A Bayesian Approach for Joint Discriminative Dictionary and Classifier Learning
IEEE Transactions on Systems, Man, and Cybernetics: Systems(2022)
摘要
Sparse representation has been widely applied to image classification, where the key issue is to extract a suitable discriminative dictionary. To this end, we propose a joint dictionary and classifier learning algorithm based on a parameterized Bayesian model. Therein, the Gaussian priors of a dictionary endow it with the capability of discrimination and representation. Moreover, we introduce a multivariate Gaussian prior for the sparse codes to achieve group sparsity, thereby substantially improving the classification performance. Furthermore, the sparse codes are estimated by a group-sparse Bayesian learning (GSBL) method, and the dictionary atoms are updated sequentially by maximizing a posterior. Moreover, to avoid manual parameter adjustment, the hyperparameters are optimized by an evidence maximization method. Accordingly, we develop a classification scheme via GSBL. Finally, extensive experiments are conducted on six benchmark datasets of face classification, object recognition, handwritten recognition, and scene categorization to substantiate the effectiveness and superiority of the proposed method.
更多查看译文
关键词
Dictionary learning,image classification,sparse Bayesian learning,sparse representation (SR)
AI 理解论文
溯源树
样例
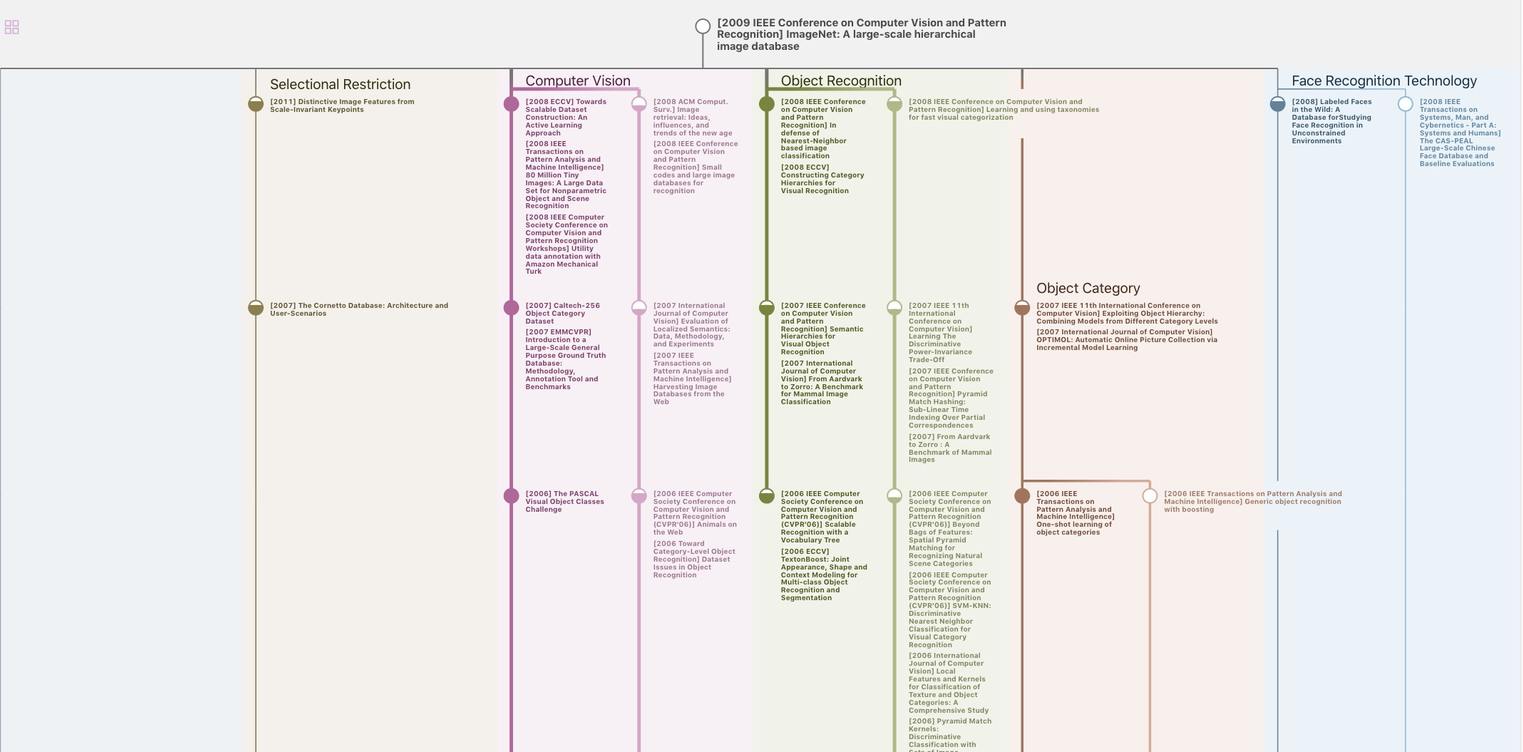
生成溯源树,研究论文发展脉络
Chat Paper
正在生成论文摘要