Model application to quantitatively evaluate placental features from ultrasound images with gestational diabetes
JOURNAL OF CLINICAL ULTRASOUND(2022)
摘要
Purpose The goal of this study was to introduce PFCnet (placental features classification network), an multimodel model for evaluating and classifying placental features in gestational diabetes mellitus (GDM) and normal late pregnancy. Deep learning algorithms could be utilized to fully automate the examination of alterations in the placenta caused by hyperglycemia. Methods A total of 718 placental ultrasound images, including 139 cases of GDM, were collected, including gray-scale images (GSIs) and microflow images (MFIs). Ultrasonic assessment parameters and perinatal features were recorded. We divided gestational age into two categories for analysis (37 weeks and 37 weeks) based on the cut-off value level of placental maturity. The PFCnet model was introduced for identifying placental characteristics from normal and GDM pregnancies after extensive training and optimization. The model was scored using metrics such as sensitivity, specificity, accuracy, and the area under the curve (AUC). Results In view of multimodal fusion (GSIs and MFIs) and deep network optimization training, the overall diagnostic performance of the PFCnet model depending on the region of interest (ROI) was excellent (AUC: 93%), with a sensitivity of 89%, a specificity of 92%, and an accuracy of 92% in the independent test set. The fusion features of GSIs and MFIs in the placenta showed a higher discriminative power than single-mode features (accuracy: Fusion 92% vs. GSIs 84% vs. MFIs 82%). The independent test set at 37 weeks exhibited a better specificity (75% vs. 69%) but a lower sensitivity(95% vs. 100%). Conclusions With its dual channel identification of placental parenchymal and vascular lesions in obstetric complications, the PFCnet classification model has the potential to be a useful tool for detecting placental tissue abnormalities caused by hyperglycemia.
更多查看译文
关键词
deep convolutional neural networks, gestational diabetes mellitus, placenta, pregnancy complications
AI 理解论文
溯源树
样例
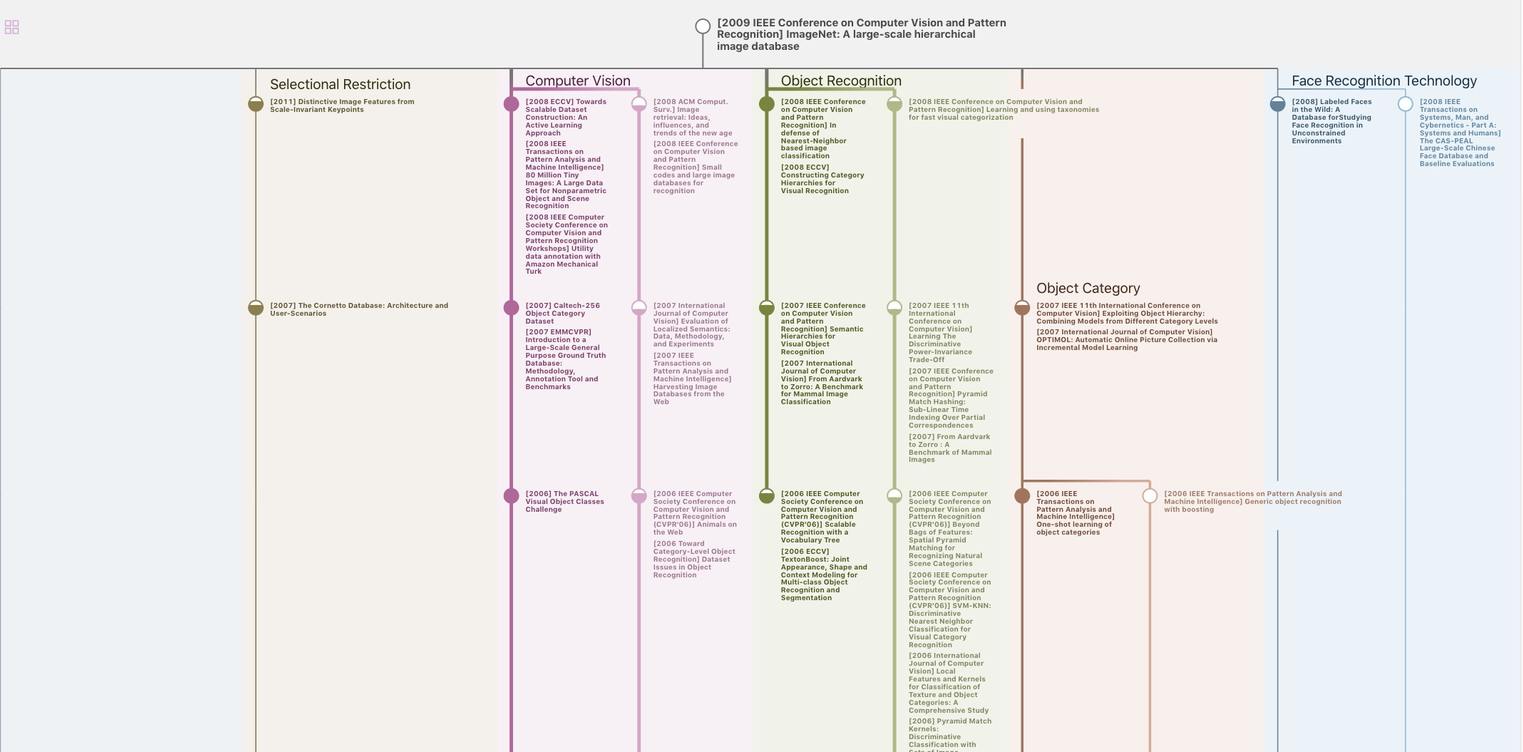
生成溯源树,研究论文发展脉络
Chat Paper
正在生成论文摘要