Towards Robotic Knee Arthroscopy: Spatial and Spectral Learning Model for Surgical Scene Segmentation
Algorithms for Intelligent SystemsProceedings of International Joint Conference on Advances in Computational Intelligence(2022)
摘要
Minimally invasive surgeries are complex to perform, and surgical outcomes are varied due to a limited view of the surgical scene. There is a lack of reliable vision systems that can identify and segment different tissue types intra-operatively. Here we introduce a novel approach towards overcoming this limitation. Our approach extracts geometric and spectral information captured by a miniaturized camera using deep learning algorithms. We have successfully implemented a deep neural network and trained it for in-situ labelling of soft and hard tissues acquired from clinically relevant cadaveric studies. Although presented and validated for knee arthroscopy our approach can be implemented across different endoscopic platforms. The intraoperative nature of tissue segmentation could be easily implemented in medical imaging systems for achieving better outcomes in minimally invasive procedures. In addition to segmenting different tissue types like, ACL, femur, tibia, cartilage, and meniscus, our network can also segment surgical tools present inside the knee cavity. The achieved dice similarity score for the tissue types femur, tibia, ACL, and meniscus were 0.91, 0.71, 0.39, and 0.62.
更多查看译文
关键词
robotic knee arthroscopy,spectral learning model,segmentation
AI 理解论文
溯源树
样例
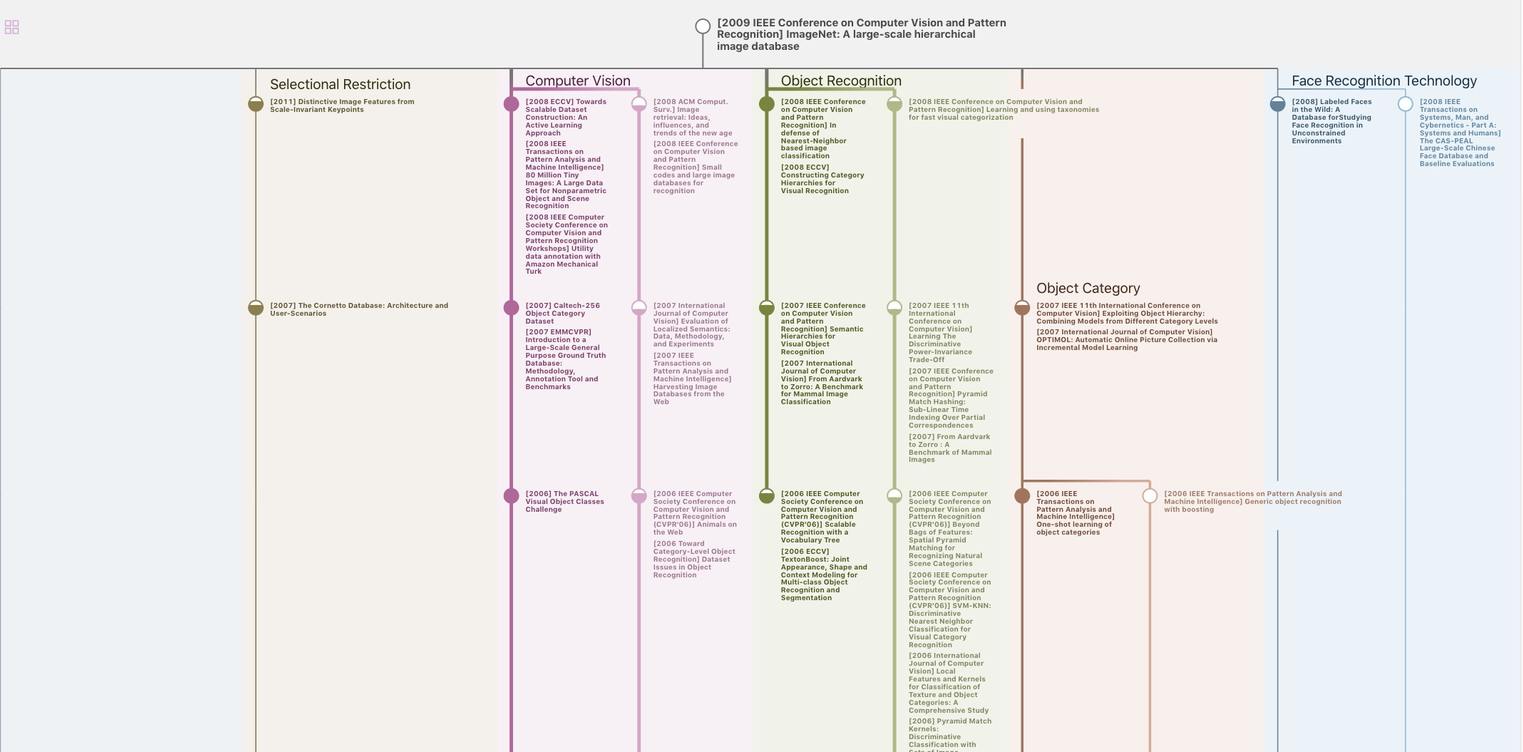
生成溯源树,研究论文发展脉络
Chat Paper
正在生成论文摘要