Decision Trees for Glaucoma Screening Based on the Asymmetry of the Retinal Nerve Fiber Layer in Optical Coherence Tomography
SENSORS(2022)
摘要
Purpose: The aim of this study was to analyze the relevance of asymmetry features between both eyes of the same patient for glaucoma screening using optical coherence tomography. Methods: Spectral-domain optical coherence tomography was used to estimate the thickness of the peripapillary retinal nerve fiber layer in both eyes of the patients in the study. These measurements were collected in a dataset from healthy and glaucoma patients. Several metrics for asymmetry in the retinal nerve fiber layer thickness between the two eyes were then proposed. These metrics were evaluated using the dataset by performing a statistical analysis to assess their significance as relevant features in the diagnosis of glaucoma. Finally, the usefulness of these asymmetry features was demonstrated by designing supervised machine learning models that can be used for the early diagnosis of glaucoma. Results: Machine learning models were designed and optimized, specifically decision trees, based on the values of proposed asymmetry metrics. The use of these models on the dataset provided good classification of the patients (accuracy 88%, sensitivity 70%, specificity 93% and precision 75%). Conclusions: The obtained machine learning models based on retinal nerve fiber layer asymmetry are simple but effective methods which offer a good trade-off in classification of patients and simplicity. The fast binary classification relies on a few asymmetry values of the retinal nerve fiber layer thickness, allowing their use in the daily clinical practice for glaucoma screening.
更多查看译文
关键词
optical coherence tomography (OCT), peripapillary OCT, retinal nerve fiber layer (RNFL), RNFL thickness asymmetry, retinal imaging analysis, glaucoma, decision trees
AI 理解论文
溯源树
样例
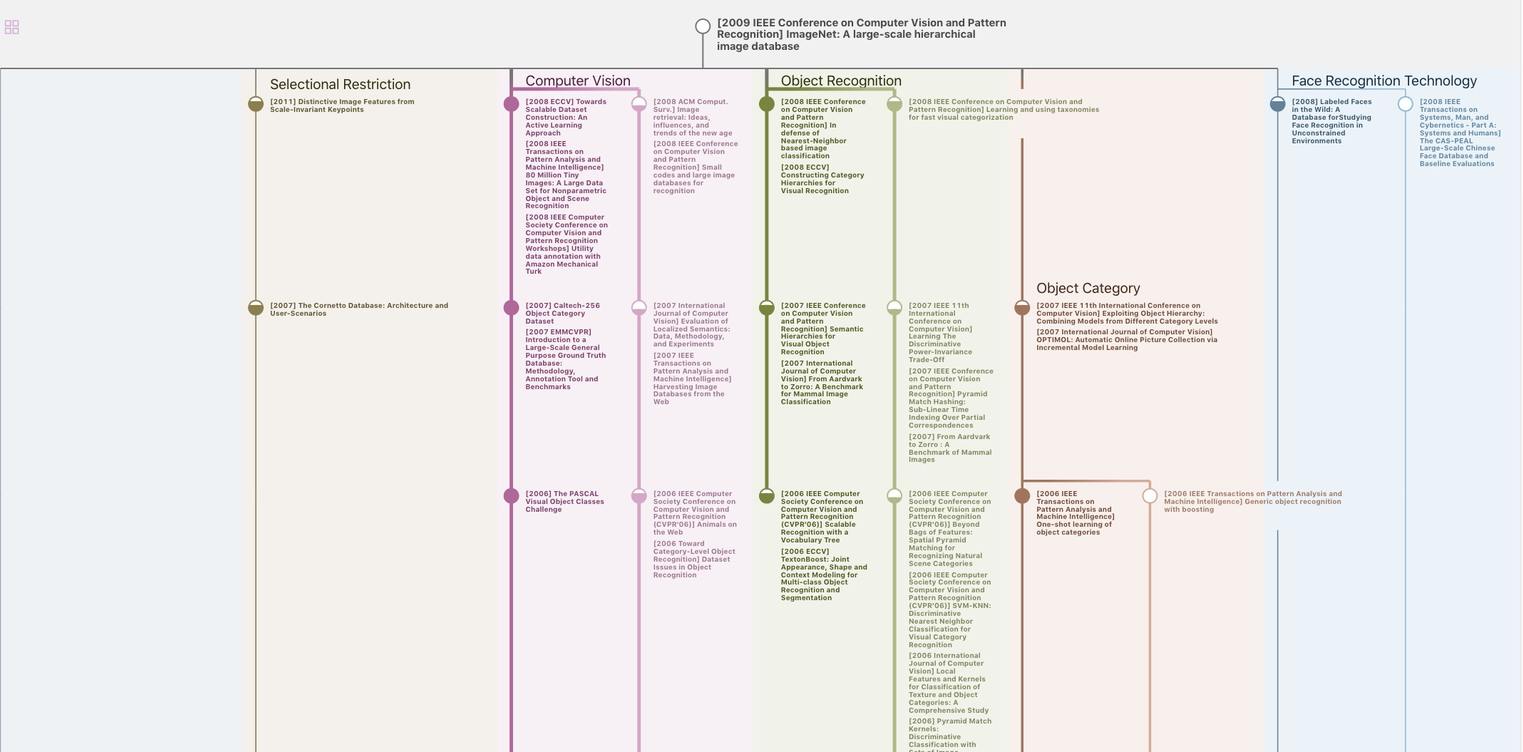
生成溯源树,研究论文发展脉络
Chat Paper
正在生成论文摘要