AUnet: A Deep Learning Framework for Surface Water Channel Mapping Using Large-Coverage Remote Sensing Images and Sparse Scribble Annotations from OSM Data
REMOTE SENSING(2022)
摘要
Water is a vital component of life that exists in a variety of forms, including oceans, rivers, ponds, streams, and canals. The automated methods for detecting, segmenting, and mapping surface water have improved significantly with the advancements in satellite imagery and remote sensing. Many strategies and techniques to segment water resources have been presented in the past. However, due to the variant width and complex appearance, the segmentation of the water channel remains challenging. Moreover, traditional supervised deep learning frameworks have been restricted by the scarcity of water channel datasets that include precise water annotations. With this in mind, this research presents the following three main contributions. Firstly, we curated a new dataset for water channel mapping in the Pakistani region. Instead of employing pixel-level water channel annotations, we used a weakly trained method to extract water channels from VHR pictures, relying only on OpenStreetMap (OSM) waterways to create sparse scribbling annotations. Secondly, we benchmarked the dataset on state-of-the-art semantic segmentation frameworks. We also proposed AUnet, an atrous convolution inspired deep learning network for precise water channel segmentation. The experimental results demonstrate the superior performance of the proposed AUnet model for segmenting using weakly supervised labels, where it achieved a mean intersection over union score of 0.8791 and outperformed state-of-the-art approaches by 5.90% for the extraction of water channels.
更多查看译文
关键词
deep learning,remote sensing,water channel extraction,segmentation,Landsat-8 satellite,Google Earth Engine
AI 理解论文
溯源树
样例
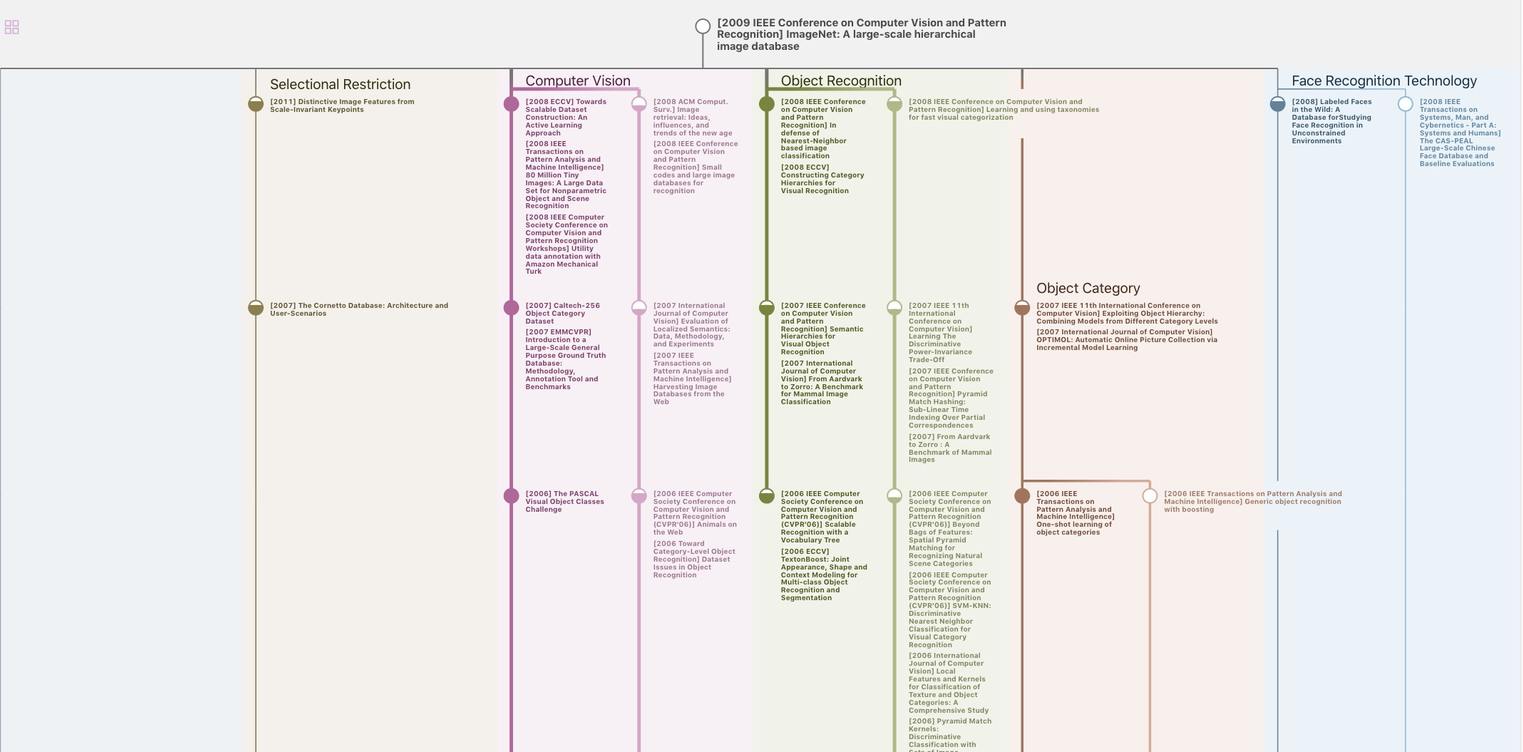
生成溯源树,研究论文发展脉络
Chat Paper
正在生成论文摘要