Trusted multi-scale classification framework for whole slide image
BIOMEDICAL SIGNAL PROCESSING AND CONTROL(2024)
摘要
Despite remarkable efforts, the classification of gigapixel whole-slide images (WSIs) is severely restricted by either limited computing resources or inadequate utilization of knowledge from different scales. Furthermore, previous attempts have often lacked the ability to estimate uncertainty for different scales. Typically, pathologists jointly analyze WSIs at different magnifications, changing the magnification repeatedly when uncertain to discover various tissue features. Motivated by this diagnostic process, we propose a trusted multi-scale classification framework for WSIs. Using the Vision Transformer as the backbone for multi-branches, our framework models joint classification, estimates uncertainty for each magnification of a microscope, and integrates evidence from different magnifications. Additionally, we propose a novel patch selection schema using attention rollout and non-maximum suppression to exploit discriminative patches from WSIs and reduce computational requirements. To empirically investigate the effectiveness of our approach, we conducted experiments on two benchmark databases for WSI classification. The results suggest that our trusted framework significantly improves WSI classification performance compared to state-of-the-art methods.
更多查看译文
关键词
Histopathological images analysis,Whole slide images,Trusted multi-scale classification,Uncertainty estimation
AI 理解论文
溯源树
样例
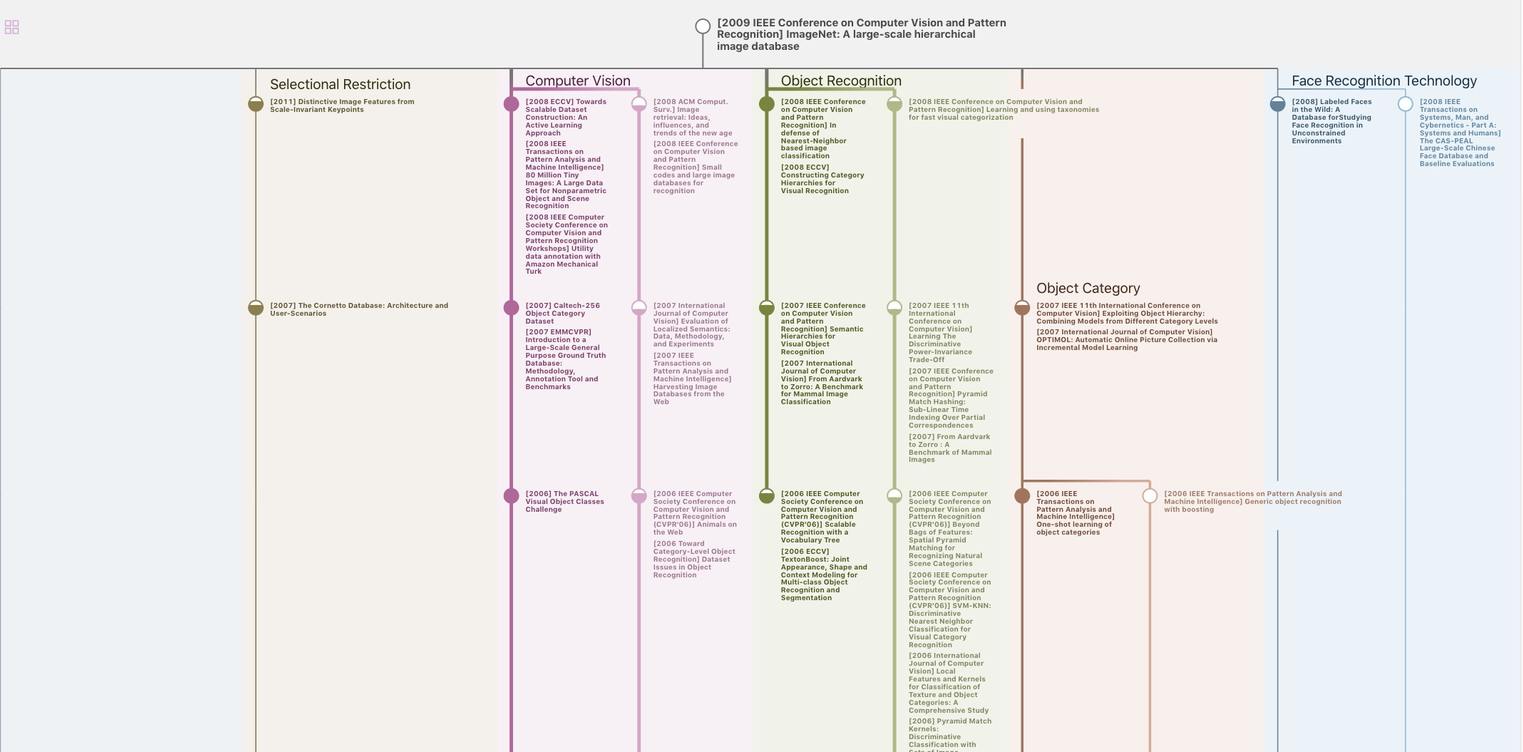
生成溯源树,研究论文发展脉络
Chat Paper
正在生成论文摘要