Impact of finite size effect on applicability of generalized fractal and spectral dimensions to biological networks
SSRN Electronic Journal(2022)
摘要
In recent years, researchers have explored fractal dimension, spectral dimension, and multifractal analysis as ways of describing the emergent hierarchical structure of complex networks. However, fractality implies an infinite recursion that is impossible for finite networks describing real-world biological systems. We show that there is a substantial finite size effect on two widely used empirical methods (box-covering and sandbox) of estimating generalized fractal dimensions. As a partial solution to this issue we introduce here a generalized method for calculating network spectral dimension using a memory-biased random walk (MBRW). To observe the impact of network size, we start with an ensemble of networks representing a variety of biological systems, identify their community structures using Infomap, and use a modified stochastic block model to generate networks with similar community structure but varying size. We find that, compared to shortest-path-based generalized fractal dimension methods, the MBRW generalized spectral dimension (Dq) shows a clearer and more consistent ordering of networks by community structure for all orders (q) considered. We also find that, among the measures of multifractality and multispectrality, only MBRW multi-spectrality (range of Dq values) changes in a consistent direction under randomization of each level of community structure. Our results show that network size is an important consideration when comparing the fractal or spectral dimensions of real-world networks and that observing the interaction between network structure and an agent acting in time with memory provides insights into network structure not available through calculations based on purely topological features.
更多查看译文
关键词
Biological networks,Fractal dimension,Spectral dimension,Multifractal analysis,Multi-spectral analysis,Memory-biased random walk
AI 理解论文
溯源树
样例
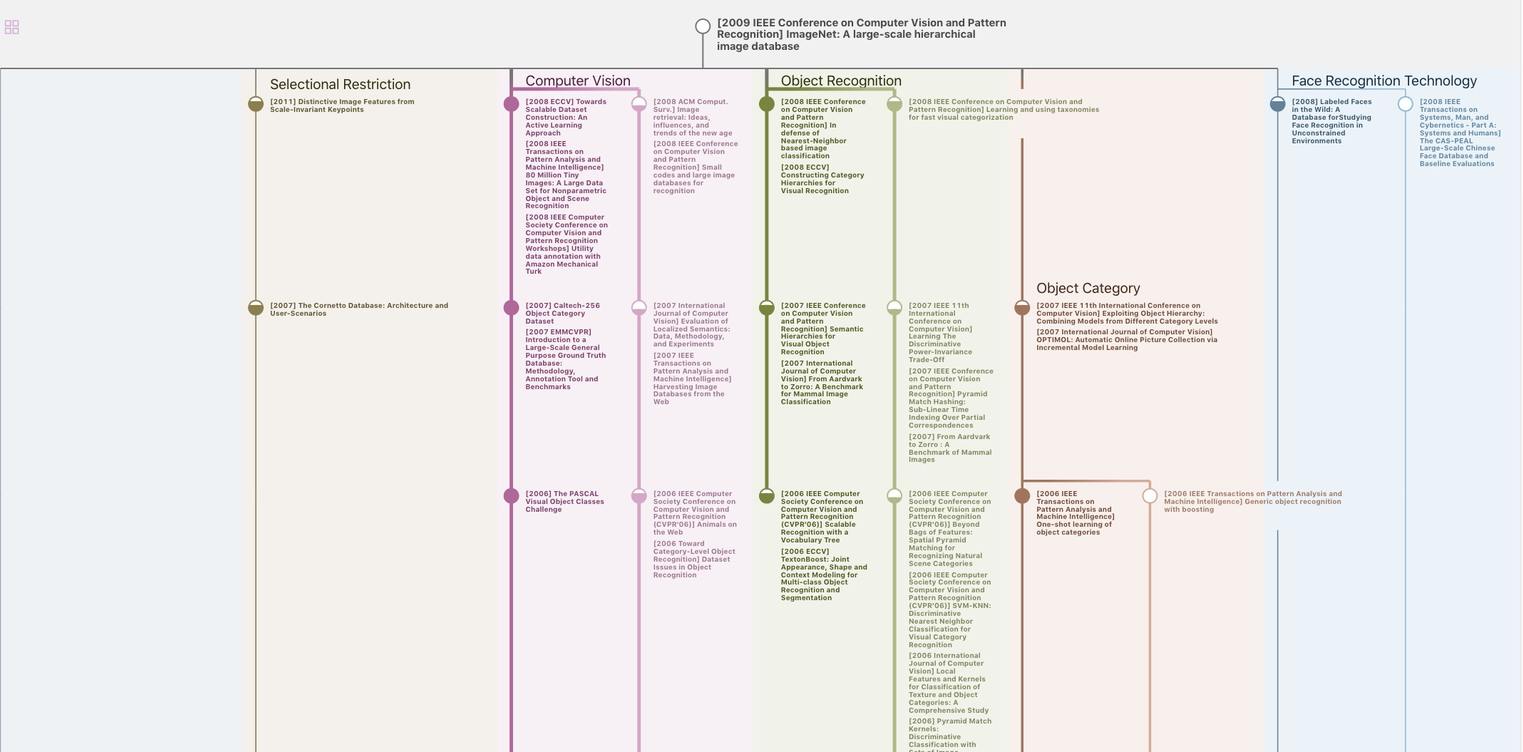
生成溯源树,研究论文发展脉络
Chat Paper
正在生成论文摘要