A Non-isotropic Probabilistic Take on Proxy-based Deep Metric Learning.
European Conference on Computer Vision(2022)
摘要
Proxy-based Deep Metric Learning (DML) learns deep representations by embedding images close to their class representatives (proxies), commonly with respect to the angle between them. However, this disregards the embedding norm, which can carry additional beneficial context such as class- or image-intrinsic uncertainty. In addition, proxy-based DML struggles to learn class-internal structures. To address both issues at once, we introduce non-isotropic probabilistic proxy-based DML. We model images as directional von Mises-Fisher (vMF) distributions on the hypersphere that can reflect image-intrinsic uncertainties. Further, we derive non-isotropic von Mises-Fisher (nivMF) distributions for class proxies to better represent complex class-specific variances. To measure the proxy-to-image distance between these models, we develop and investigate multiple distribution-to-point and distribution-to-distribution metrics. Each framework choice is motivated by a set of ablational studies, which showcase beneficial properties of our probabilistic approach to proxy-based DML, such as uncertainty-awareness, better behaved gradients during training, and overall improved generalization performance. The latter is especially reflected in the competitive performance on the standard DML benchmarks, where our approach compares favourably, suggesting that existing proxy-based DML can significantly benefit from a more probabilistic treatment. Code is available at http://github.com/ExplainableML/Probabilistic_Deep_Metric_Learning.
更多查看译文
关键词
Deep metric learning,von Mises-Fisher,Non-isotropy,Probablistic embeddings,Uncertainty
AI 理解论文
溯源树
样例
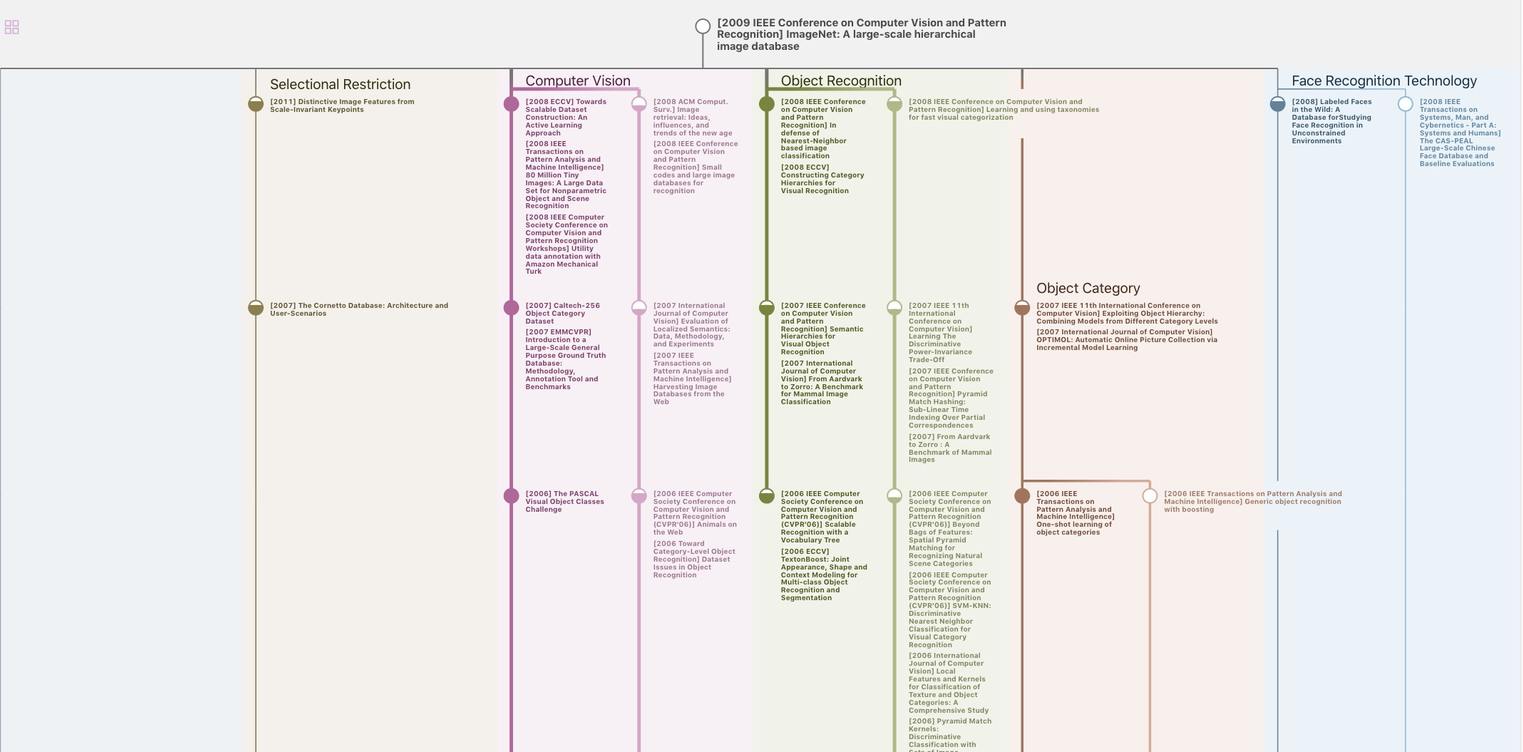
生成溯源树,研究论文发展脉络
Chat Paper
正在生成论文摘要