Transfer Learning-enabled Modelling Framework for Digital Twin
2022 IEEE 25th International Conference on Computer Supported Cooperative Work in Design (CSCWD)(2022)
摘要
Recently the machine learning-enabled modeling technology has become a powerful tool to develop data-driven models for explaining, predicting, and describing system behaviors. In particular, it has become a key tool for developing data-driven models for emerging digital twin development which demands the living models for simulating system behaviors. However, such data-driven models carry a fatal deficiency: once the operational environments changed, the model may hardly work well or even becomes useless. This paper attempts to address this issue by proposing to apply transfer learning techniques to develop lifetime robust models for real-world applications. After laying out problems and the reasons of model performance degradation, this paper presents a framework for developing lifetime predictive models for digital twin. A show case from our on-going research project along with the preliminary results demonstrates the feasibility and usefulness of the proposed predictive modeling methods.
更多查看译文
关键词
machine learning,predictive modeling,transfer learning,digital twin,model behavior transfer,data subspace transfer,distribution shift
AI 理解论文
溯源树
样例
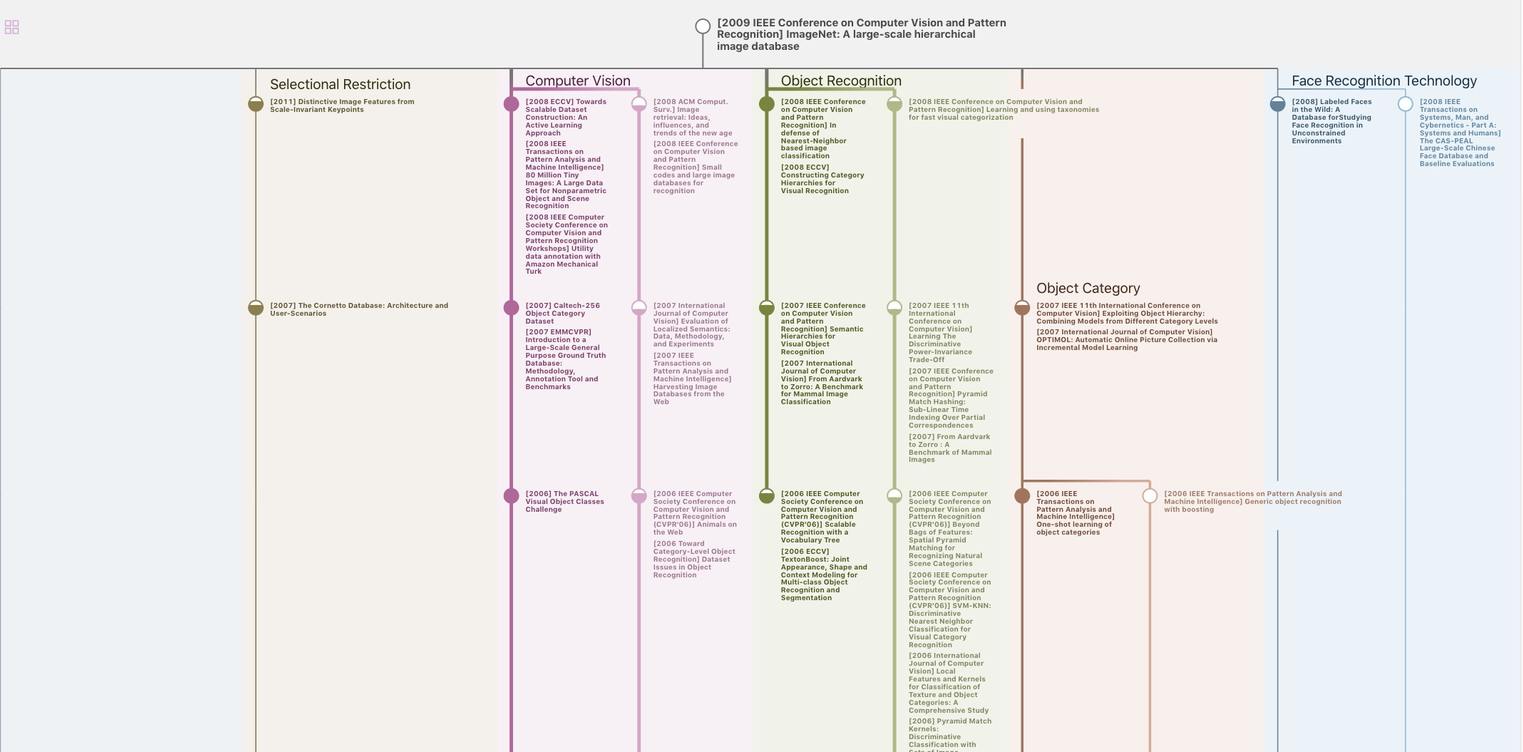
生成溯源树,研究论文发展脉络
Chat Paper
正在生成论文摘要