Big Learning: A Universal Machine Learning Paradigm?
ICLR 2023(2022)
摘要
Recent breakthroughs based on big/foundation models reveal a vague avenue for AI, that is, \emph{big data, big/foundation models, big learning, $\cdots$}. Following that avenue, here we elaborate on our newly introduced big learning. Specifically, big learning exhaustively exploits the information/tasks inherent in its large-scale \emph{complete/incomplete} training data, by learning to simultaneously model many/all joint/conditional/marginal data distributions (thus named big learning) with one universal foundation model. We reveal that big learning is what existing foundation models are implicitly doing; accordingly, our big learning provides high-level guidance for flexible design and improvements of foundation models. Besides, big learning ($i$) is equipped with great flexibilities for complete/incomplete training data and for customizing trustworthy data tasks; ($ii$) potentially delivers all joint/conditional/marginal data capabilities after training; ($iii$) significantly reduces the training-test gap with improved model generalization; and ($iv$) potentially unifies conventional machine learning paradigms and enables their flexible cooperations, manifested as a universal learning paradigm. Preliminary experiments verified the effectiveness of the presented big learning.
更多查看译文
关键词
Foundation models,big learning,incomplete data,GAN
AI 理解论文
溯源树
样例
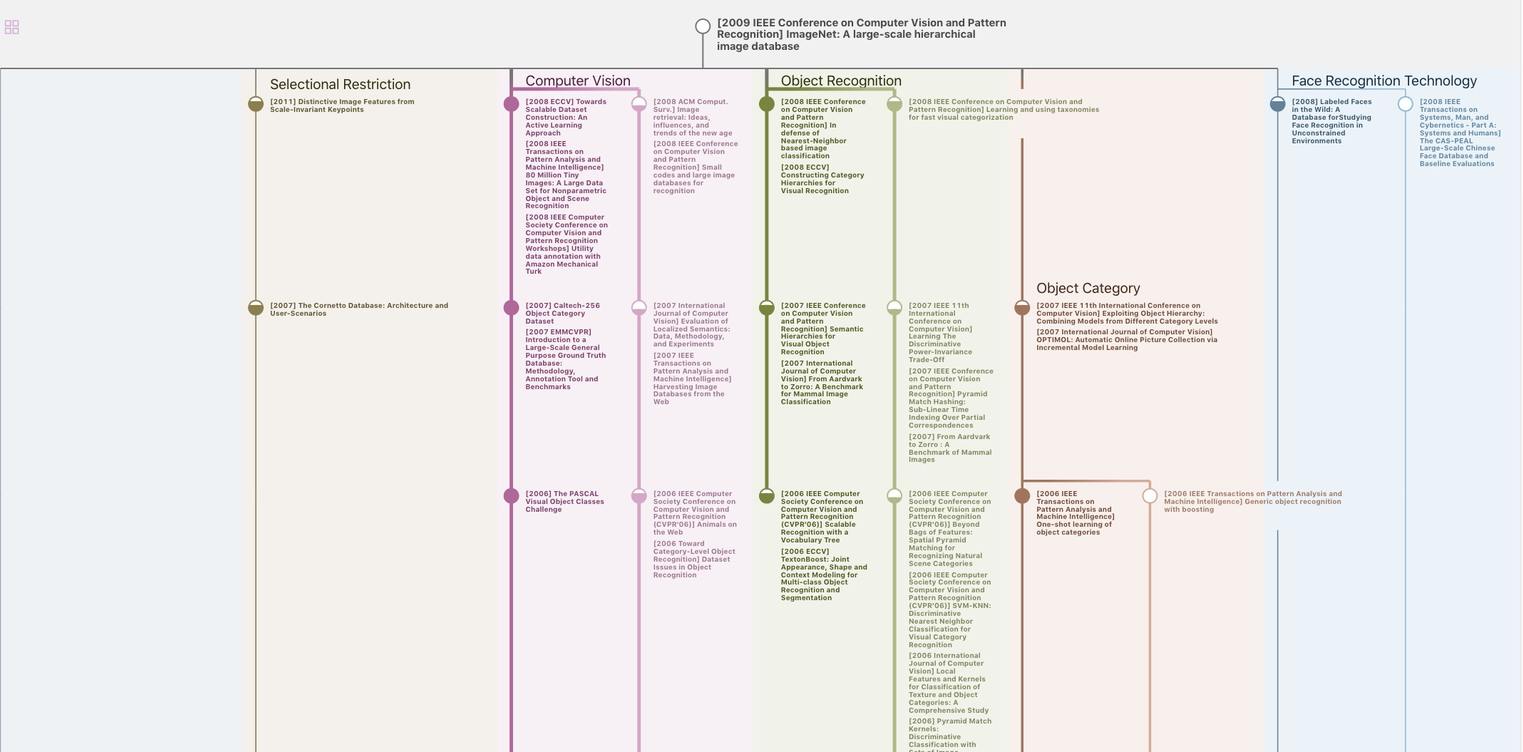
生成溯源树,研究论文发展脉络
Chat Paper
正在生成论文摘要