On the Interpretability of Machine Learning Regression for Path-Loss Prediction of Millimeter-Wave Links
SSRN Electronic Journal(2022)
摘要
Millimeter-wave communication systems design requires accurate path-loss (PL) prediction, which is critical to determining coverage area and system capacity. In this work, four machine learning (ML) algorithms are proposed for PL prediction in an indoor environment for 5G millimeter-wave frequencies, from 26.5 to 40 GHz. They are artificial neural network (ANN), support vector regression (SVR), random forest (RF), and gradient tree boosting (GTB). We compare their performances, including also the empirical PL models ABG and CIF incorporating the number of crossed walls, as we propose. For the ML models, we present a methodology to select the predictor coalition by examining the marginal performance and the interpretability gains. The methodology allows choosing the most appropriate predictor coalition for building machines while not overlooking the non-linear connections between predictors and the path-loss. The results show that the ML techniques improve the prediction accuracy of empirical models. Among the ML algorithms employed, considering the optimal subset of predictors, the GTB model presents the best prediction and generalization capacities.
更多查看译文
关键词
Path-loss,Machine learning,Model selection,Ensemble methods
AI 理解论文
溯源树
样例
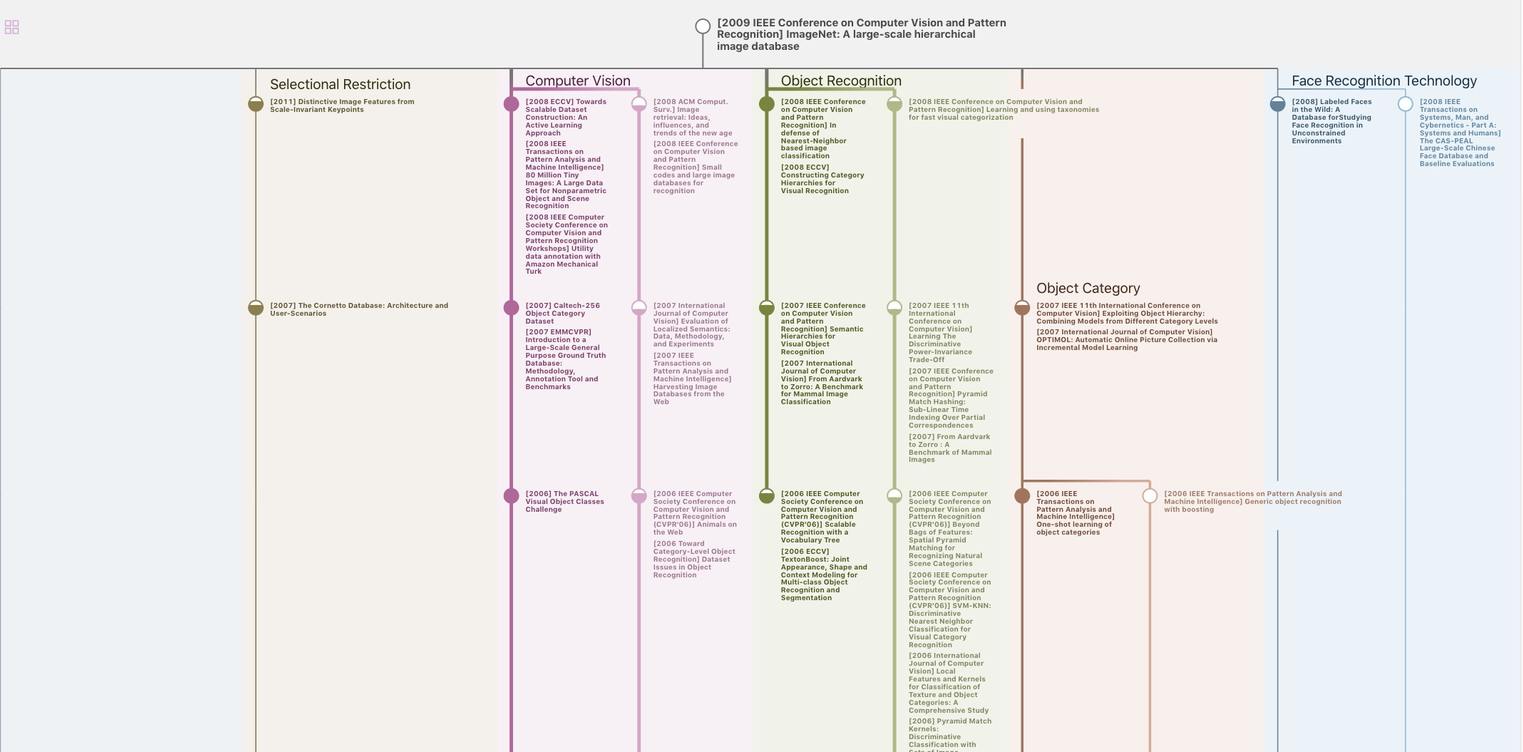
生成溯源树,研究论文发展脉络
Chat Paper
正在生成论文摘要