TD-Sampler: Learning a Training Difficulty Based Sampling Strategy for Few-Shot Object Detection
2022 7th International Conference on Cloud Computing and Big Data Analytics (ICCCBDA)(2022)
摘要
Success of deep learning on image applications heavily relies on tremendous data. Since collecting sufficient data is difficult for certain applications, e.g., medical image processing, few-shot learning has recently become a more attractive research field in deep learning community. For few-shot object detection, previous methods popularly finetunes a pretrained detector for data-scarce novel classes using a small number of data-abundant base class data. To effectively adopt all base class data in the finetuning stage while overcoming incurred imbalanced learning, we propose a training difficulty (TD) criterion for training data in this paper. Training data with a bigger TD more probably changes a detector's activation pattern significantly, compared to data with a smaller TD. Since the TD criterion requires time-consuming backpropagation information, we learn a TD predic-tor with the detector in the pretraining stage to accelerate TD computation. Based on the TD predictor, we propose a sampling strategy which prefers a batch of training data with more novel class data and lower probability of incurring significant changes on the detector's activation pattern. Experimental results on two popular datasets Pascal VOC and MSCOCO show that for novel class data, our proposed method, TD-Sampler, achieves state-of-the-art on Pascal VOC, and improves mAP averagely by 22.9% on MSCOCO compared to the previous best method. Ablation study further shows that averaged accuracy drop of ours without TD based sampling is 21.3% compared to ours. Therefore, the TD based sampling is a crucial component of our proposed method.
更多查看译文
关键词
Few-shot learning,object detection,sampling,training difficulty,activation pattern
AI 理解论文
溯源树
样例
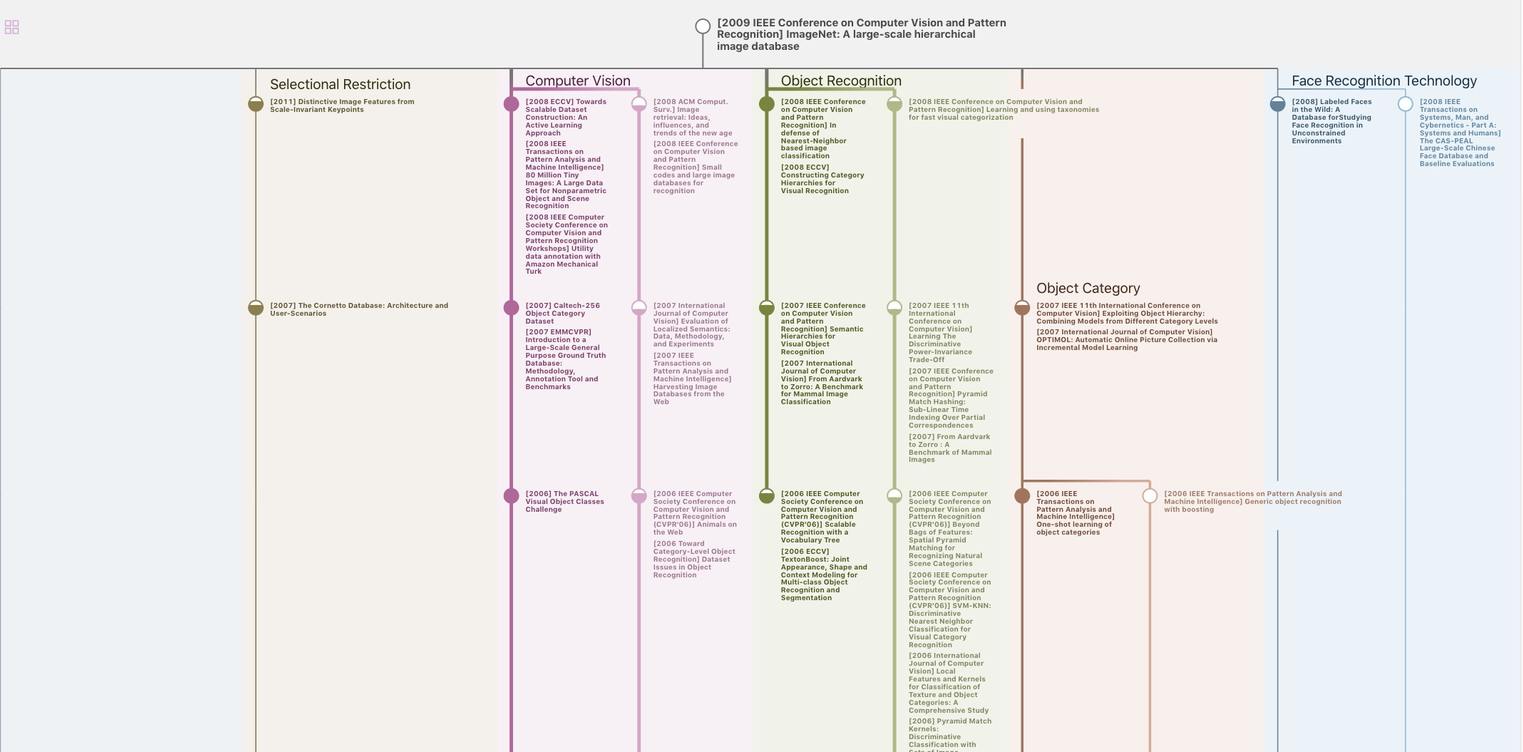
生成溯源树,研究论文发展脉络
Chat Paper
正在生成论文摘要