Rolling Bearing Fault Diagnosis Method Base on Periodic Sparse Attention and LSTM
IEEE Sensors Journal(2022)
摘要
The rolling bearing fault signals are complex time series with complex dynamic characteristics and non-uniform periodicity due to the influence of random interference, such as random impulse noise and equipment vibration. This will affect the accuracy of the diagnostis method. This paper analyses the characteristics of bearing fault signals and discussed the basic ideas of current diagnosis methods. In order to decrease the computational complexity in time series analysis process and reduce the influence of random interference in subsequent feature extraction, this paper proposes a rolling bearing fault diagnosis method base on periodic sparse attention and LSTM (PSAL) for non-uniform bearing vibration signals. According to the periodic characteristics of bearing fault, a periodic sparse attention network is proposed to decrease the time consumption in the process of reducing the influence of random interference and enhancing the feature. Then, LSTM is used to extract long-term dependence features in the fault signals. Finally, two sets of rolling bearing datasets are adopted to verify the validity and superiority of the proposed method by comparing with other methods.
更多查看译文
关键词
Attention mechanism,LSTM,intermittent faults diagnosis,rolling bearing
AI 理解论文
溯源树
样例
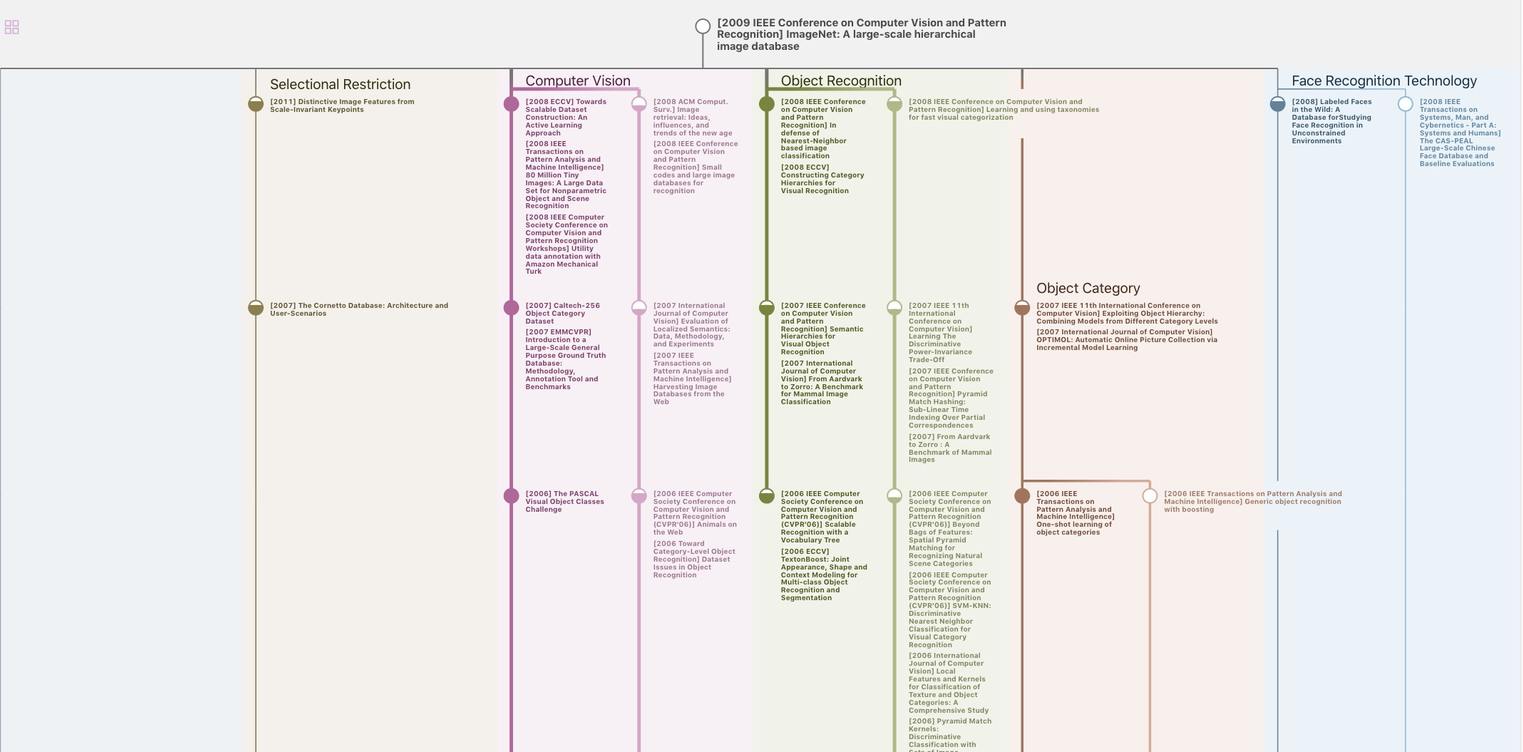
生成溯源树,研究论文发展脉络
Chat Paper
正在生成论文摘要