Automatic Synthesis of Neurons for Recurrent Neural Nets
arxiv(2022)
摘要
We present a new class of neurons, ARNs, which give a cross entropy on test data that is up to three times lower than the one achieved by carefully optimized LSTM neurons. The explanations for the huge improvements that often are achieved are elaborate skip connections through time, up to four internal memory states per neuron and a number of novel activation functions including small quadratic forms. The new neurons were generated using automatic programming and are formulated as pure functional programs that easily can be transformed. We present experimental results for eight datasets and found excellent improvements for seven of them, but LSTM remained the best for one dataset. The results are so promising that automatic programming to generate new neurons should become part of the standard operating procedure for any machine learning practitioner who works on time series data such as sensor signals.
更多查看译文
关键词
synthesis,neurons
AI 理解论文
溯源树
样例
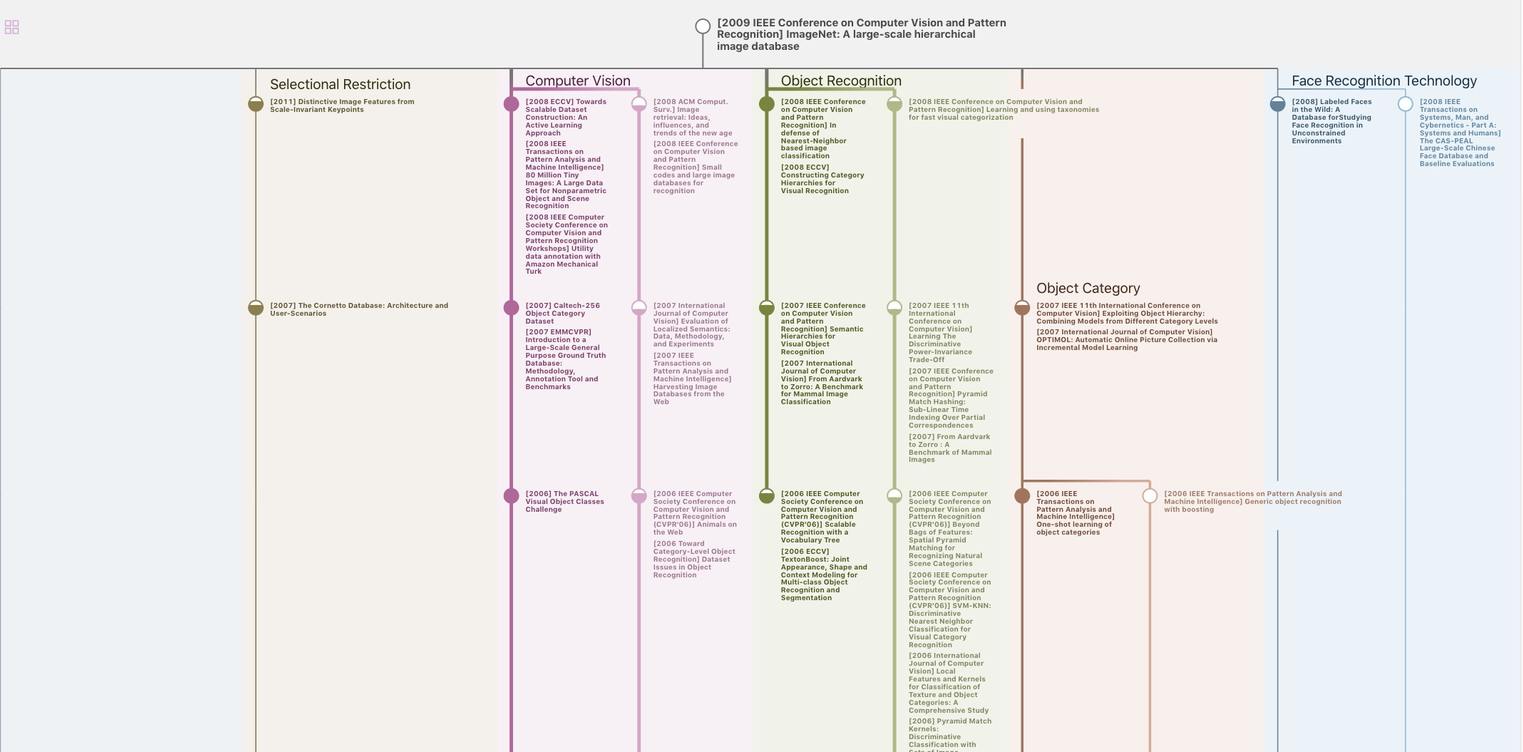
生成溯源树,研究论文发展脉络
Chat Paper
正在生成论文摘要