Uncertainty-aware self-supervised neural network for liver T (1? ) mapping with relaxation constraint
CoRR(2022)
摘要
Objective. T-1p mapping is a promising quantitative MRI technique for the non-invasive assessment of tissue properties. Learning-based approaches can map T-1p from a reduced number of T-1p weighted images but requires significant amounts of high-quality training data. Moreover, existing methods do not provide the confidence level of the T-1p estimation. We aim to develop a learning-based liver T-1p mapping approach that can map T-1p with a reduced number of images and provide uncertainty estimation. Approach. We proposed a self-supervised neural network that learns a T-1p mapping using the relaxation constraint in the learning process. Epistemic uncertainty and aleatoric uncertainty are modelled for the T1p quantification network to provide a Bayesian confidence estimation of the T-1p mapping. The uncertainty estimation can also regularize the model to prevent it from learning imperfect data. Main results. We conducted experiments on T-1p data collected from 52 patients with non-alcoholic fatty liver disease. The results showed that when only collecting two T-1p-weighted images, our method outperformed the existing methods for T-1p quantification of the liver. Our uncertainty estimation can further regularize the model to improve the performance of the model and it is consistent with the confidence level of liver T-1p values. Significance. Our method demonstrates the potential for accelerating the T-1p mapping of the liver by using a reduced number of images. It simultaneously provides uncertainty of T-1p quantification which is desirable in clinical applications.
更多查看译文
关键词
quantitative MRI,self-supervised learning,uncertainty estimation,T-1 rho
AI 理解论文
溯源树
样例
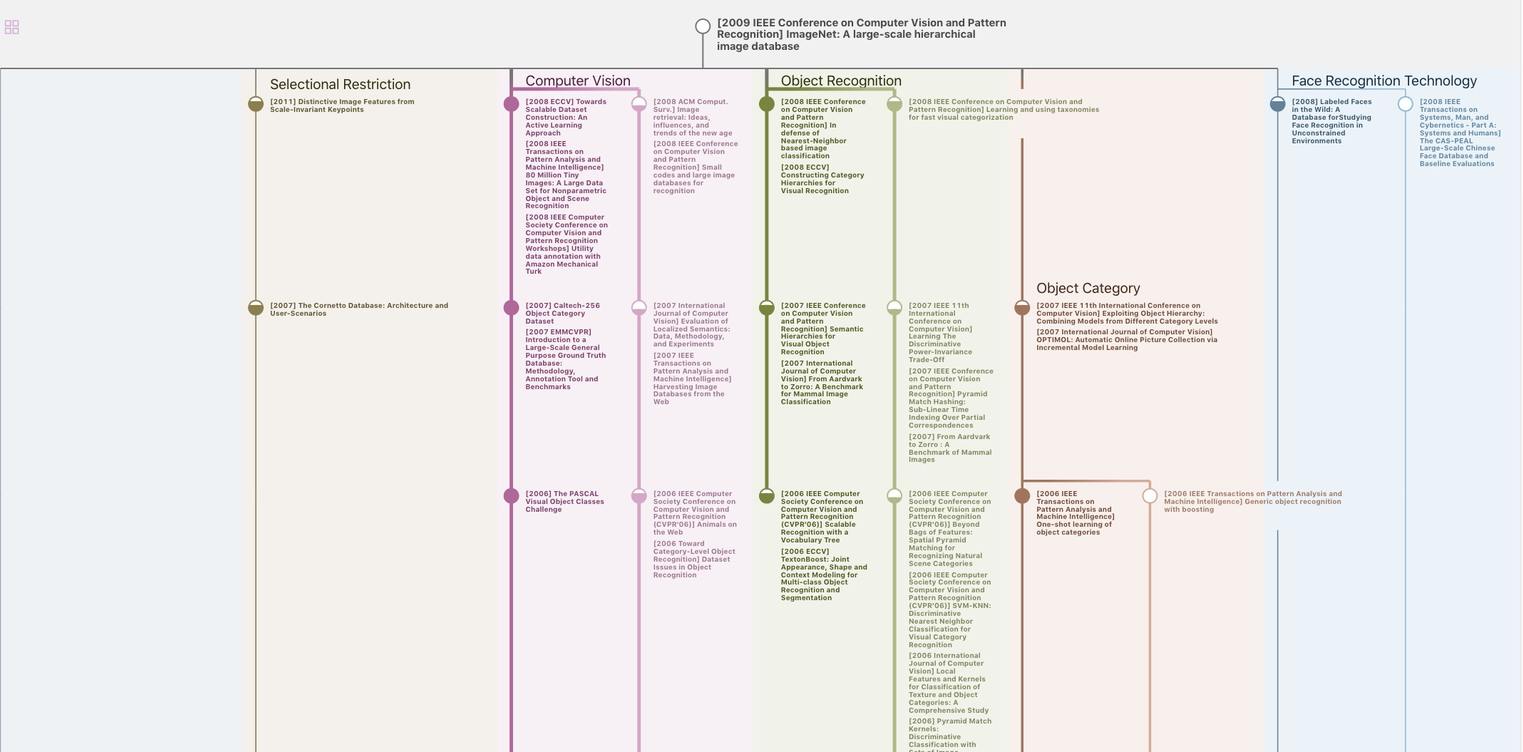
生成溯源树,研究论文发展脉络
Chat Paper
正在生成论文摘要