Dynamic Budget Throttling in Repeated Second-Price Auctions
arXiv (Cornell University)(2022)
摘要
Throttling is one of the most popular budget control methods in today's online advertising markets. When a budget-constrained advertiser employs throttling, she can choose whether to participate in an auction or not after the advertising platform recommends a bid. This paper focuses on the dynamic budget throttling process in repeated second-price auctions from a theoretical view. An essential feature of the underlying problem is that the advertiser does not know the distribution of the highest competing bid upon entering the market. To model the difficulty of eliminating such uncertainty, we consider two different information structures in order. The advertiser could obtain the highest competing bid in each round with full-information feedback. Meanwhile, with partial information feedback, the advertiser could only obtain the highest competing bid in the auctions she participates in. We propose the OGD-CB algorithm, which involves simultaneous distribution learning and revenue optimization facing online ad queries. In both settings, we demonstrate that this algorithm guarantees an $O(\sqrt{T\log T})$ regret with probability $1 - O(1/T)$ relative to the fluid adaptive throttling benchmark. By proving a lower bound of $\Omega(\sqrt{T})$ on the minimal regret for even the hindsight optimum, we establish the near optimality of our algorithm. Finally, we compare the fluid optimum of throttling to that of pacing, another widely adopted budget control method. The numerical relationship of these benchmarks benefits us with further insights into the comparison of different online algorithms for budget management.
更多查看译文
AI 理解论文
溯源树
样例
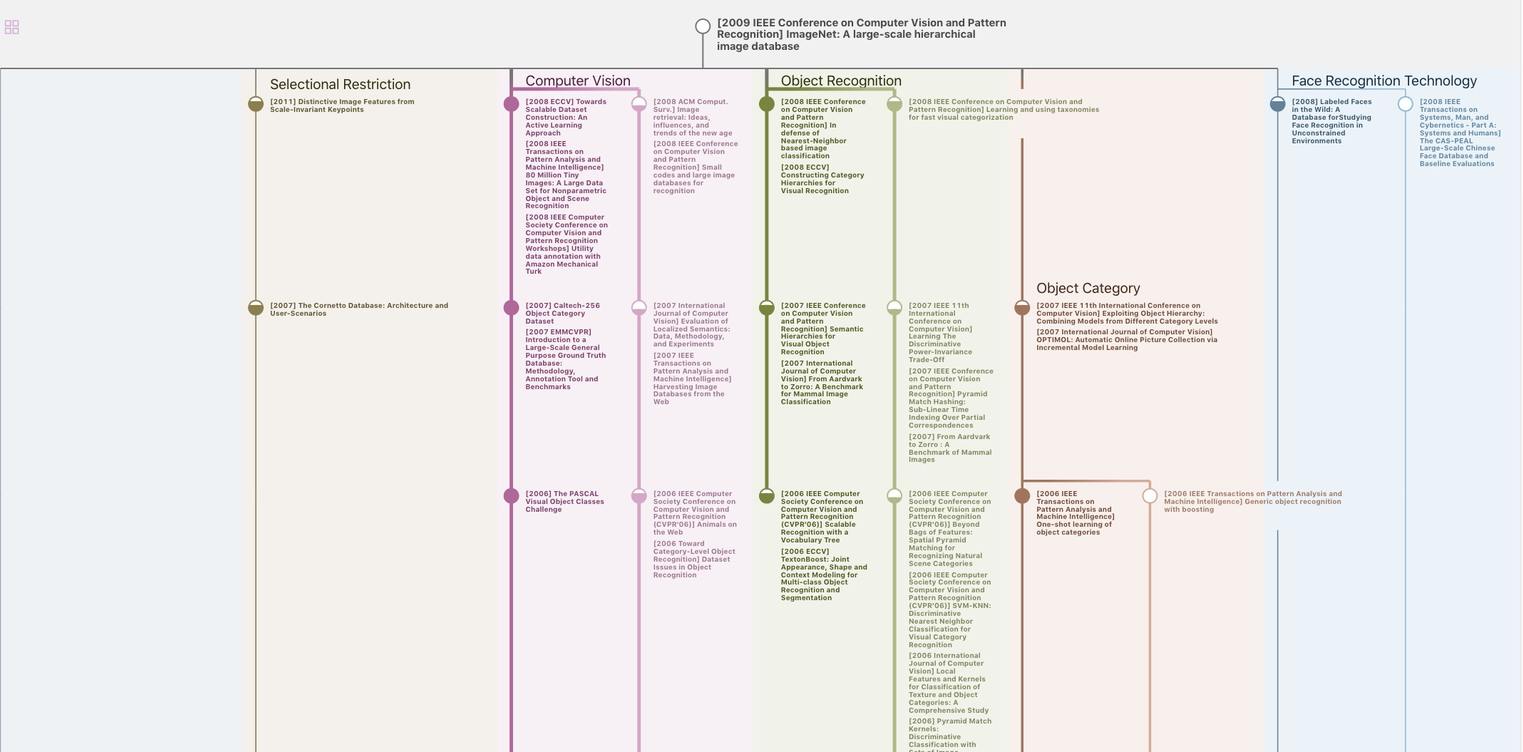
生成溯源树,研究论文发展脉络
Chat Paper
正在生成论文摘要