MO965: The Implementation of A Novel Algorithm for Optimal Predictor Selection, Exploiting Miqubo on D-Wave Quantum Annealer, for Predicting Kidney Graft Loss After Urinary Sepsis in Kidney Transplant Recipients
Nephrology Dialysis Transplantation(2022)
摘要
Abstract BACKGROUND AND AIMS Kidney transplant recipients (KTRs) hospitalized for urinary sepsis are at increased risk of graft loss (GL). Thus, there is a distinct need to develop models to accurately predict significant graft complications. MIQUBO (Mutual Information Quadratic Unconstrained Binary Optimization) is a concept, which in great approximation relies on optimization (maximization) of Mutual Information (the amount of information that is carried by a single variable) based on QUBO (Quadratic Unconstrained Binary Optimization) problem [1,2]. This method is found to be especially fit to solve complex statistical problems due to its ability to reduce models dimensionality, especially when deployed on a D-wave quantum computer [3]. In this study, we have investigated the feasibility of MIQUBO as a novel feature selection method to consciously extend machine learning prediction models, using a previously published dataset [4], to predict GL after urosepsis in KTRs. METHOD The analyzed dataset included 101 KTRs hospitalized for urosepsis, 100 KTRs hospitalized for UTI and 100 healthy KTRs without any history of UTI or sepsis. To predict GL at 12 months post-discharge after an episode of urosepsis, four features have been used to predict graft loss in a previous (unpublished) study by our group. These four features are further referred to as restricted variable set (RVS*). Subsequently, from the total of 150 variables included in the dataset, we have selected 12 additional features using MIQUBO deployed on a D-Wave quantum annealer. The extended variable set (EVS) was created based upon Conditional Mutual Information (CMI) of each variable. Then, 13 different machine learning (ML) models were build using each set of variables. Each developed model was explored for its performance according to the amount of included additional features (random versus MI-ranked). RESULTS The overall frequency of graft loss after 12 months of observation equaled 10.3% in the whole study cohort. However, the frequency of GL after urosepsis has reached 18% after 12 months from discharge, as compared with 3% in the healthy controls. The variables included in both sets of features (RVS and EVS) and their values were presented in Table 1. The best performance among developed models (using the EVS) has been achieved using Gaussian Process and QDA models, yielding area-under-the-receiver-operator-curve (AUROC) of approximately 0.91. From 13 developed pairs of models (for both sets of variables), 8 have proven to be statistically superior (AUROC comparison) in favor of MIQUBO-extended models. The mean difference of AUROCC between EVS- and RVS-models equaled 0.092 ± 0.11. Furthermore, a trend has been observed towards better performance of models (in regard of achieved AUC and recall) when ML-models were extended utilizing features based upon MIQUBO MI-ranking as compared with features randomly chosen from EVS. Representative plots were presented on Figure 1. The point ‘0’ on these plots refers to the parameters of models built on sole RVS. CONCLUSION Our preliminary experiment suggests that MIQUBO deployed on a quantum annealer is an efficient method for extending machine learning models with variables derived from extensive and imbalanced datasets, without losing on models’ reproducibility or accuracy.
更多查看译文
AI 理解论文
溯源树
样例
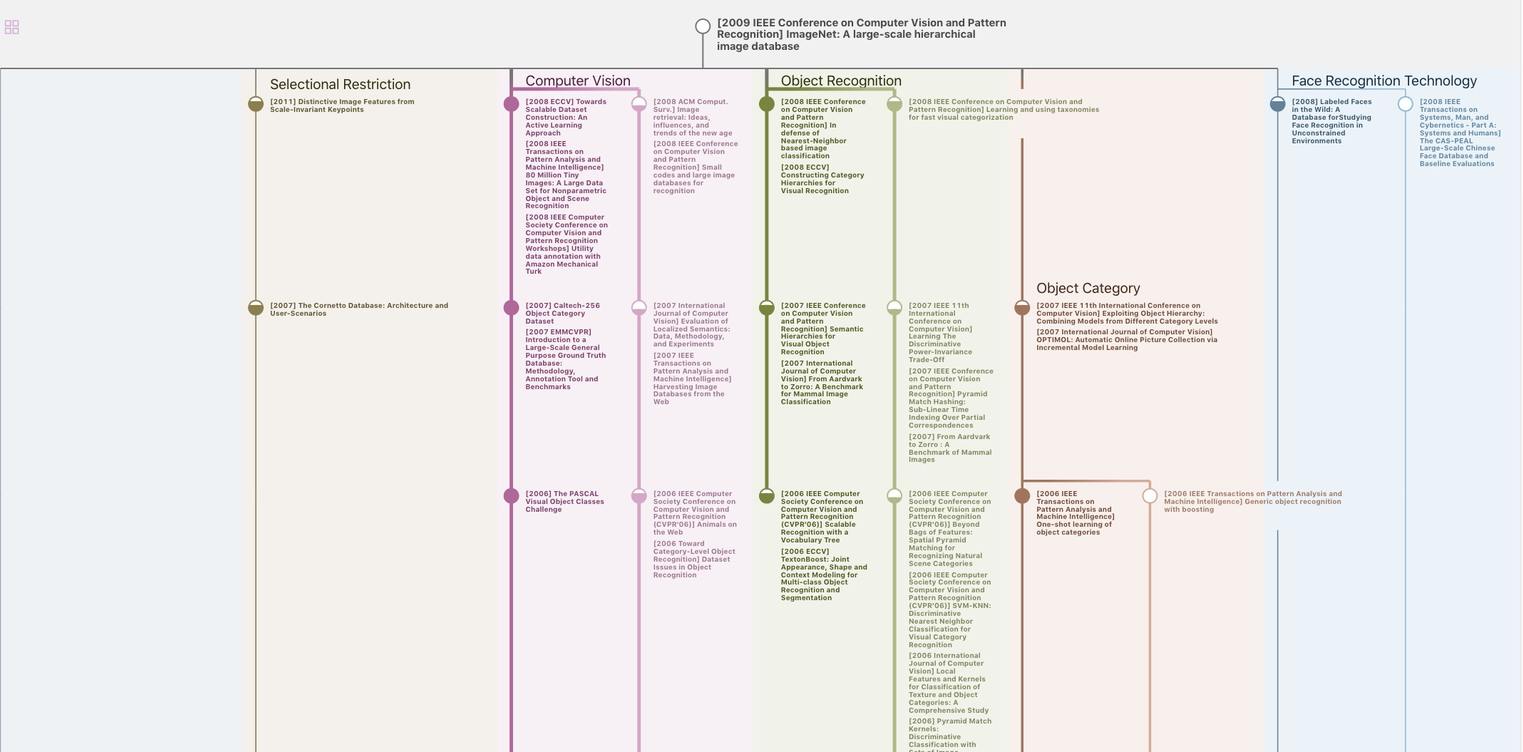
生成溯源树,研究论文发展脉络
Chat Paper
正在生成论文摘要