Integral correction of initial and model errors in system of multigrid NLS-4DVar data assimilation for numerical weather prediction (SNAP)
Quarterly Journal of the Royal Meteorological Society(2022)
摘要
Model error has a marked influence on forecast error, and integral correction of the initial and model errors in a novel four-dimensional variational data assimilation (4DVar) method (i4DVar) has recently been developed. Unlike traditional 4DVar, in which the analysis increment focuses only on the initial time point, i4DVar introduces an averaged integral correction term to correct the error evolution at multiple times with the same time interval in the assimilation window; that is, the assimilation window is divided into several sub-windows. In this study, we incorporated i4DVar into the System of Multigrid Nonlinear Least-squares-4DVar Data Assimilation for Numerical Weather Prediction (NWP) (SNAP), hereafter referred to as SN-i4DVar, and explored its impacts on NWP. The effectiveness and performance of SN-i4DVar were evaluated using two extreme rainstorm cases assimilating conventional and satellite observations, respectively. The results indicated that integral correction of the initial and model errors reduced analysis error and forecast error growth rates. SN-i4DVar performed better in precipitation forecasting than the original SNAP and exhibited generally better forecasting capability in terms of the horizontal wind speed (u and v), temperature, and humidity variables, indicating that SN-i4DVar simultaneously countered initial model errors to improve NWP capability. Meanwhile, the 4DEnVar method, which considers the optimal analysis in the ensemble space, benefits from the improved ensemble space generated according to the formulas of i4DVar, and the observation information can be more effectively absorbed and a more accurate analysis can be obtained by SN-i4DVar.
更多查看译文
关键词
model error, multigrid data assimilation, numerical weather prediction, precipitation
AI 理解论文
溯源树
样例
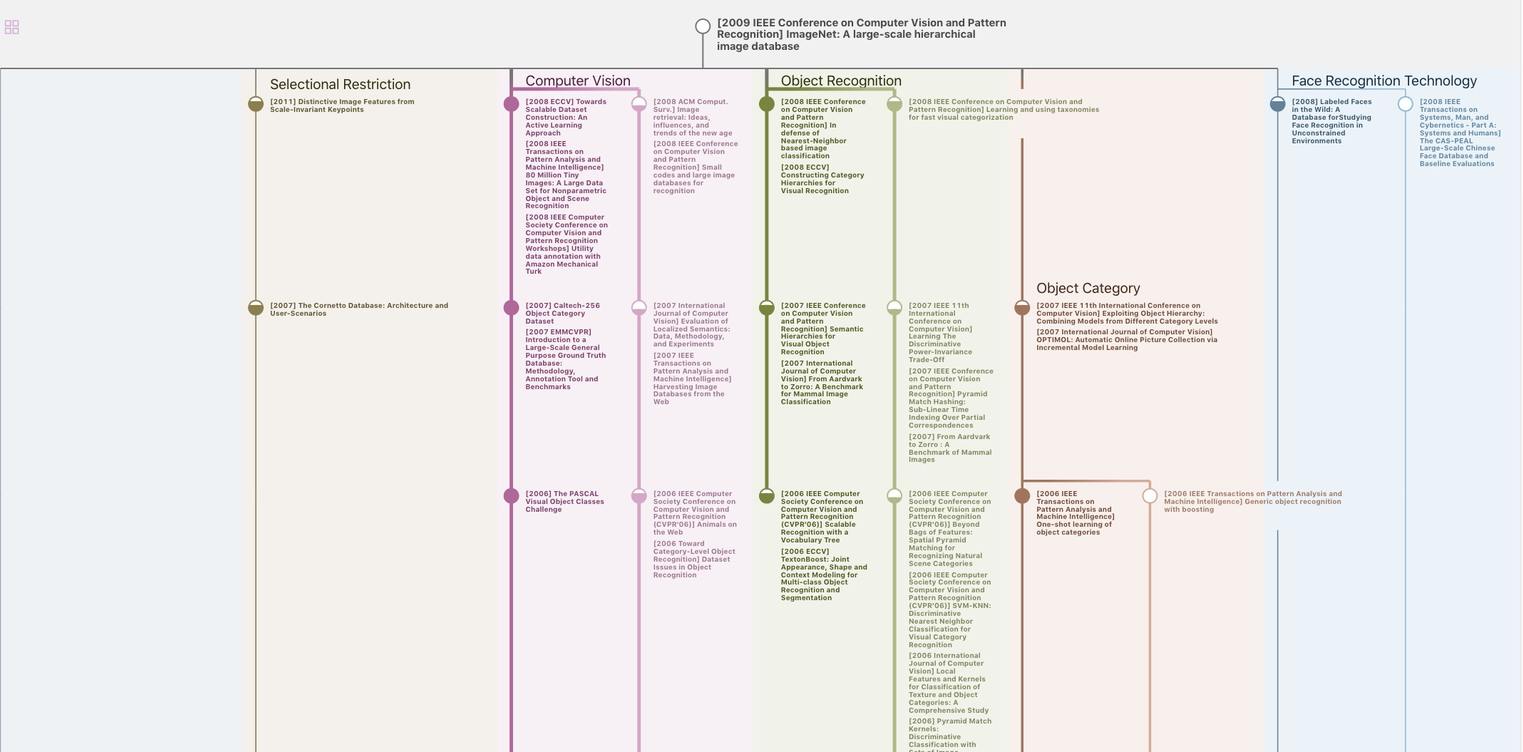
生成溯源树,研究论文发展脉络
Chat Paper
正在生成论文摘要