Recent Developments in Machine Learning Methods for Stochastic Control and Games
SSRN Electronic Journal(2023)
摘要
Stochastic optimal control and games have a wide range of applications, from
finance and economics to social sciences, robotics, and energy management. Many
real-world applications involve complex models that have driven the development
of sophisticated numerical methods. Recently, computational methods based on
machine learning have been developed for solving stochastic control problems
and games. In this review, we focus on deep learning methods that have unlocked
the possibility of solving such problems, even in high dimensions or when the
structure is very complex, beyond what traditional numerical methods can
achieve. We consider mostly the continuous time and continuous space setting.
Many of the new approaches build on recent neural-network-based methods for
solving high-dimensional partial differential equations or backward stochastic
differential equations, or on model-free reinforcement learning for Markov
decision processes that have led to breakthrough results. This paper provides
an introduction to these methods and summarizes the state-of-the-art works at
the crossroad of machine learning and stochastic control and games.
更多查看译文
关键词
stochastic control
AI 理解论文
溯源树
样例
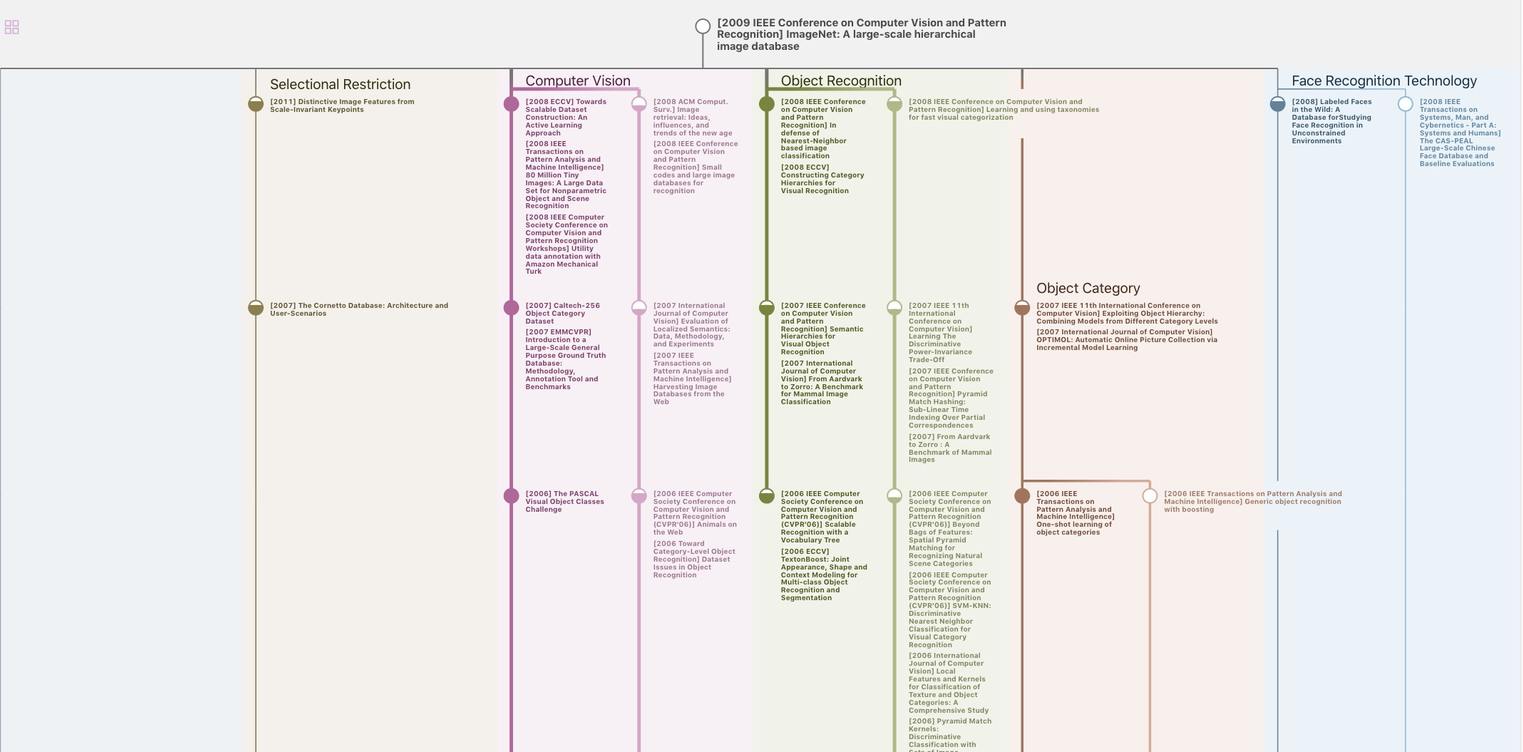
生成溯源树,研究论文发展脉络
Chat Paper
正在生成论文摘要