Improved Inference in Financial Factor Models
SSRN Electronic Journal(2022)
摘要
Conditional heteroskedasticity of the error terms is a common occurrence in financial factor models, such as the CAPM and Fama-French factor models. This feature necessitates the use of heteroskedasticity consistent (HC) standard errors to make valid inference for regression coefficients. In this paper, we show that using weighted least squares (WLS) or adaptive least squares (ALS) to estimate model parameters generally leads to smaller HC standard errors compared to ordinary least squares (OLS), which translates into improved inference in the form of shorter confidence intervals and more powerful hypothesis tests. In an extensive empirical analysis based on historical stock returns and commonly used factors, we find that conditional heteroskedasticity is pronounced and that WLS and ALS can dramatically shorten confidence intervals compared to OLS, especially during times of financial turmoil.
更多查看译文
关键词
codes,C12,C13,C21
AI 理解论文
溯源树
样例
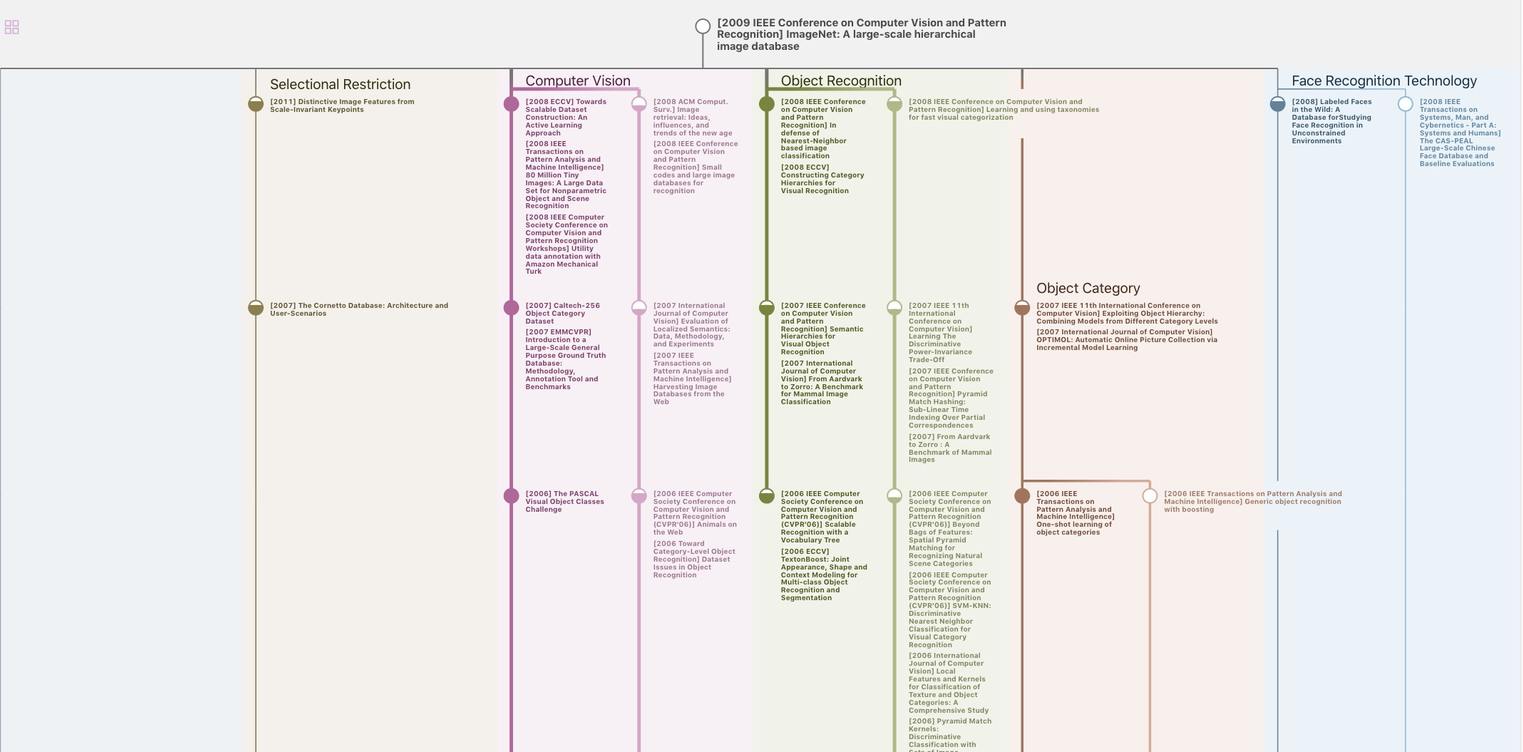
生成溯源树,研究论文发展脉络
Chat Paper
正在生成论文摘要