A comparison of spectral clustering and the walktrap algorithm for community detection in network psychometrics.
Psychological methods(2022)
摘要
Spectral clustering is a well-known method for clustering the vertices of an undirected network. Although its use in network psychometrics has been limited, spectral clustering has a close relationship to the commonly used walktrap algorithm. In this article, we report results from simulation experiments designed to evaluate the ability of spectral clustering and the walktrap algorithm to recover underlying cluster (or community) structure in networks. The salient findings include: (a) the recovery performance of the walktrap algorithm can be improved by using K-means clustering instead of hierarchical clustering; (b) -means and -median clustering led to comparable recovery performance when used to cluster vertices based on the eigenvectors of Laplacian matrices in spectral clustering; (c) spectral clustering using the unnormalized Laplacian matrix generally yielded inferior cluster recovery in comparison to the other methods; (d) when the correct number of clusters was provided for the methods, spectral clustering using the normalized Laplacian matrix led to better recovery than the walktrap algorithm; and (e) when the correct number of clusters was not provided, the walktrap algorithm using the modularity index was better than spectral clustering using the eigengap heuristic at determining the appropriate number of clusters. Overall, both the walktrap algorithm and spectral clustering of the normalized Laplacian matrix are effective for partitioning the vertices of undirected networks, with the latter performing better in most instances. (PsycInfo Database Record (c) 2022 APA, all rights reserved).
更多查看译文
关键词
network psychometrics,spectral clustering,walktrap algorithm,K-means clustering,K-median clustering
AI 理解论文
溯源树
样例
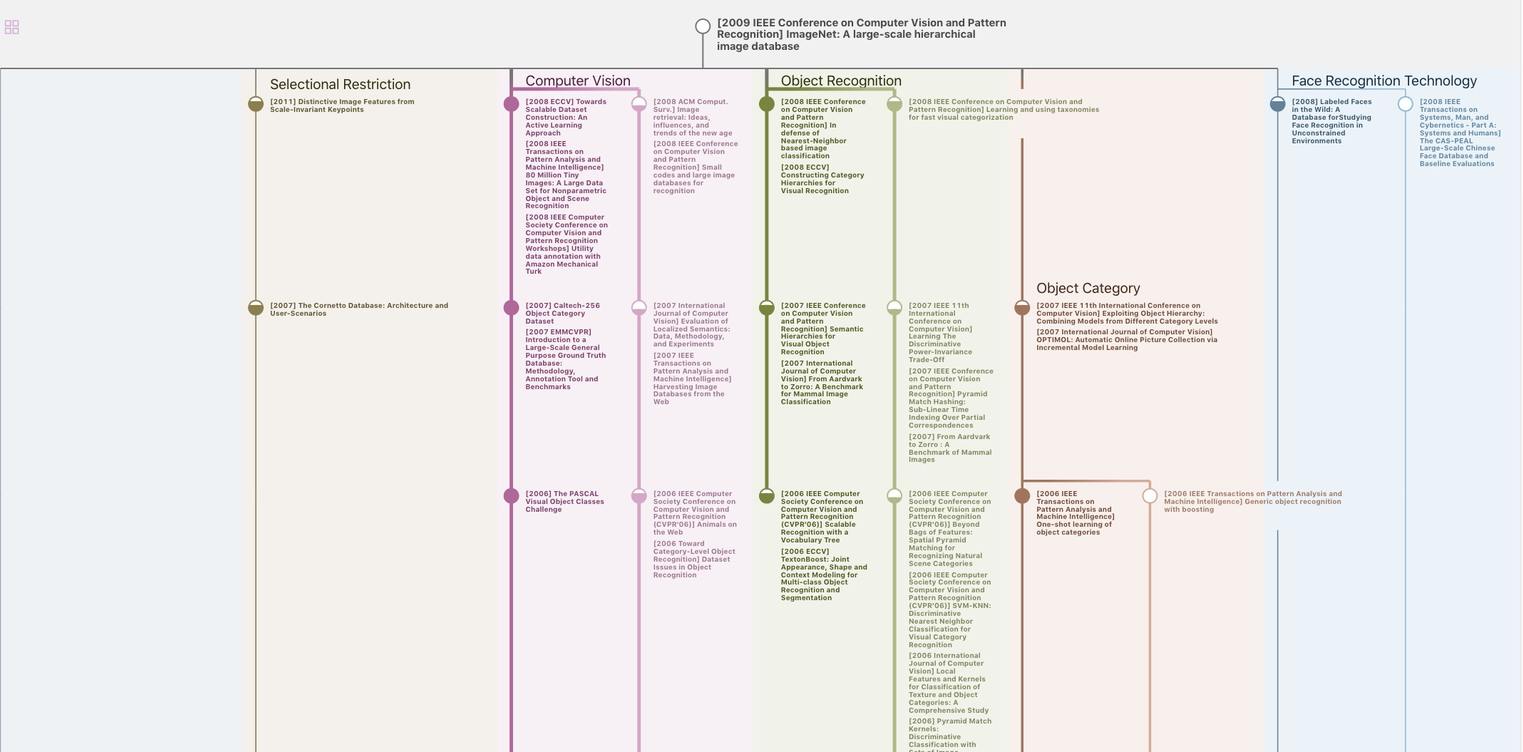
生成溯源树,研究论文发展脉络
Chat Paper
正在生成论文摘要