K-means find density peaks in molecular conformation clustering
CHINESE JOURNAL OF CHEMICAL PHYSICS(2022)
摘要
Performing cluster analysis on molecular conformation is an important way to find the representative conformation in the molecular dynamics trajectories. Usually, it is a critical step for interpreting complex conformational changes or interaction mechanisms. As one of the density-based clustering algorithms, find density peaks (FDP) is an accurate and reasonable candidate for the molecular conformation clustering. However, facing the rapidly increasing simulation length due to the increase in computing power, the low computing efficiency of FDP limits its application potential. Here we propose a marginal extension to FDP named K-means find density peaks (KFDP) to solve the mass source consuming problem. In KFDP, the points are initially clustered by a high efficiency clustering algorithm, such as K-means. Cluster centers are defined as typical points with a weight which represents the cluster size. Then, the weighted typical points are clustered again by FDP, and then are refined as core, boundary, and redefined halo points. In this way, KFDP has comparable accuracy as FDP but its computational complexity is reduced from O(n(2)) to O(n). We apply and test our KFDP method to the trajectory data of multiple small proteins in terms of torsion angle, secondary structure or contact map. The comparing results with K-means and density-based spatial clustering of applications with noise show the validation of the proposed KFDP.
更多查看译文
关键词
K-means find density peaks,Molecular clustering,Density-based spatial clustering of applications with noise
AI 理解论文
溯源树
样例
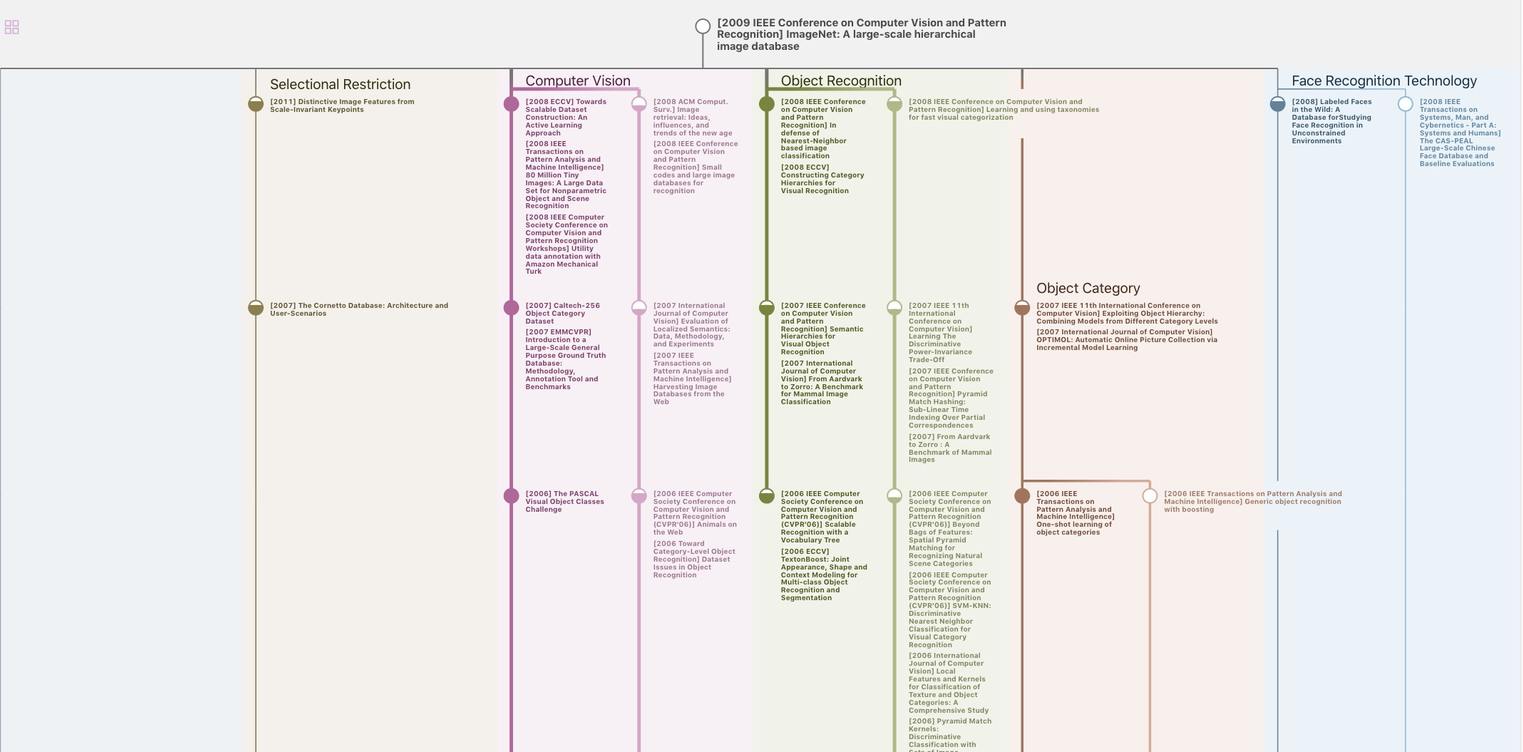
生成溯源树,研究论文发展脉络
Chat Paper
正在生成论文摘要