Estimation of PM2.5 Concentration Based on Support Vector Regression With Improved Dark Channel Prior and High Frequency Information in Images
IEEE Access(2022)
摘要
The rapid development of the petrochemical industry has caused great harm to the environment. Fine suspended particles with a diameter of less than 2.5 microns cause serious health problems when inhaled air of high concentration of PM2.5. Therefore, an estimate of the concentration of PM2.5 is sought. However, it generally requires expensive instruments installed in an air quality monitoring station and a professional to operate them. In addition to the expensive cost, the instruments require a high maintenance fee and are restricted by geographical location. To eliminate the difficulty, this paper presents a low-cost and effective approach to estimate the concentration of PM2.5 using image processing schemes. The proposed approach consists of four stages. First, images with different concentrations of PM2.5 were taken and the related relative humidity (RH) was collected. Second, an automatically selected region of interest (RoI) was used to extract two features from an image, namely high-frequency information and transmittance by an improved dark channel. Third, the two extracted features, together with the RH measurement, were used to build a support vector regression (SVR) model. Fourth, the SVR model was applied to estimate the concentration of PM2.5, whose performance was then evaluated and compared with four simple regression models and a modified reported SVR method. In the given data set, the proposed method outperforms the comparison methods in terms of R-2 and root mean squared error. The best performance of our method reaches R-2 =0.816 which is generally satisfactory in related applications.
更多查看译文
关键词
Air quality monitoring,automatic region of interest selection,high frequency information extraction,improved dark channel,PM2.5 concentration estimation
AI 理解论文
溯源树
样例
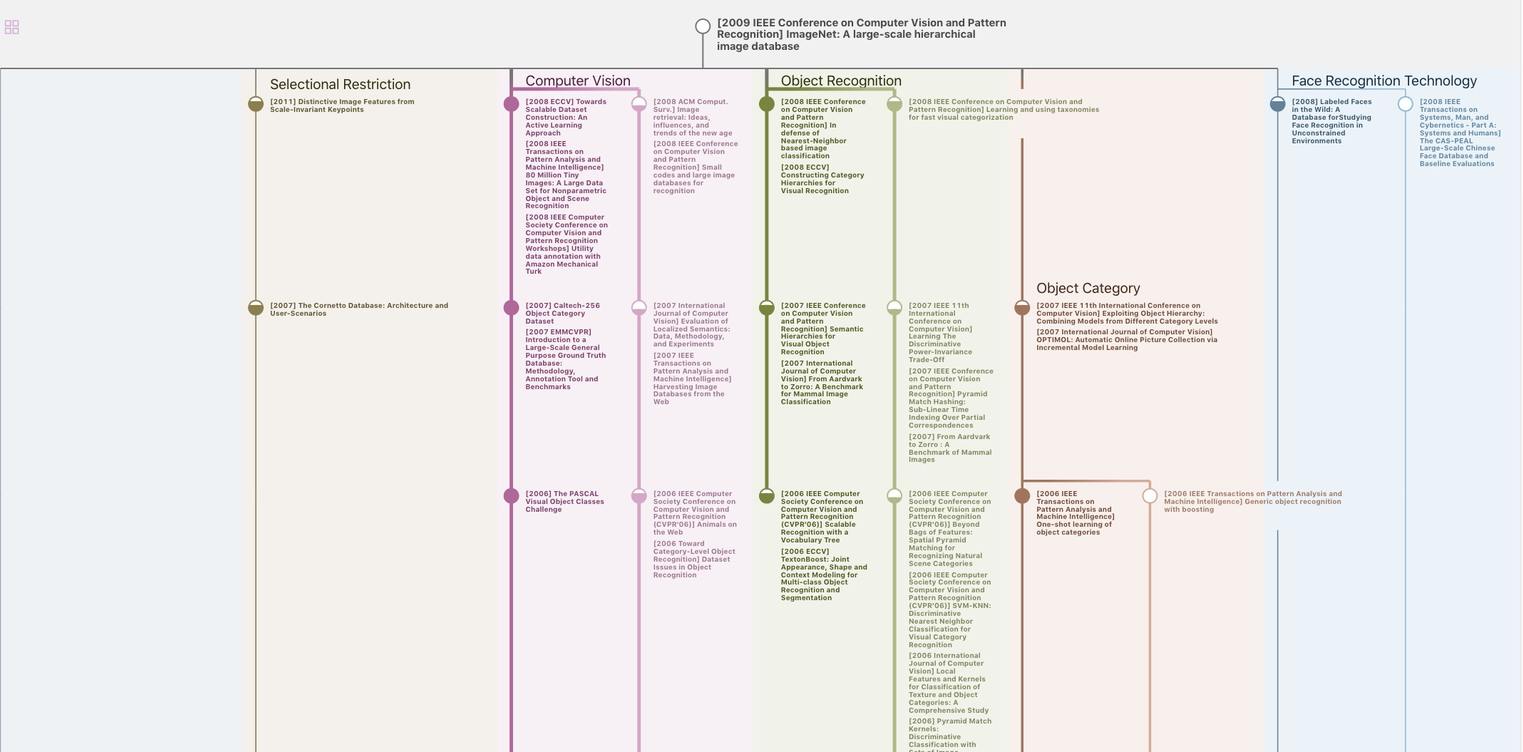
生成溯源树,研究论文发展脉络
Chat Paper
正在生成论文摘要