Federated Learning-Based Space-Time Data Prediction on Edge Devices
2022 7th International Conference on Intelligent Computing and Signal Processing (ICSP)(2022)
摘要
With the wide application of edge devices in Internet of Things (IoT), the current space-time data prediction algorithms are unable to accurately predict the frequently updated time series data due to the inability to automatically update the prediction models according to the different scenarios. In addition, degrading the prediction performance due to the collected data cannot be shared among edge devices with privacy protection. In order to solve above problem and achieve accurate prediction under privacy-preserving, the space-time convolution networks based on federated learning (FedSTCN) algorithm is proposed. Specifically, the space-time convolution networks (STCN) is adopted to extract temporal and spatial features for achieving high-precision multi-sensor monitoring data prediction. Moreover, the FedSTCN shares model training weights between edge devices under privacy-preserving to guarantee the distributed prediction performance. Numerical simulations show that the proposed FedSTCN algorithm achieves superior prediction performance than existing the-state-of-art space-time data prediction algorithms with fewer datasets and privacy protection.
更多查看译文
关键词
edge computing,federated learning,IoT,space-time data prediction
AI 理解论文
溯源树
样例
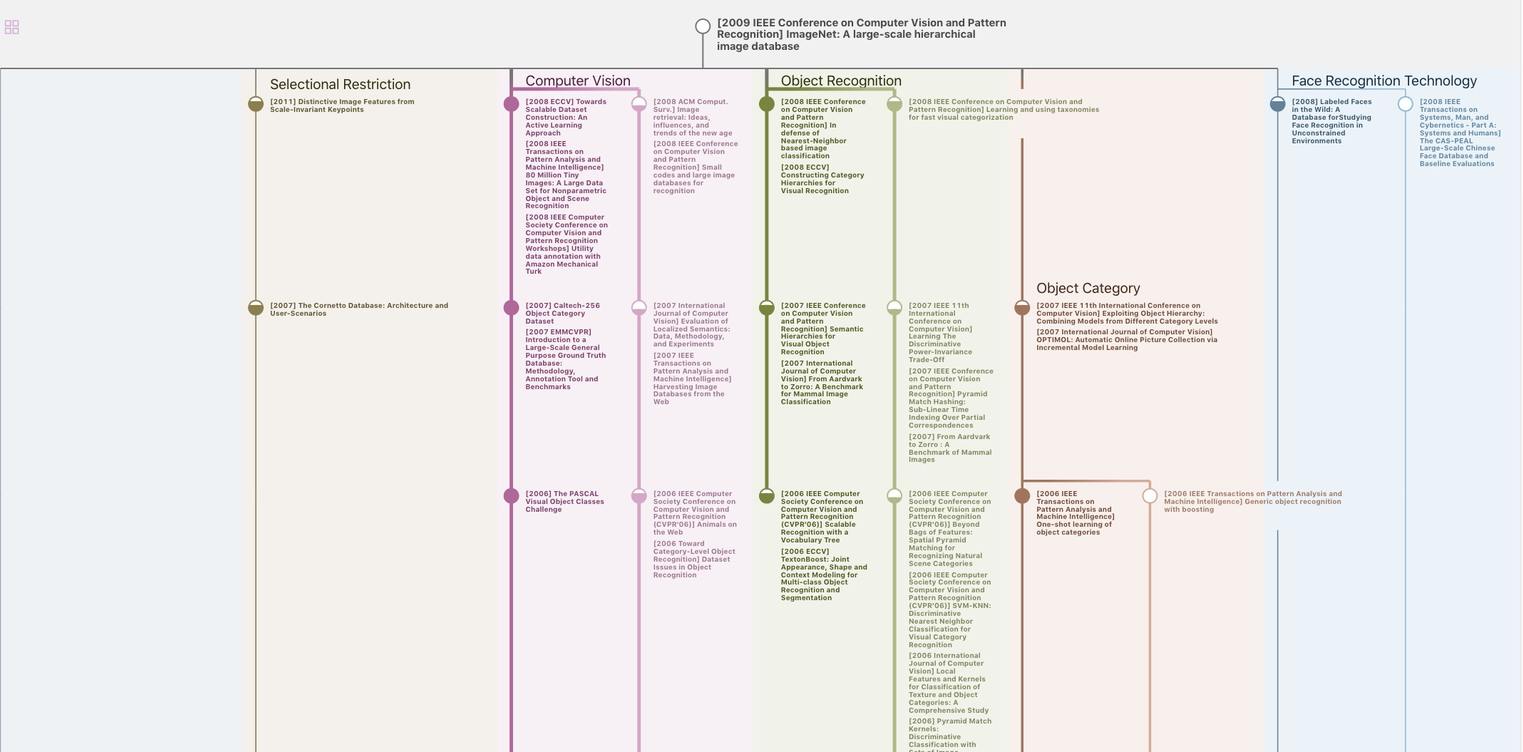
生成溯源树,研究论文发展脉络
Chat Paper
正在生成论文摘要