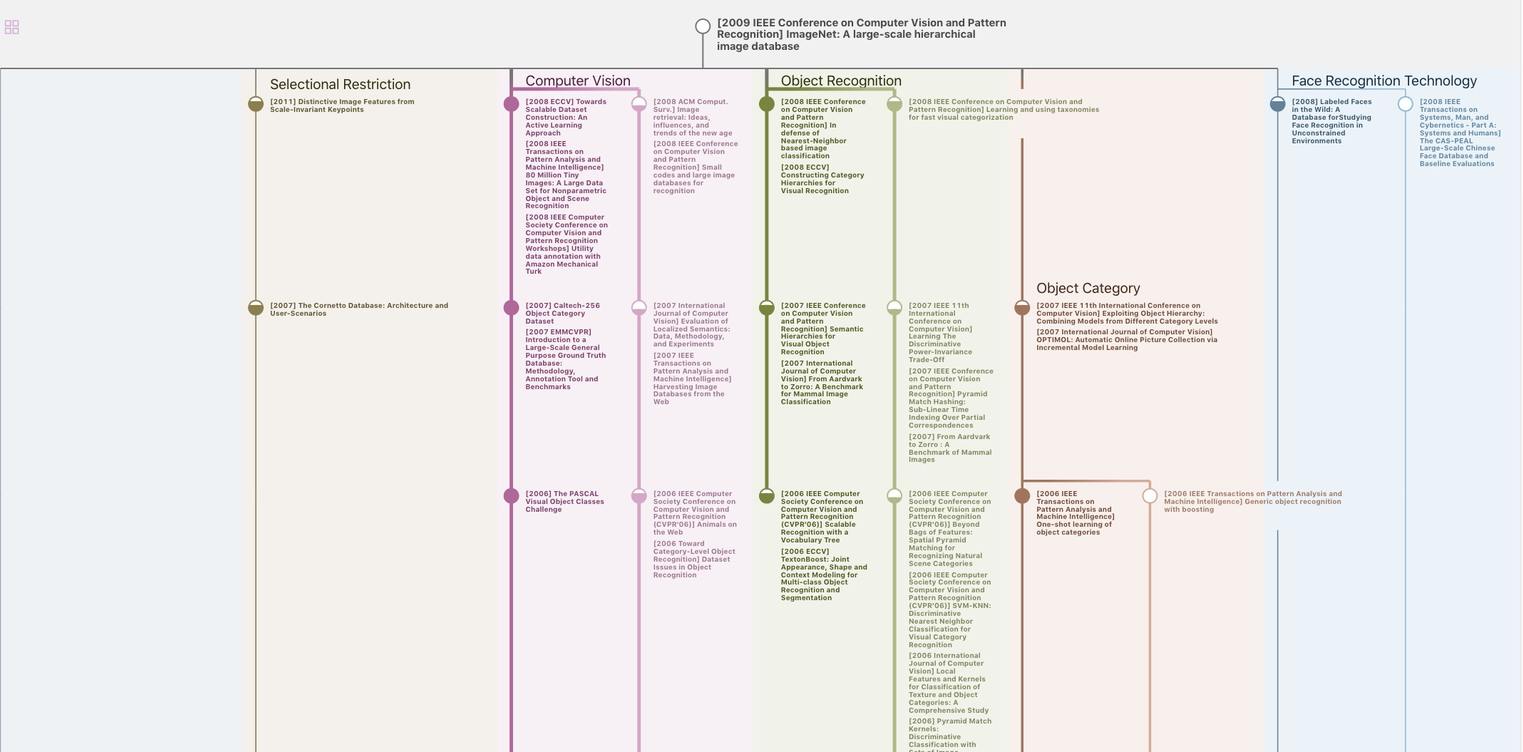
生成溯源树,研究论文发展脉络
Chat Paper
正在生成论文摘要