Online Learning in Supply-Chain Games
arxiv(2022)
摘要
We study a repeated game between a supplier and a retailer who want to maximize their respective profits without full knowledge of the problem parameters. After characterizing the uniqueness of the Stackelberg equilibrium of the stage game with complete information, we show that even with partial knowledge of the joint distribution of demand and production costs, natural learning dynamics guarantee convergence of the joint strategy profile of supplier and retailer to the Stackelberg equilibrium of the stage game. We also prove finite-time bounds on the supplier's regret and asymptotic bounds on the retailer's regret, where the specific rates depend on the type of knowledge preliminarily available to the players. In the special case when the supplier is not strategic (vertical integration), we prove optimal finite-time regret bounds on the retailer's regret (or, equivalently, the social welfare) when costs and demand are adversarially generated and the demand is censored.
更多查看译文
关键词
learning,supply-chain
AI 理解论文
溯源树
样例
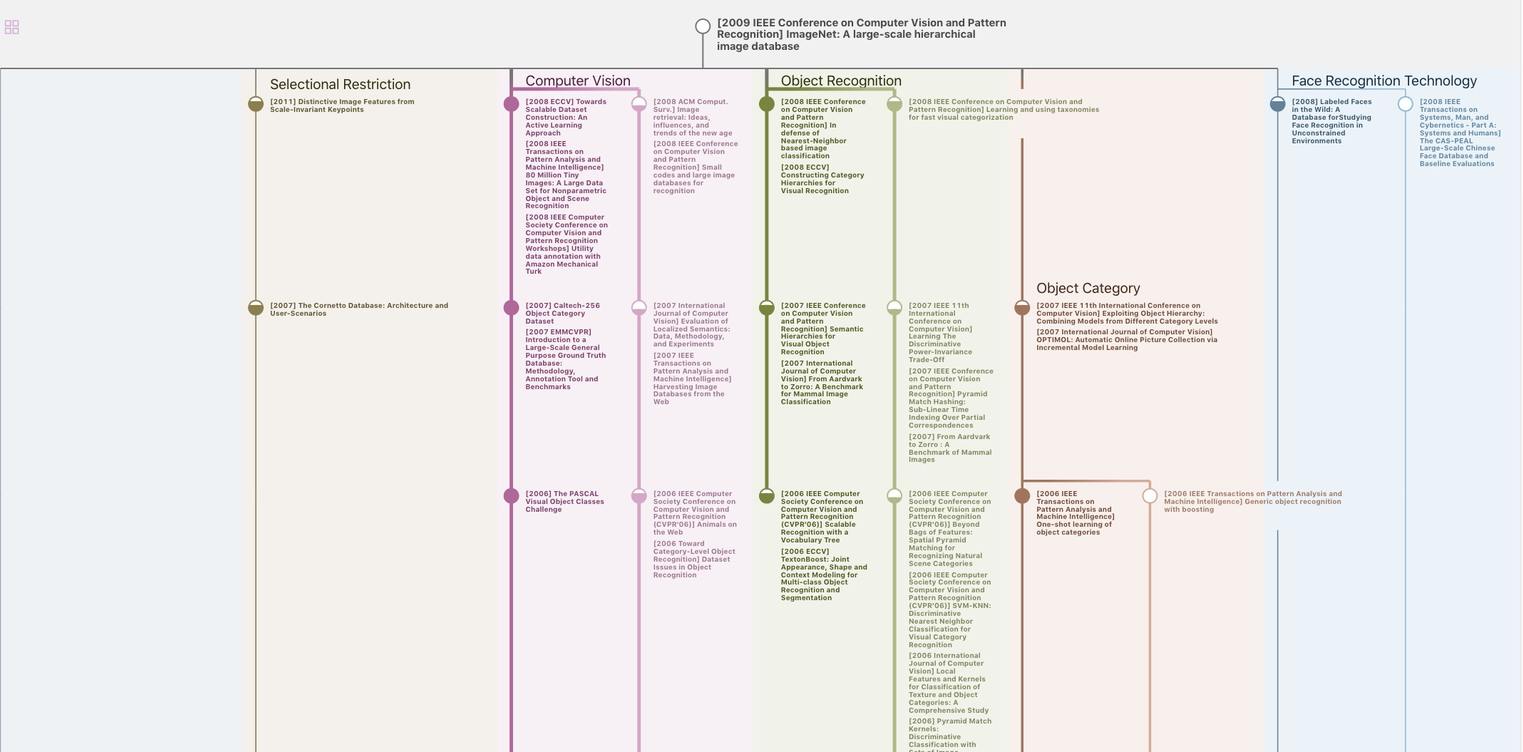
生成溯源树,研究论文发展脉络
Chat Paper
正在生成论文摘要