Learning the stress-strain fields in digital composites using Fourier neural operator
iScience(2022)
摘要
Increased demands for high-performance materials have led to advanced composite materials with complex hierarchical designs. However, designing a tailored material microstructure with targeted properties and performance is extremely challenging due to the innumerable design combinations and prohibitive computational costs for physics-based solvers. In this study, we employ a neural operator-based framework, namely Fourier neural operator (FNO), to learn the mechanical response of 2D composites. We show that the FNO exhibits high-fidelity predictions of the complete stress and strain tensor fields for geometrically complex composite microstructures with very few training data and purely based on the microstructure. The model also exhibits zero-shot generalization on unseen arbitrary geometries with high accuracy. Furthermore, the model exhibits zero-shot super-resolution capabilities by predicting high-resolution stress and strain fields directly from low-resolution input configurations. Finally, the model also provides high-accuracy predictions of equivalent measures for stress-strain fields, allowing realistic upscaling of the results.
更多查看译文
关键词
Artificial intelligence,Mechanical property,Computational materials science
AI 理解论文
溯源树
样例
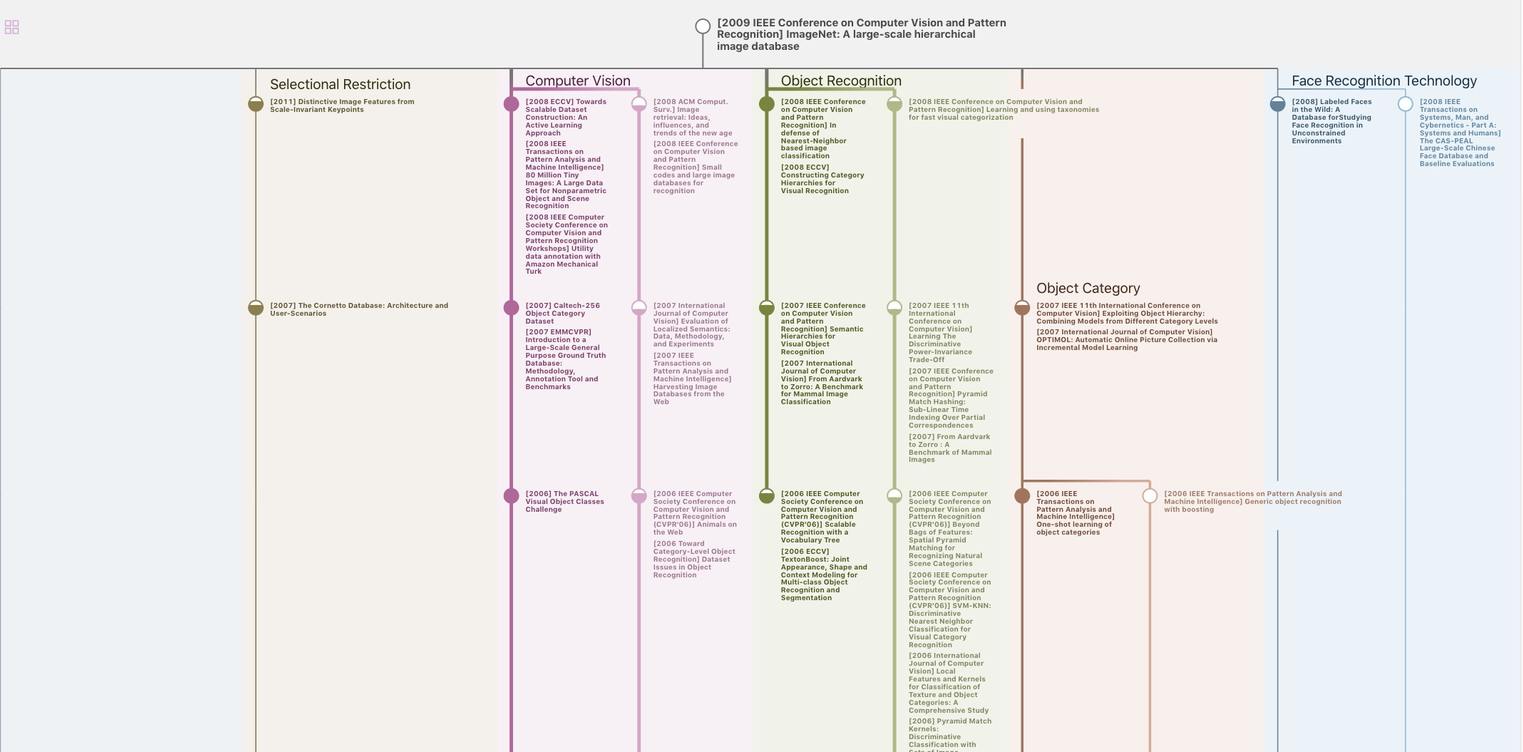
生成溯源树,研究论文发展脉络
Chat Paper
正在生成论文摘要