Learning Causal Effects on Hypergraphs
KDD '22: Proceedings of the 28th ACM SIGKDD Conference on Knowledge Discovery and Data Mining(2022)
摘要
Hypergraphs provide an effective abstraction for modeling multi-way group interactions among nodes, where each hyperedge can connect any number of nodes. Different from most existing studies which leverage statistical dependencies, we study hypergraphs from the perspective of causality. Specifically, in this paper, we focus on the problem of individual treatment effect (ITE) estimation on hypergraphs, aiming to estimate how much an intervention (e.g., wearing face covering) would causally affect an outcome (e.g., COVID-19 infection) of each individual node. Existing works on ITE estimation either assume that the outcome on one individual should not be influenced by the treatment assignments on other individuals (i.e., no interference), or assume the interference only exists between pairs of connected individuals in an ordinary graph. We argue that these assumptions can be unrealistic on real-world hypergraphs, where higher-order interference can affect the ultimate ITE estimations due to the presence of group interactions. In this work, we investigate high-order interference modeling, and propose a new causality learning framework powered by hypergraph neural networks. Extensive experiments on real-world hypergraphs verify the superiority of our framework over existing baselines.
更多查看译文
关键词
causal effects,learning
AI 理解论文
溯源树
样例
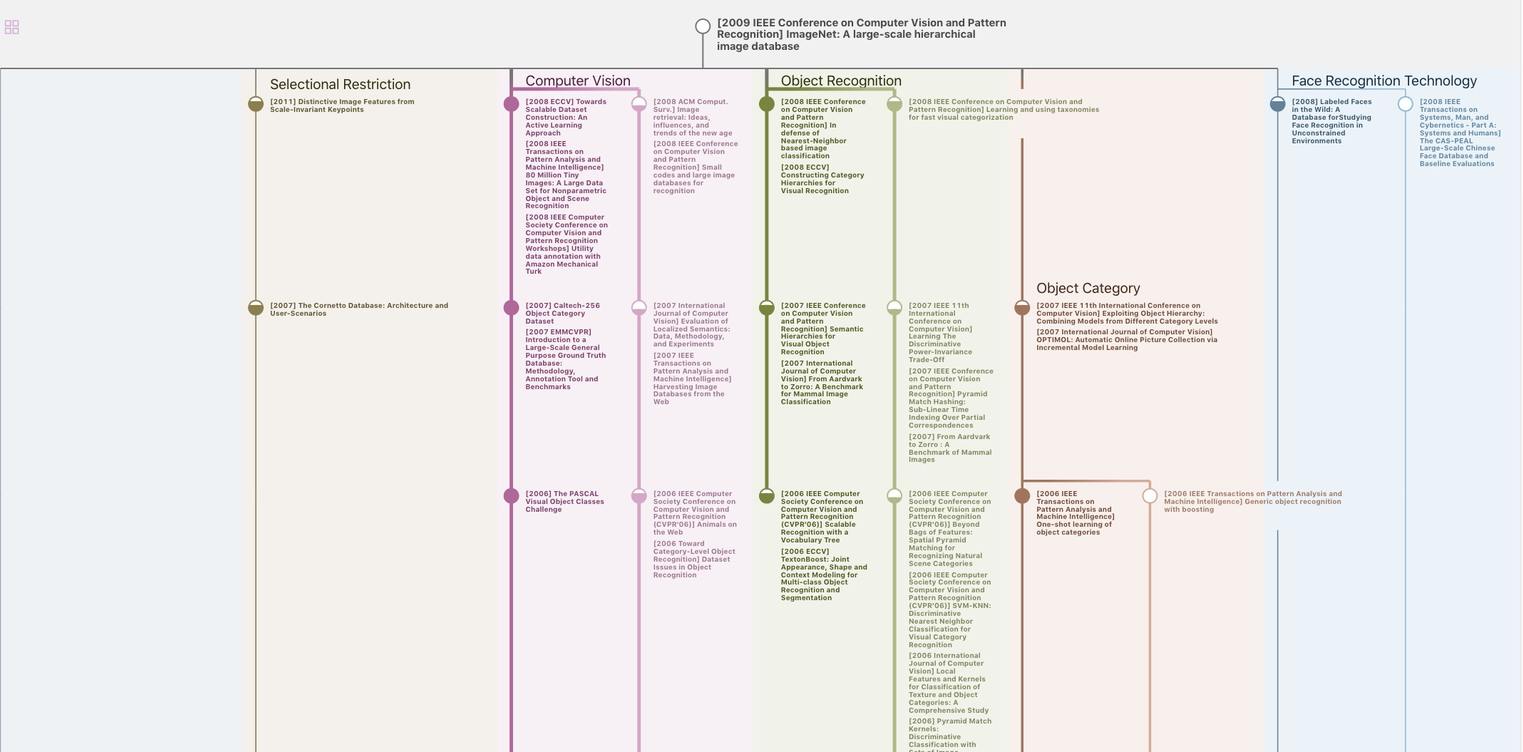
生成溯源树,研究论文发展脉络
Chat Paper
正在生成论文摘要