Egocentric Visual Self-Modeling for Autonomous Robot Dynamics Prediction and Adaptation
arxiv(2022)
摘要
The ability of robots to model their own dynamics is key to autonomous
planning and learning, as well as for autonomous damage detection and recovery.
Traditionally, dynamic models are pre-programmed or learned from external
observations. Here, we demonstrate for the first time how a task-agnostic
dynamic self-model can be learned using only a single first-person-view camera
in a self-supervised manner, without any prior knowledge of robot morphology,
kinematics, or task. Through experiments on a 12-DoF robot, we demonstrate the
capabilities of the model in basic locomotion tasks using visual input.
Notably, the robot can autonomously detect anomalies, such as damaged
components, and adapt its behavior, showcasing resilience in dynamic
environments. Furthermore, the model's generalizability was validated across
robots with different configurations, emphasizing its potential as a universal
tool for diverse robotic systems. The egocentric visual self-model proposed in
our work paves the way for more autonomous, adaptable, and resilient robotic
systems.
更多查看译文
AI 理解论文
溯源树
样例
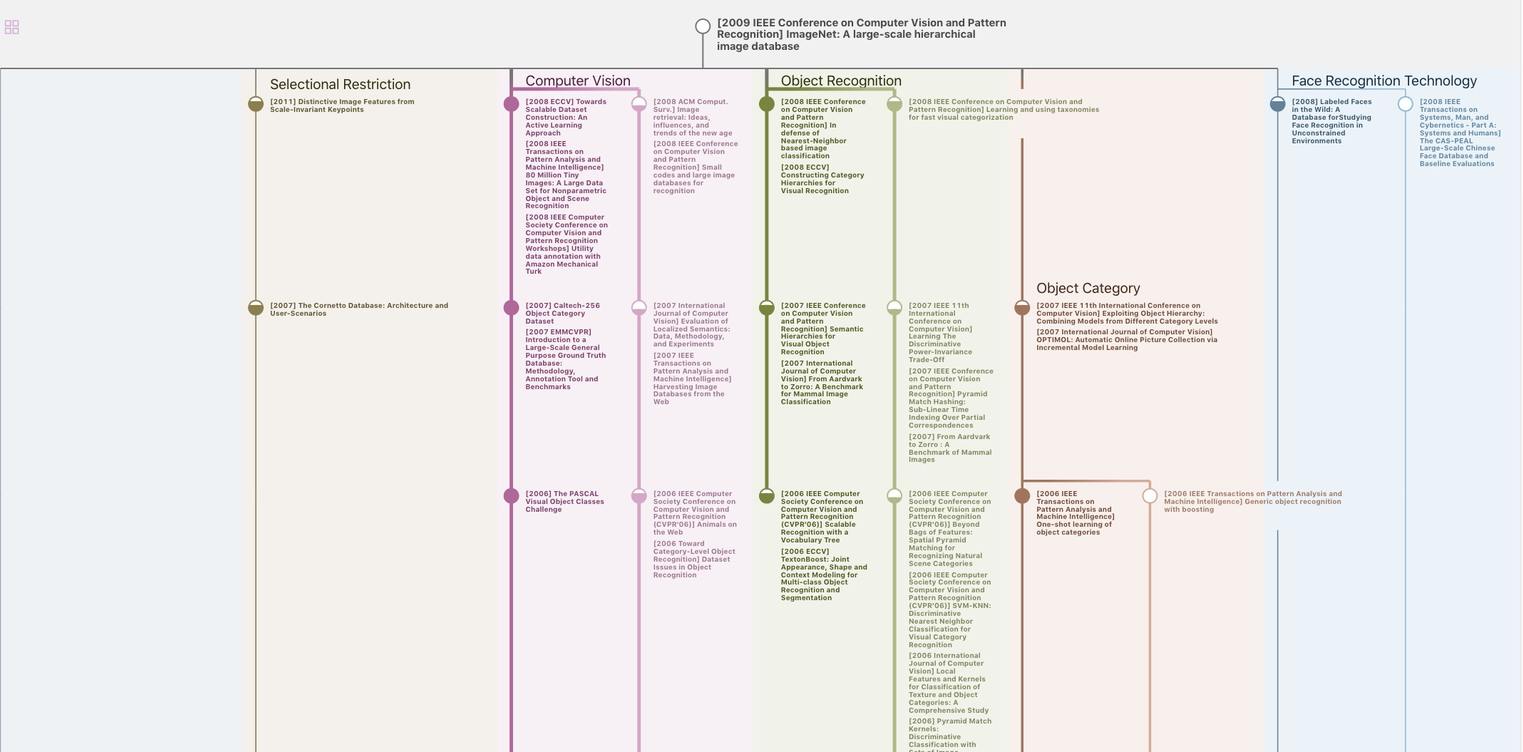
生成溯源树,研究论文发展脉络
Chat Paper
正在生成论文摘要