A Boundary Consistency-Aware Multitask Learning Framework for Joint Activity Segmentation and Recognition With Wearable Sensors
IEEE Transactions on Industrial Informatics(2023)
摘要
With the development of industrial and sensing technology, sensor-based activity recognition has become a promising technology for informatics applications. However, in a typical activity recognition procedure, sensory data segmentation, usually considered a preprocess with sliding windows, rarely has been investigated and significantly affected the recognition performance. In this article, we propose a novel deep-learning method to jointly segment and recognize activities with wearable sensors. Our contributions are three-fold: First, we introduce a multistage temporal convolutional network for sample-level activity prediction to overcome the multiclass windows problem. Second, for alleviating oversegmentation errors, our model forms a multitask learning framework with a boundary prediction module to adjust the entire model’s gradients. Third, we innovatively propose a boundary consistency loss to enforce the consistency of the activity and boundary prediction. Our method shows impressive performance on three public datasets, especially achieving 16% improvement over very recently advanced competing methods with class-average F1-score on the Hospital dataset. The code of this work will be open source on
https://github.com/xspc/Segmentation-Sensor-based-HAR
.
更多查看译文
关键词
Activity recognition,boundary prediction,multitask learning,time-series segmentation,wearable sensors
AI 理解论文
溯源树
样例
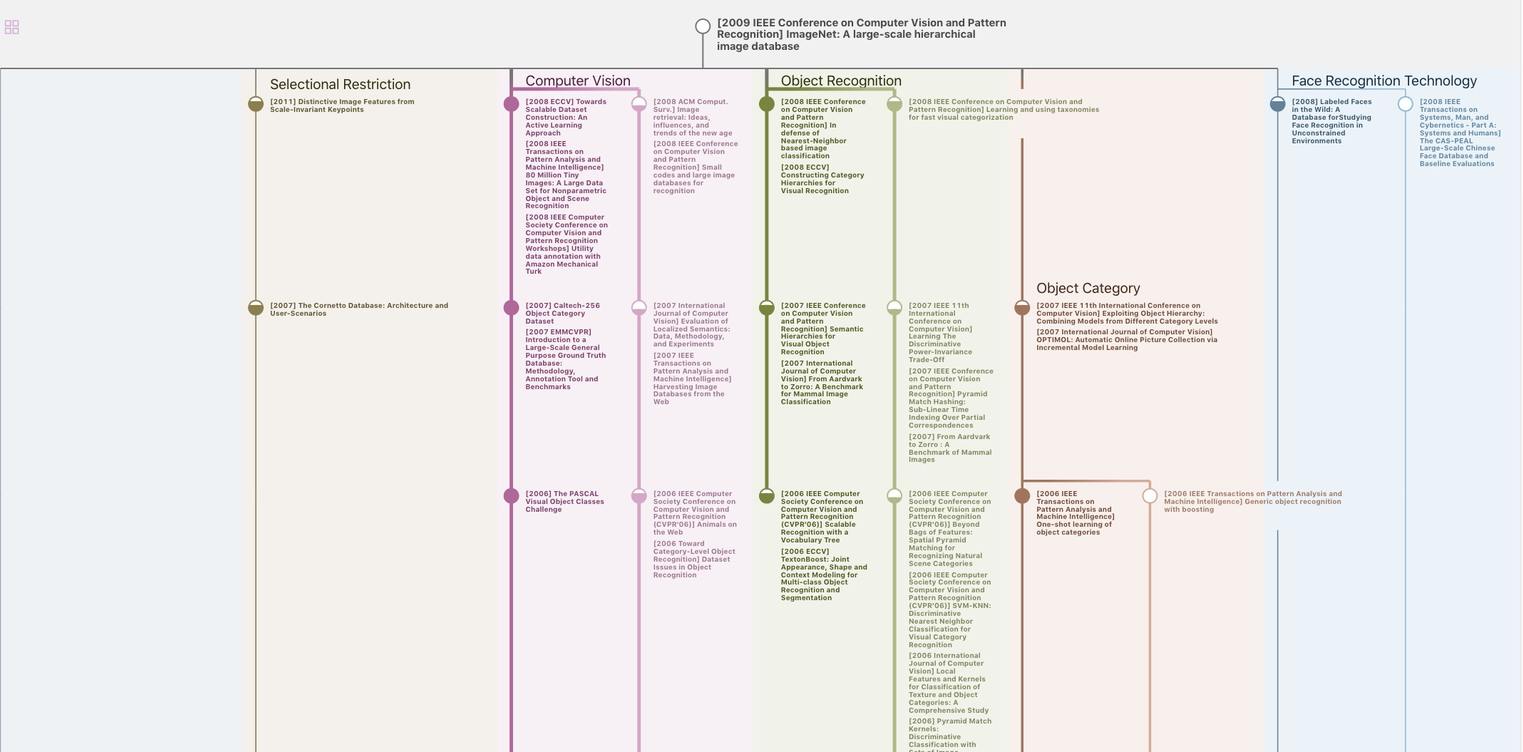
生成溯源树,研究论文发展脉络
Chat Paper
正在生成论文摘要