The Contribution of Lyrics and Acoustics to Collaborative Understanding of Mood
International Conference on Web and Social Media (ICWSM)(2022)
摘要
In this work, we study the association between song lyrics and mood through a data-driven analysis. Our data set consists of nearly one million songs, with song-mood associations derived from user playlists on the Spotify streaming platform. We take advantage of state-of-the-art natural language processing models based on transformers to learn the association between the lyrics and moods. We find that a pretrained transformer-based language model in a zero-shot setting -- i.e., out of the box with no further training on our data -- is powerful for capturing song-mood associations. Moreover, we illustrate that training on song-mood associations results in a highly accurate model that predicts these associations for unseen songs. Furthermore, by comparing the prediction of a model using lyrics with one using acoustic features, we observe that the relative importance of lyrics for mood prediction in comparison with acoustics depends on the specific mood. Finally, we verify if the models are capturing the same information about lyrics and acoustics as humans through an annotation task where we obtain human judgments of mood-song relevance based on lyrics and acoustics.
更多查看译文
关键词
mood,lyrics,acoustics,collaborative understanding
AI 理解论文
溯源树
样例
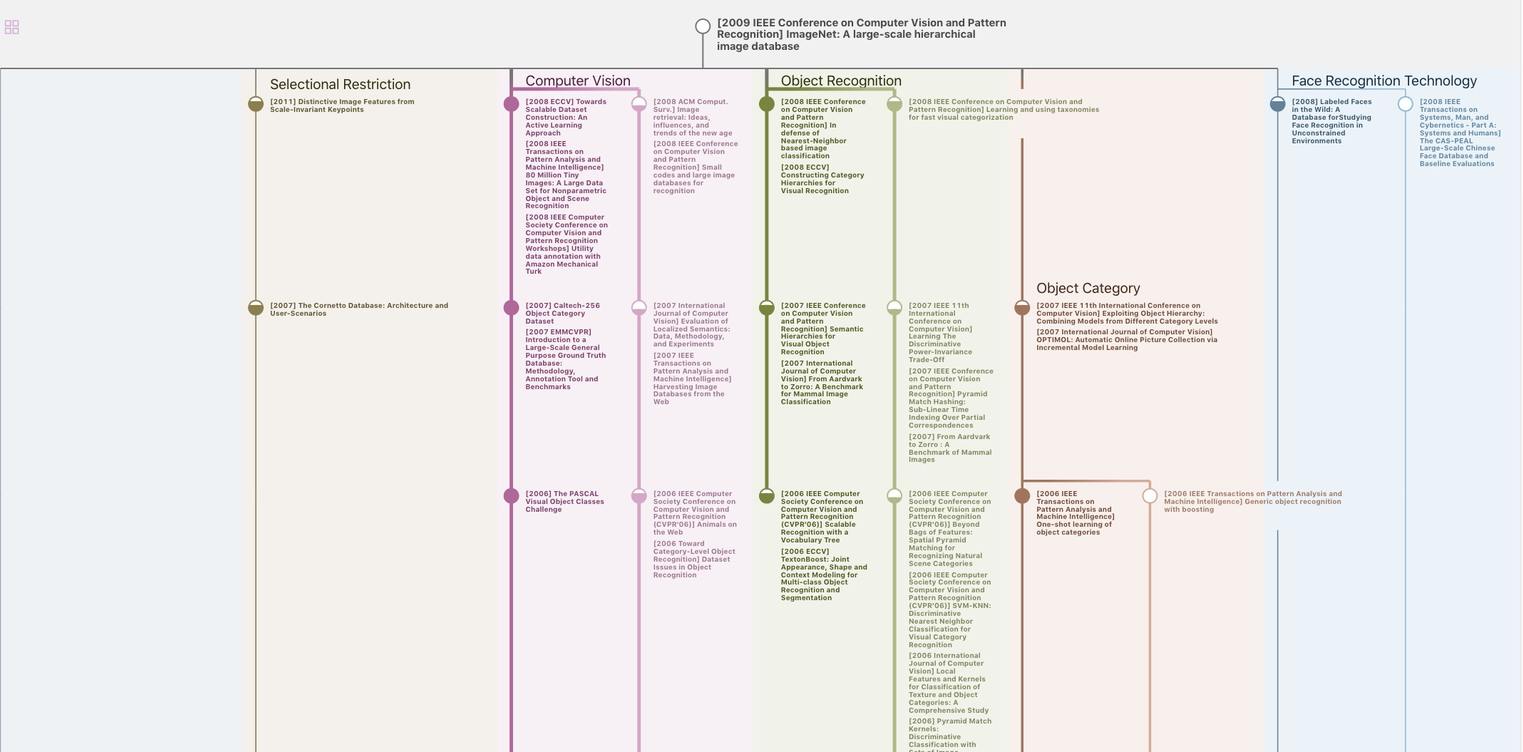
生成溯源树,研究论文发展脉络
Chat Paper
正在生成论文摘要