FedHAP: Federated Hashing With Global Prototypes for Cross-Silo Retrieval
IEEE TRANSACTIONS ON PARALLEL AND DISTRIBUTED SYSTEMS(2024)
摘要
Deep hashing has been widely applied in large-scale data retrieval due to its superior retrieval efficiency and low storage cost. However, data are often scattered in data silos with privacy concerns, so performing centralized data storage and retrieval is not always possible. Leveraging the approach of federated learning (FL) to perform deep hashing is a recent research trend. However, existing frameworks mostly rely on the aggregation of the local deep hashing models, which are trained by performing similarity learning with local skewed data only. Therefore, they cannot work well for non-IID clients in a real federated environment. To overcome these challenges, we propose a novel federated hashing framework that enables participating clients to jointly train a shared deep hashing model by leveraging the class-wise prototypical hash codes. Globally, sharing global prototypes with only one prototypical hash code per class assists in learning consistent code distributions across clients while minimizing the cost of communication and privacy. Locally, the use of the global prototypical hash codes are maximized by jointly training a discriminator network and the local hashing network. Extensive experiments on benchmark datasets are conducted to demonstrate that our method can significantly improve the performance of the deep hashing model in the federated environments with non-IID data distributions.
更多查看译文
关键词
Federated learning,information retrieval,deep hashing
AI 理解论文
溯源树
样例
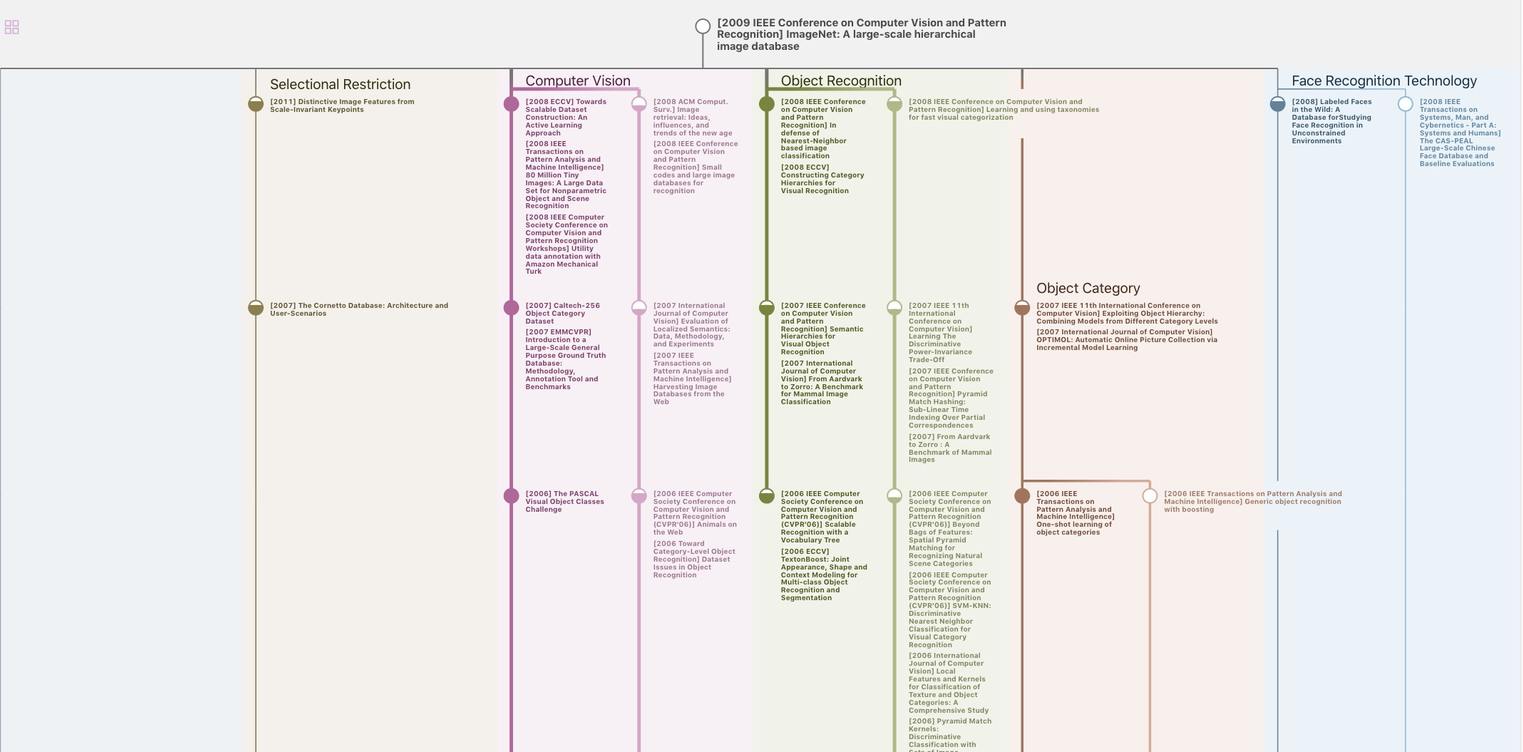
生成溯源树,研究论文发展脉络
Chat Paper
正在生成论文摘要