FairDistillation: Mitigating Stereotyping in Language Models
arxiv(2023)
摘要
Large pre-trained language models are successfully being used in a variety of tasks, across many languages. With this everincreasing usage, the risk of harmful side effects also rises, for example by reproducing and reinforcing stereotypes. However, detecting and mitigating these harms is difficult to do in general and becomes computationally expensive when tackling multiple languages or when considering different biases. To address this, we present FairDistillation: a cross-lingual method based on knowledge distillation to construct smaller language models while controlling for specific biases. We found that our distillation method does not negatively affect the downstream performance on most tasks and successfully mitigates stereotyping and representational harms. We demonstrate that FairDistillation can create fairer language models at a considerably lower cost than alternative approaches.
更多查看译文
关键词
Knowledge distillation, Fairness, BERT, Language models
AI 理解论文
溯源树
样例
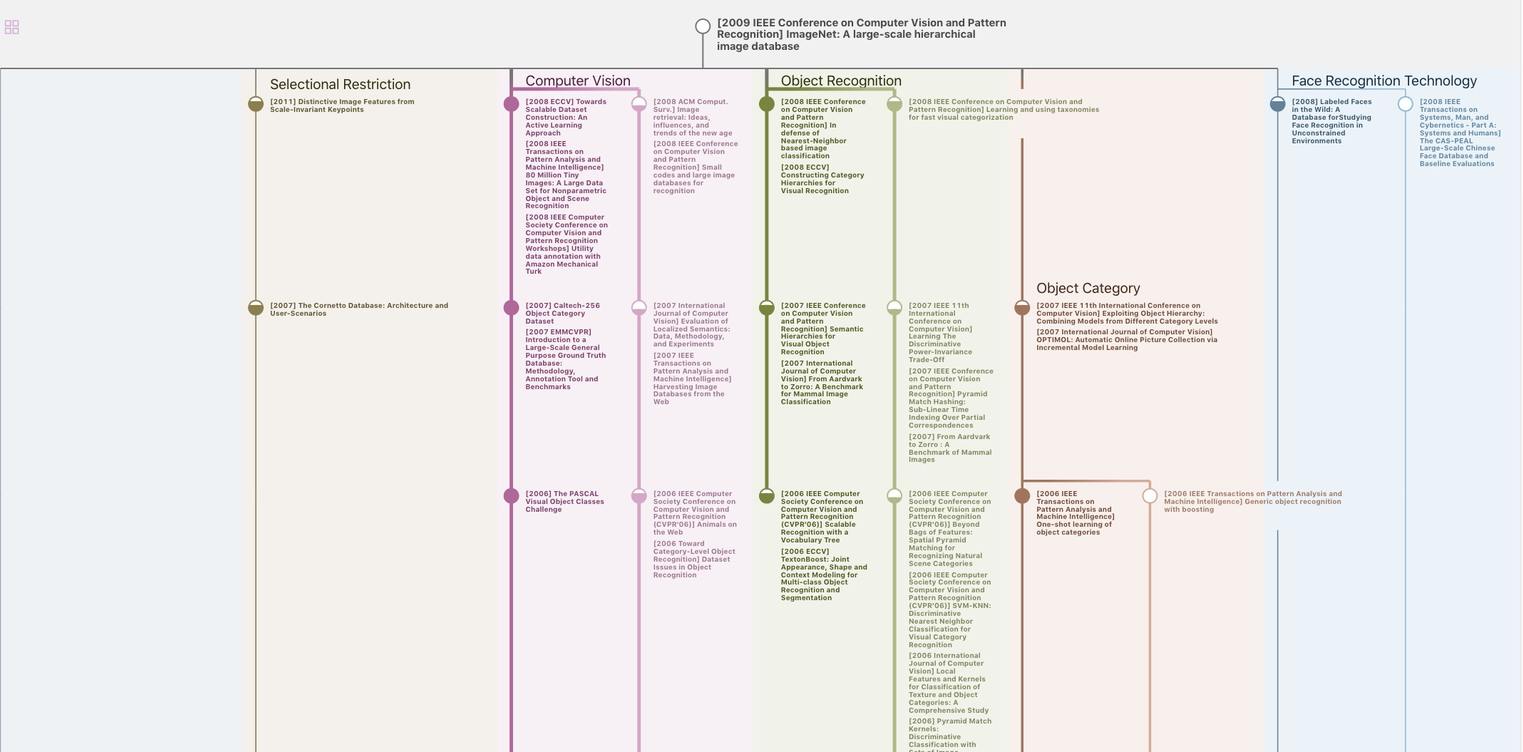
生成溯源树,研究论文发展脉络
Chat Paper
正在生成论文摘要