Transferability-Guided Cross-Domain Cross-Task Transfer Learning
IEEE TRANSACTIONS ON NEURAL NETWORKS AND LEARNING SYSTEMS(2024)
摘要
We propose two novel transferability metrics fast optimal transport-based conditional entropy (F-OTCE) and joint correspondence OTCE (JC-OTCE) to evaluate how much the source model (task) can benefit the learning of the target task and to learn more generalizable representations for cross-domain cross-task transfer learning. Unlike the original OTCE metric that requires evaluating the empirical transferability on auxiliary tasks, our metrics are auxiliary-free such that they can be computed much more efficiently. Specifically, F-OTCE estimates transferability by first solving an optimal transport (OT) problem between source and target distributions and then uses the optimal coupling to compute the negative conditional entropy (NCE) between the source and target labels. It can also serve as an objective function to enhance downstream transfer learning tasks including model finetuning and domain generalization (DG). Meanwhile, JC-OTCE improves the transferability accuracy of F-OTCE by including label distances in the OT problem, though it incurs additional computation costs. Extensive experiments demonstrate that F-OTCE and JC-OTCE outperform state-of-the-art auxiliary-free metrics by 21.1% and 25.8% , respectively, in correlation coefficient with the ground-truth transfer accuracy. By eliminating the training cost of auxiliary tasks, the two metrics reduce the total computation time of the previous method from 43 min to 9.32 and 10.78 s, respectively, for a pair of tasks. When applied in the model finetuning and DG tasks, F-OTCE shows significant improvements in the transfer accuracy in few-shot classification experiments, with up to 4.41% and 2.34% accuracy gains, respectively.
更多查看译文
关键词
Cross-domain,cross-task,few-shot learning,source selection,task relatedness,transfer learning,transferability estimation
AI 理解论文
溯源树
样例
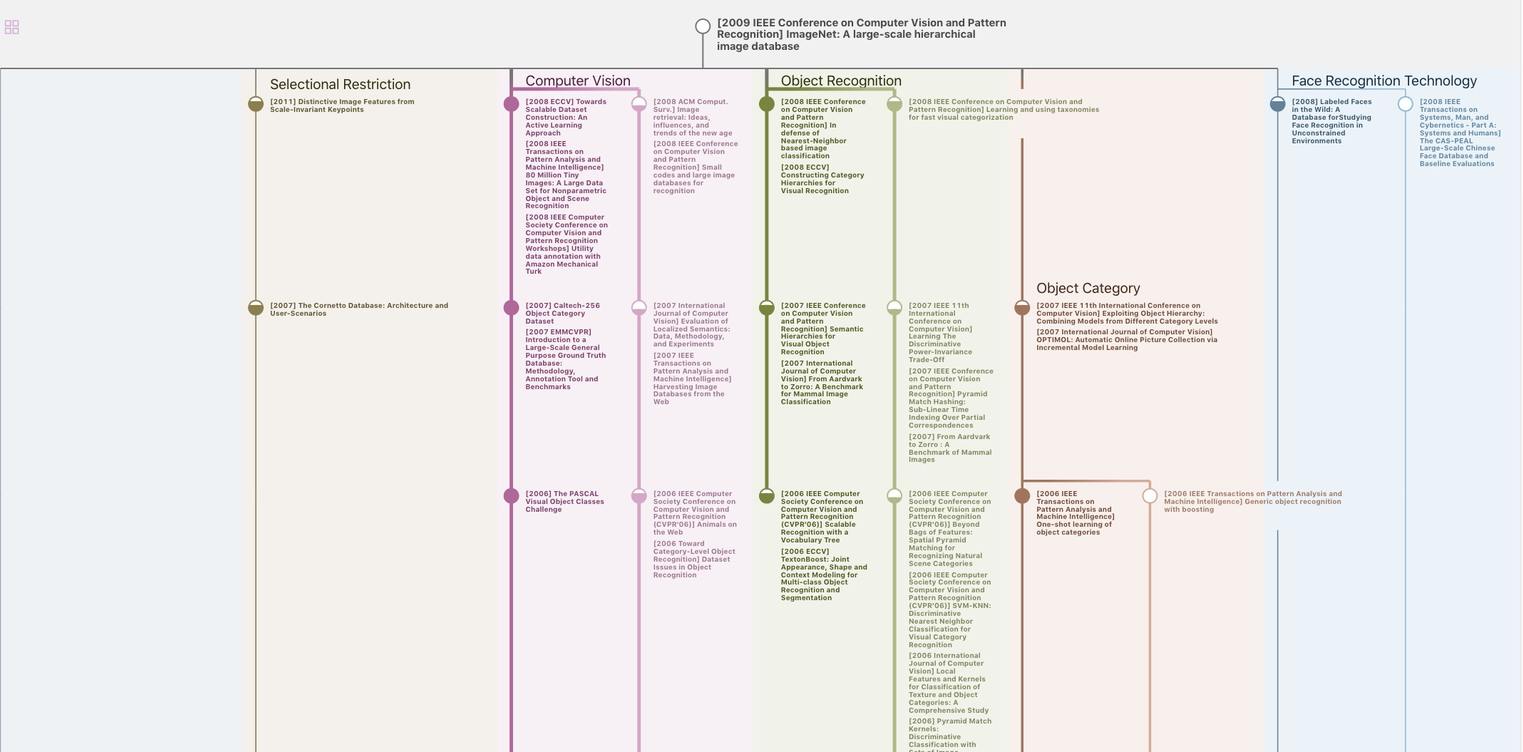
生成溯源树,研究论文发展脉络
Chat Paper
正在生成论文摘要