Learning Diverse Tone Styles for Image Retouching
IEEE TRANSACTIONS ON IMAGE PROCESSING(2024)
摘要
Image retouching, aiming to regenerate the visually pleasing renditions of given images, is a subjective task where the users are with different aesthetic sensations. Most existing methods adopt a deterministic model to learn the retouching style from a specific expert, making it less flexible to meet diverse subjective preferences. Besides, the intrinsic diversity of an expert due to the targeted processing of different images is also deficiently described. To circumvent such issues, we propose to learn diverse image retouching with normalizing flow-based architectures. Unlike current flow-based methods which directly generate the output image, we argue that learning in a one-dimensional style space could 1) disentangle the retouching styles from the image content, 2) lead to a stable style presentation form, and 3) avoid the spatial disharmony effects. For obtaining meaningful image tone style representations, a joint-training pipeline is delicately designed, which is composed of a style encoder, a conditional RetouchNet, and the image tone style normalizing flow (TSFlow) module. In particular, the style encoder predicts the target style representation of an input image, which serves as the conditional information in the RetouchNet for retouching, while the TSFlow maps the style representation vector into a Gaussian distribution in the forward pass. After training, the TSFlow can generate diverse image tone style vectors by sampling from the Gaussian distribution. Extensive experiments on MIT-Adobe FiveK and PPR10K datasets show that our proposed method performs favorably against state-of-the-art methods and is effective in generating diverse results to satisfy different human aesthetic preferences.
更多查看译文
关键词
Image enhancement,image retouching,normalizing flow
AI 理解论文
溯源树
样例
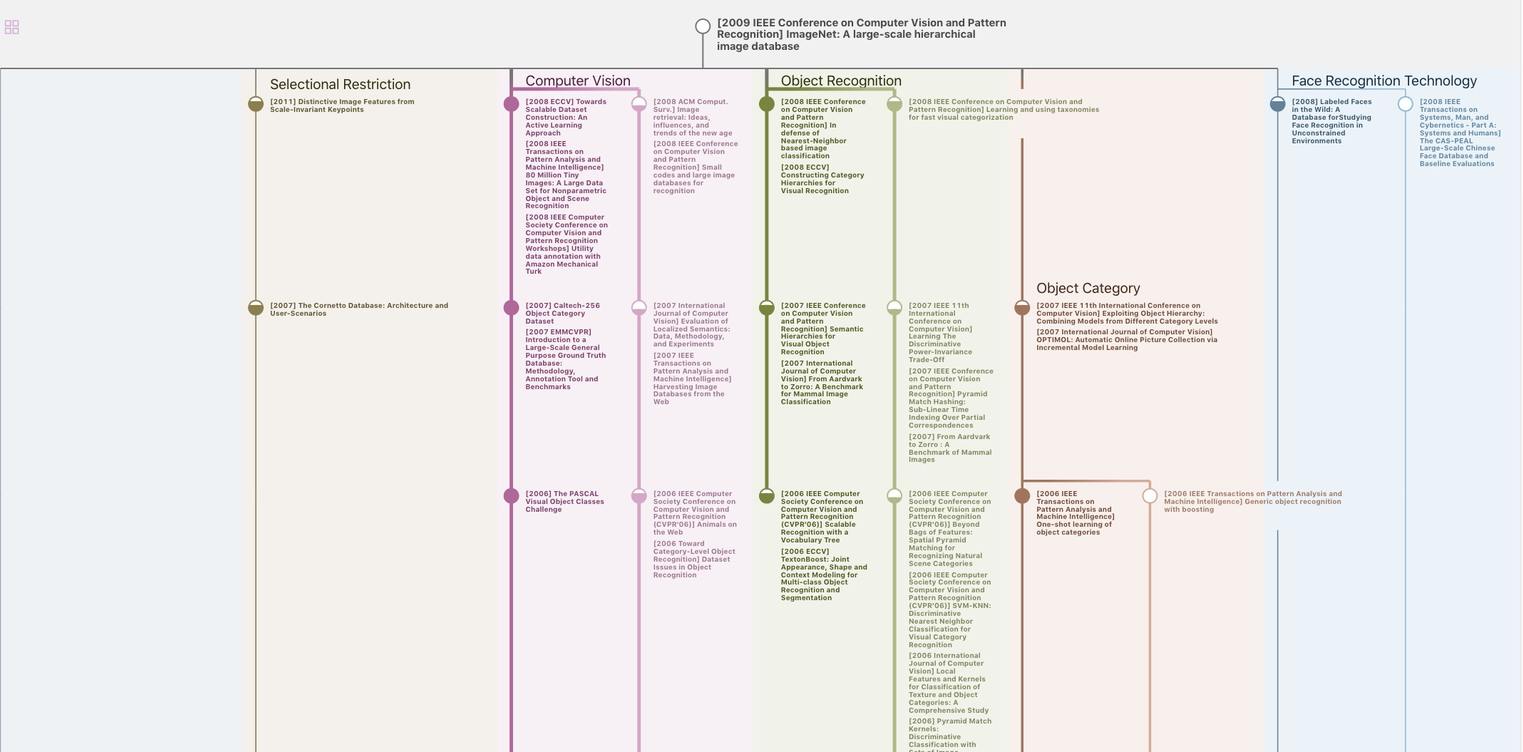
生成溯源树,研究论文发展脉络
Chat Paper
正在生成论文摘要