Outpainting by Queries
arxiv(2022)
摘要
Image outpainting, which is well studied with Convolution Neural Network (CNN) based framework, has recently drawn more attention in computer vision. However, CNNs rely on inherent inductive biases to achieve effective sample learning, which may degrade the performance ceiling. In this paper, motivated by the flexible self-attention mechanism with minimal inductive biases in transformer architecture, we reframe the generalised image outpainting problem as a patch-wise sequence-to-sequence autoregression problem, enabling query-based image outpainting. Specifically, we propose a novel hybrid vision-transformer-based encoder-decoder framework, named \textbf{Query} \textbf{O}utpainting \textbf{TR}ansformer (\textbf{QueryOTR}), for extrapolating visual context all-side around a given image. Patch-wise mode's global modeling capacity allows us to extrapolate images from the attention mechanism's query standpoint. A novel Query Expansion Module (QEM) is designed to integrate information from the predicted queries based on the encoder's output, hence accelerating the convergence of the pure transformer even with a relatively small dataset. To further enhance connectivity between each patch, the proposed Patch Smoothing Module (PSM) re-allocates and averages the overlapped regions, thus providing seamless predicted images. We experimentally show that QueryOTR could generate visually appealing results smoothly and realistically against the state-of-the-art image outpainting approaches.
更多查看译文
关键词
Image outpainting,Transformer,Query expanding
AI 理解论文
溯源树
样例
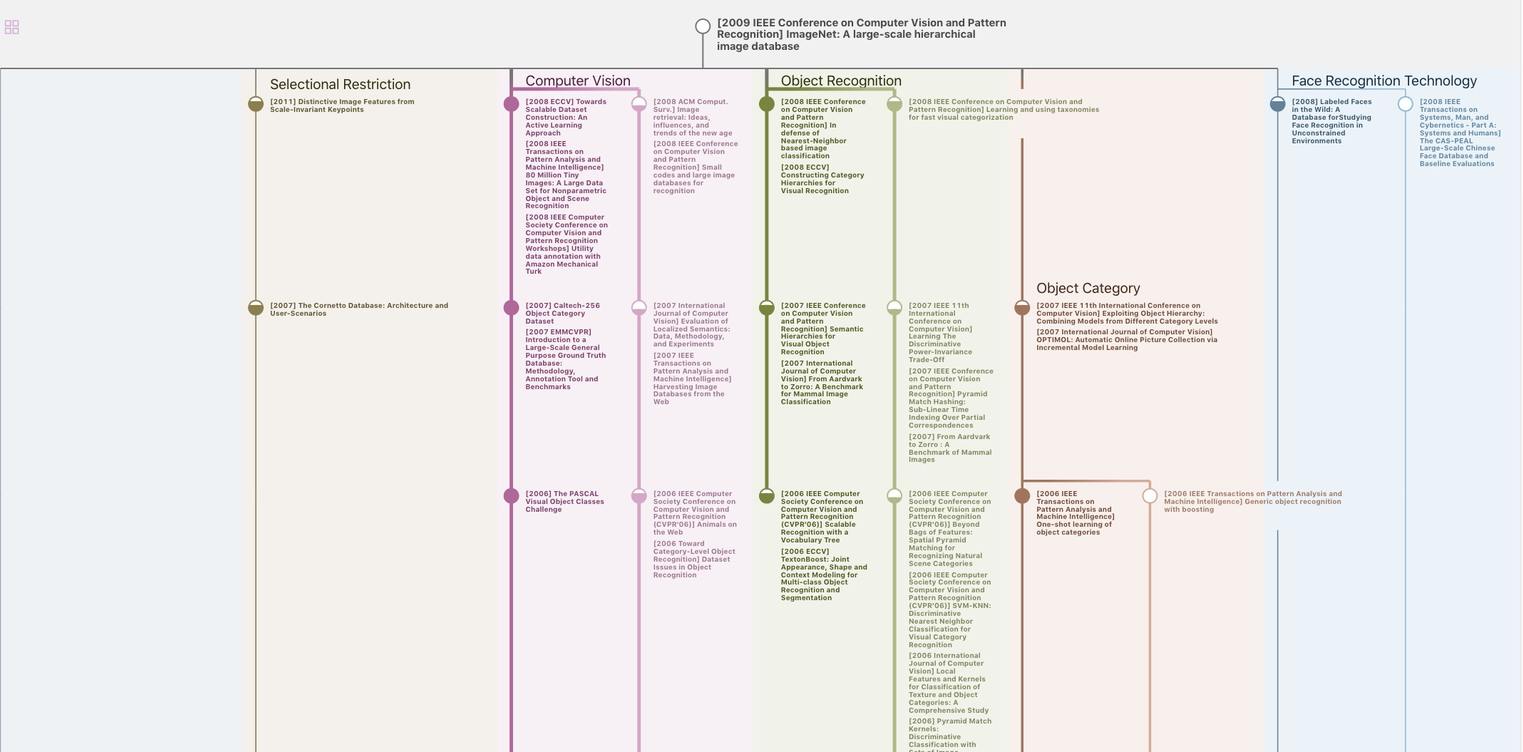
生成溯源树,研究论文发展脉络
Chat Paper
正在生成论文摘要